Stacked neural networks for predicting the membranes performance by treating the pharmaceutical active compounds
NEURAL COMPUTING & APPLICATIONS(2021)
Abstract
The removal of pharmaceutical actives compounds (PhACs) by nanofiltration (NF) and reverse osmosis (RO) of paramount importance in membrane separation processes. However, modeling remains a difficult approach due to the strongly nonlinear performance of the removal mechanisms of organic molecules by NF/RO. The present work features the application of neural networks based on quantitative structure–activity relationship (single neural networks “QSAR-SNN” and bootstrap aggregated neural networks “QSAR-BANN (Staking of 30 networks) ") for prediction of the removal of 23 pharmaceutical active compounds (PhACs). Overall, the models proposed are able to accurately correlate 599 experimental data points gathered from the literature. According to the results, the QSAR-BANN (Staking of 30 networks) is a more powerful and effective computational learning machine than the QSAR-SNN. The regression coefficients “ R^2 ” and the root mean squared error “ RMSE ” for the QSAR-BANN (Staking of 30 networks) model are estimated to be 0.9672 and 3.2810
MoreTranslated text
Key words
Stacked Neural Networks,QSAR,Pharmaceutical Active Compounds,Removal,Membranes
AI Read Science
Must-Reading Tree
Example
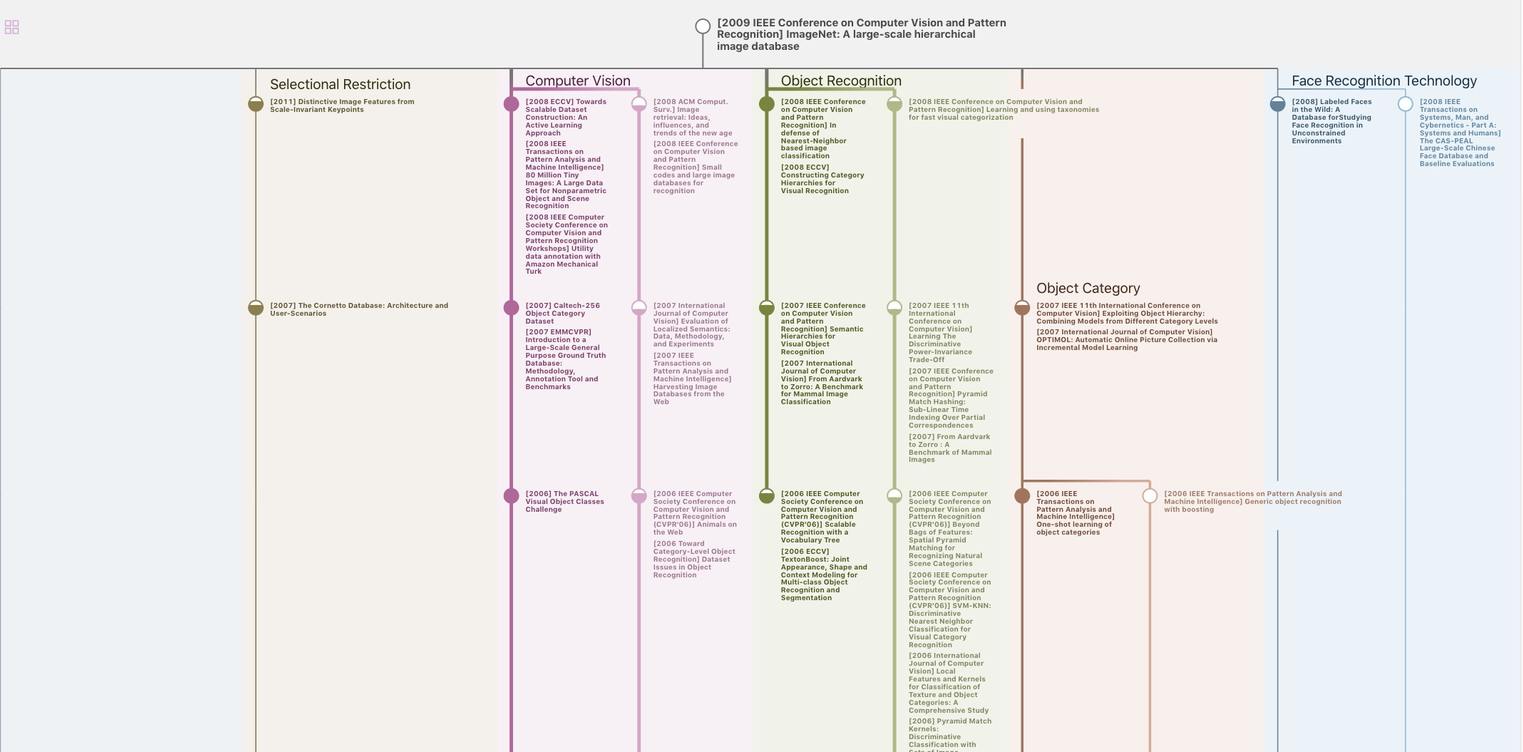
Generate MRT to find the research sequence of this paper
Chat Paper
Summary is being generated by the instructions you defined