Accelerated forward-backward optimization using deep learning
SIAM JOURNAL ON OPTIMIZATION(2024)
摘要
We propose several deep -learning accelerated optimization solvers with convergence guarantees. We use ideas from the analysis of accelerated forward -backward schemes like FISTA, but instead of the classical approach of proving convergence for a choice of parameters, such as a step -size, we show convergence whenever the update is chosen in a specific set. Rather than picking a point in this set using some predefined method, we train a deep neural network to pick the best update within a given space. Finally, we show that the method is applicable to several cases of smooth and nonsmooth optimization and show superior results to established accelerated solvers.
更多查看译文
关键词
convex optimization,deep learning,proximal-gradient algorithm,inverse problems
AI 理解论文
溯源树
样例
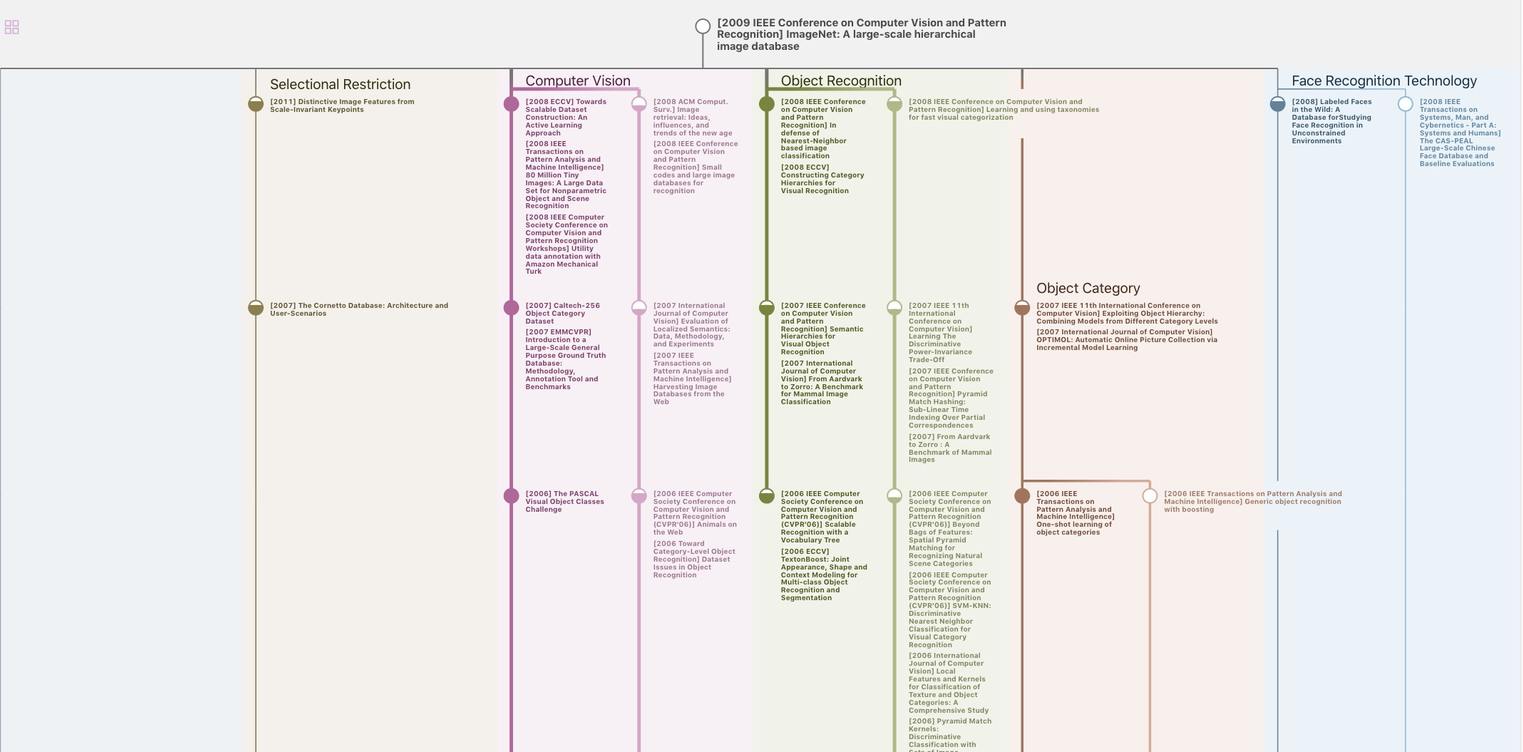
生成溯源树,研究论文发展脉络
Chat Paper
正在生成论文摘要