Fast Post-Hoc Normalization for Brain Inspired Sparse Coding on a Neuromorphic Device
IEEE Transactions on Parallel and Distributed Systems(2022)
摘要
Exploration of novel computational platforms is critical for the advancement of artificial intelligence as we approach the physical limitations of traditional hardware. Biologically accurate, energy efficient neuromorphic systems are particularly promising for enabling future breakthroughs because of their ability to process information in parallel and to scale using extremely low power. Sparse coding is a signal processing technique which has been known to model the information encoding in the primary visual cortex. When sparse solutions are solved using local neuron competition along with the unsupervised dictionary learning that mimics cortical development, we can build an end to end, hardware to software, brain inspired solution to a machine learning problem. In this article, we perform a detailed comparison of sparse coding solutions generated classically by orthogonal matching pursuit (OMP) implemented on a conventional digital processor with spike-based solutions obtained using the Intel Loihi neuromorphic processor. A novel “post-hoc” normalization technique to shorten simulation time for Loihi is presented along with analysis of optimal parameter selection, reconstruction errors, and unsupervised dictionary learning for Loihi approaches and their classical counterparts. Preliminary results show that both the Loihi full simulation approach and the post-hoc normalization approach are well suited to neuromorphic processors and operate in a size, weight and power regime that is not accessible by classical approaches. Ultimately, the use of this normalization technique allows for faster and, often, better solutions than demonstrated previously.
更多查看译文
关键词
Neuromorphic computing,machine learning,artificial intelligence,neurocomputers,computer vision,signal processing
AI 理解论文
溯源树
样例
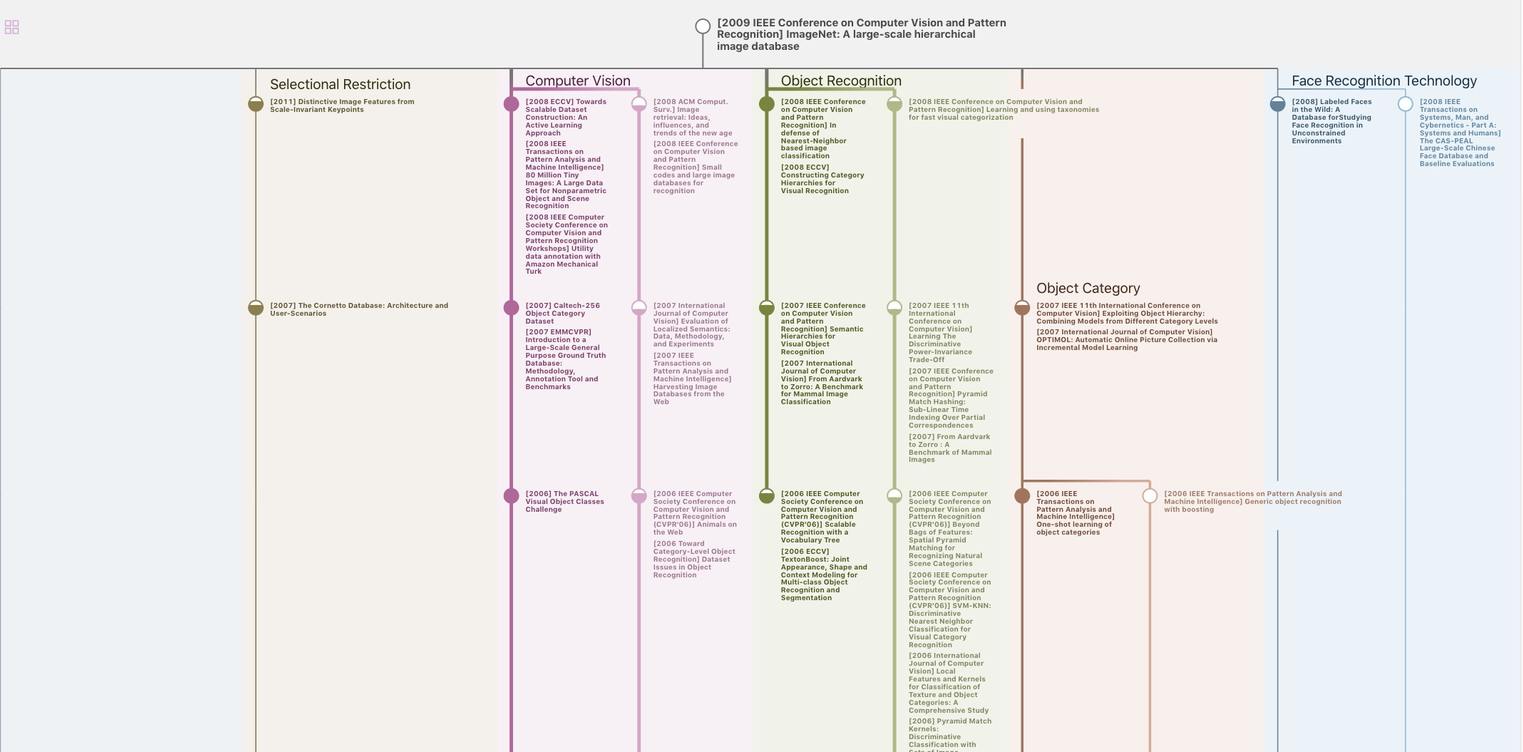
生成溯源树,研究论文发展脉络
Chat Paper
正在生成论文摘要