Maize Crop Coefficient Estimation Based On Spectral Vegetation Indices And Vegetation Cover Fraction Derived From Uav-Based Multispectral Images
AGRONOMY-BASEL(2021)
摘要
Remote sensing-based crop monitoring has evolved unprecedentedly to supply multispectral imagery with high spatial-temporal resolution for the assessment of crop evapotranspiration (ETc). Several methodologies have shown a high correlation between the Vegetation Indices (VIs) and the crop coefficient (Kc). This work analyzes the estimation of the crop coefficient (Kc) as a spectral function of the product of two variables: VIs and green vegetation cover fraction (f(v)). Multispectral images from experimental maize plots were classified to separate pixels into three classes (vegetation, shade and soil) using the OBIA (Object Based Image Analysis) approach. Only vegetation pixels were used to estimate the VIs and f(v) variables. The spectral Kc(fv:VI) models were compared with Kc based on Cumulative Growing Degree Days (CGDD) (Kc-c(GDD)). The maximum average values of Normalized Difference Vegetation Index (NDVI), WDRVI, amd EVI2 indices during the growing season were 0.77, 0.21, and 1.63, respectively. The results showed that the spectral Kc(fv:VI) model showed a strong linear correlation with Kc-c(GDD) (R-2 > 0.80). The model precision increases with plant densities, and the Kc(fv:NDVI) with 80,000 plants/ha had the best fitting performance (R-2 = 0.94 and RMSE = 0.055). The results indicate that the use of spectral models to estimate Kc based on high spatial and temporal resolution UAV-images, using only green pixels to compute VI and f(v) crop variables, offers a powerful and simple tool for ETc assessment to support irrigation scheduling in agricultural areas.
更多查看译文
关键词
Growing Degree Days, crop water requirements, irrigation management, remote sensing, OBIA
AI 理解论文
溯源树
样例
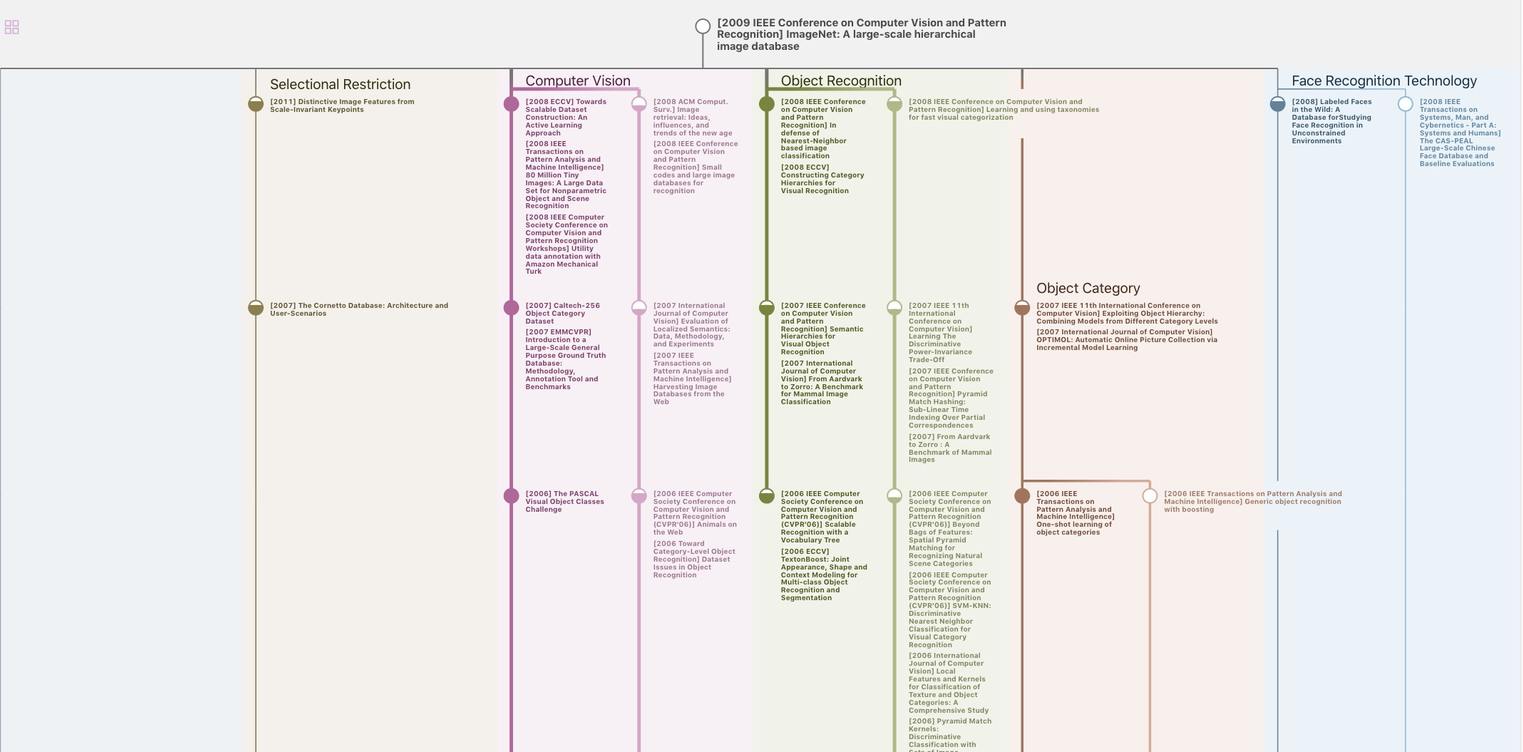
生成溯源树,研究论文发展脉络
Chat Paper
正在生成论文摘要