Not All Relevance Scores are Equal: Efficient Uncertainty and Calibration Modeling for Deep Retrieval Models
Research and Development in Information Retrieval(2021)
摘要
ABSTRACTIn any ranking system, the retrieval model outputs a single score for a document based on its belief on how relevant it is to a given search query. While retrieval models have continued to improve with the introduction of increasingly complex architectures, few works have investigated a retrieval model's belief in the score beyond the scope of a single value. We argue that capturing the model's uncertainty with respect to its own scoring of a document is a critical aspect of retrieval that allows for greater use of current models across new document distributions, collections, or even improving effectiveness for down-stream tasks. In this paper, we address this problem via an efficient Bayesian framework for retrieval models which captures the model's belief in the relevance score through a stochastic process while adding only negligible computational overhead. We evaluate this belief via a ranking based calibration metric showing that our approximate Bayesian framework significantly improves a retrieval model's ranking effectiveness through a risk aware reranking as well as its confidence calibration. Lastly, we demonstrate that this additional uncertainty information is actionable and reliable on down-stream tasks represented via cutoff prediction.
更多查看译文
关键词
uncertainty, neural networks, calibration, search
AI 理解论文
溯源树
样例
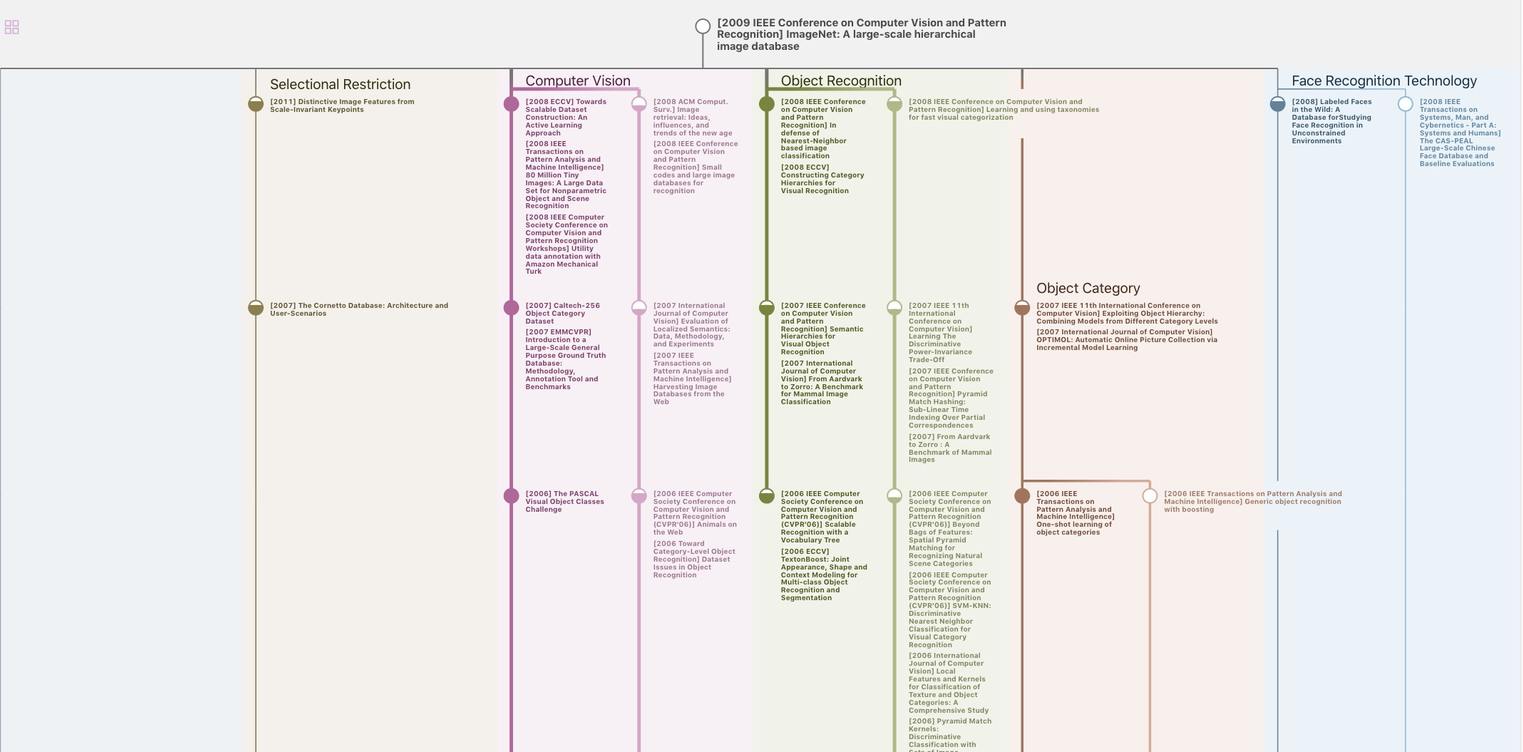
生成溯源树,研究论文发展脉络
Chat Paper
正在生成论文摘要