End-to-end joint spectral-spatial compression and reconstruction of hyperspectral images using a 3D convolutional autoencoder
JOURNAL OF ELECTRONIC IMAGING(2021)
Abstract
Hyperspectral images (HSIs) must be preprocessed by a compression model, which reduces the pressure of storing and transmitting huge data in applications. Whereas most of the existing methods consider only the compression or reconstruction requirements, an end-to-end optimization would simultaneously improve the performance of both requirements. We propose a three-dimensional convolutional autoencoder that precisely achieves end-to-end joint spectral- spatial compression and reconstruction of HSIs. In an experimental evaluation, the proposed method improved the spectral angle mapper, peak signal-to-noise ratio, and structural similarity index measurement of the reconstructed HSIs by 20.8% to 33.1%, 0.9% to 11.5%, and 0.5% to 2.2%, respectively, relative to competitive methods. (c) 2021 SPIE and IS&T [DOI: 10.1117/1.JEI.30 .4.041403]
MoreTranslated text
Key words
hyperspectral image,three-dimensional convolutional autoencoder,spectral-spatial compression,reconstruction,end-to-end optimization
AI Read Science
Must-Reading Tree
Example
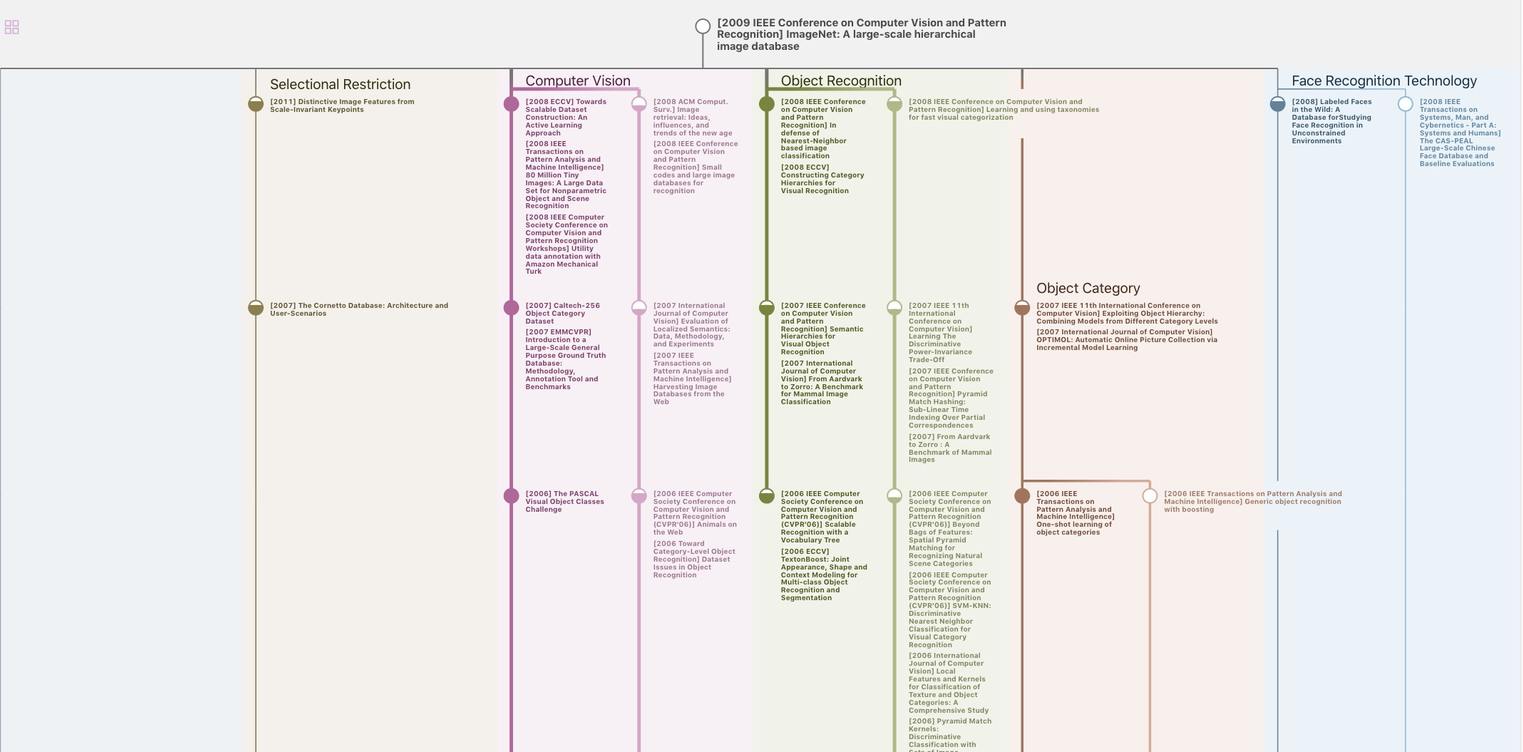
Generate MRT to find the research sequence of this paper
Chat Paper
Summary is being generated by the instructions you defined