Evaluating Gender Bias in Natural Language Inference
arxiv(2021)
摘要
Gender-bias stereotypes have recently raised significant ethical concerns in natural language processing. However, progress in detection and evaluation of gender bias in natural language understanding through inference is limited and requires further investigation. In this work, we propose an evaluation methodology to measure these biases by constructing a challenge task that involves pairing gender-neutral premises against a gender-specific hypothesis. We use our challenge task to investigate state-of-the-art NLI models on the presence of gender stereotypes using occupations. Our findings suggest that three models (BERT, RoBERTa, BART) trained on MNLI and SNLI datasets are significantly prone to gender-induced prediction errors. We also find that debiasing techniques such as augmenting the training dataset to ensure a gender-balanced dataset can help reduce such bias in certain cases.
更多查看译文
关键词
gender bias,natural language inference
AI 理解论文
溯源树
样例
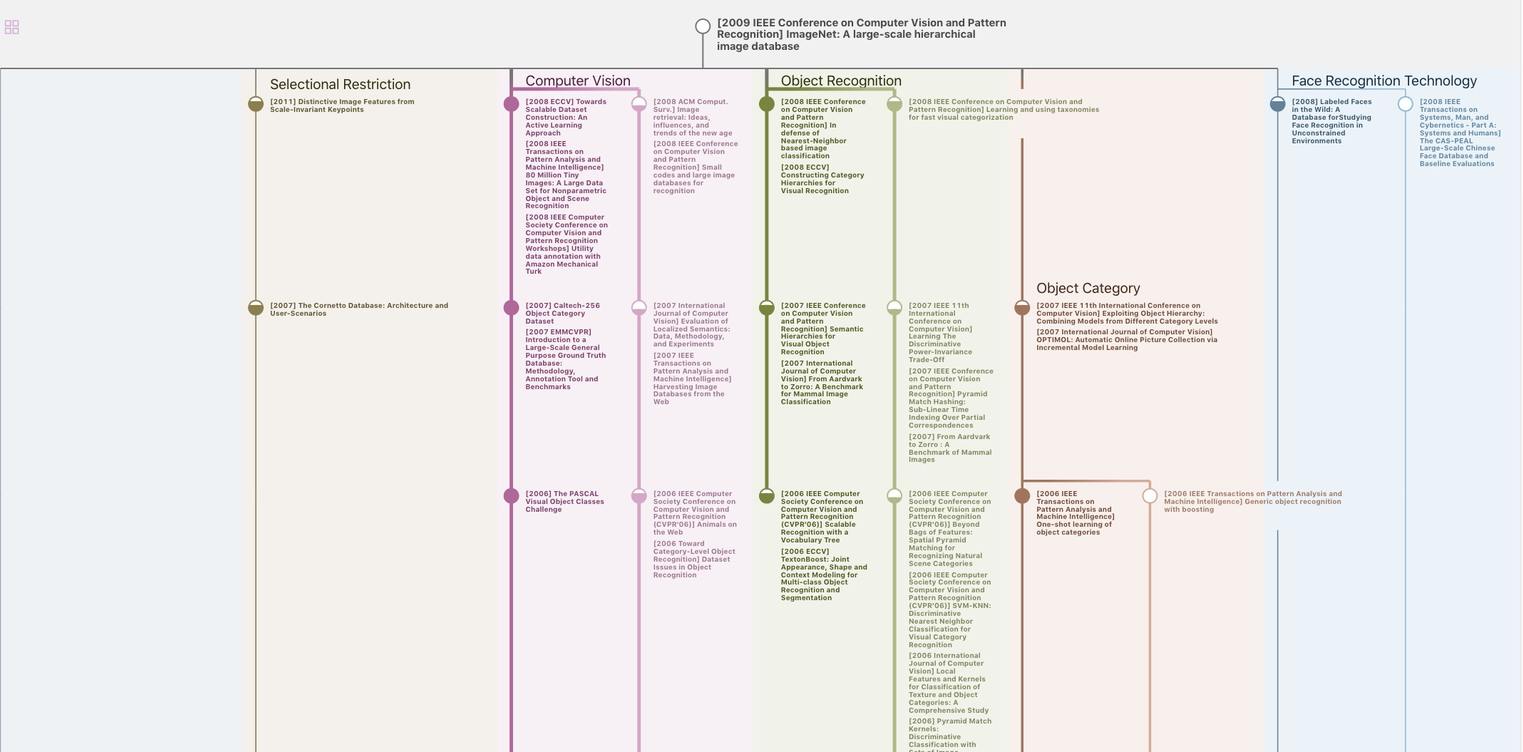
生成溯源树,研究论文发展脉络
Chat Paper
正在生成论文摘要