Machine Learning-Based Security-Aware Spatial Modulation For Heterogeneous Radio-Optical Networks
PROCEEDINGS OF THE ROYAL SOCIETY A-MATHEMATICAL PHYSICAL AND ENGINEERING SCIENCES(2021)
摘要
In this article, we propose a physical layer security (PLS) technique, namely security-aware spatial modulation (SA-SM), in a multiple-input multiple-output-based heterogeneous network, wherein both optical wireless communications and radio-frequency (RF) technologies coexist. In SA-SM, the time-domain signal is altered prior to transmission using a key at the physical layer for combating eavesdropping. Unlike conventional PLS techniques, SA-SM does not rely on channel characteristics for securing the information, as its perception is self-imposed, which allows its adoption in radio-optical networks. Additionally, a novel periodical key selection algorithm is proposed. Instead of having multiple keys stored in the nodes, by using off-the-shelf and low-complexity machine learning (ML) methods, including a support vector machine, logistic regression and a single-layer neural network, SA-SM nodes can estimate the used key. Results show that a positive secrecy capacity can be achieved for both the RF and optical links by using 1000 different keys, with a minimal signal-to-noise ratio penalty of less than 5dB for the legitimate user using SA-SM versus conventional transmission at a bit-error-rate of 10(-4). The analysis also includes computational time and classification accuracy evaluation of the various proposed ML techniques using different hardware architectures.
更多查看译文
关键词
physical layer security, machine learning, heterogeneous networks, optical wireless communications, wireless communications
AI 理解论文
溯源树
样例
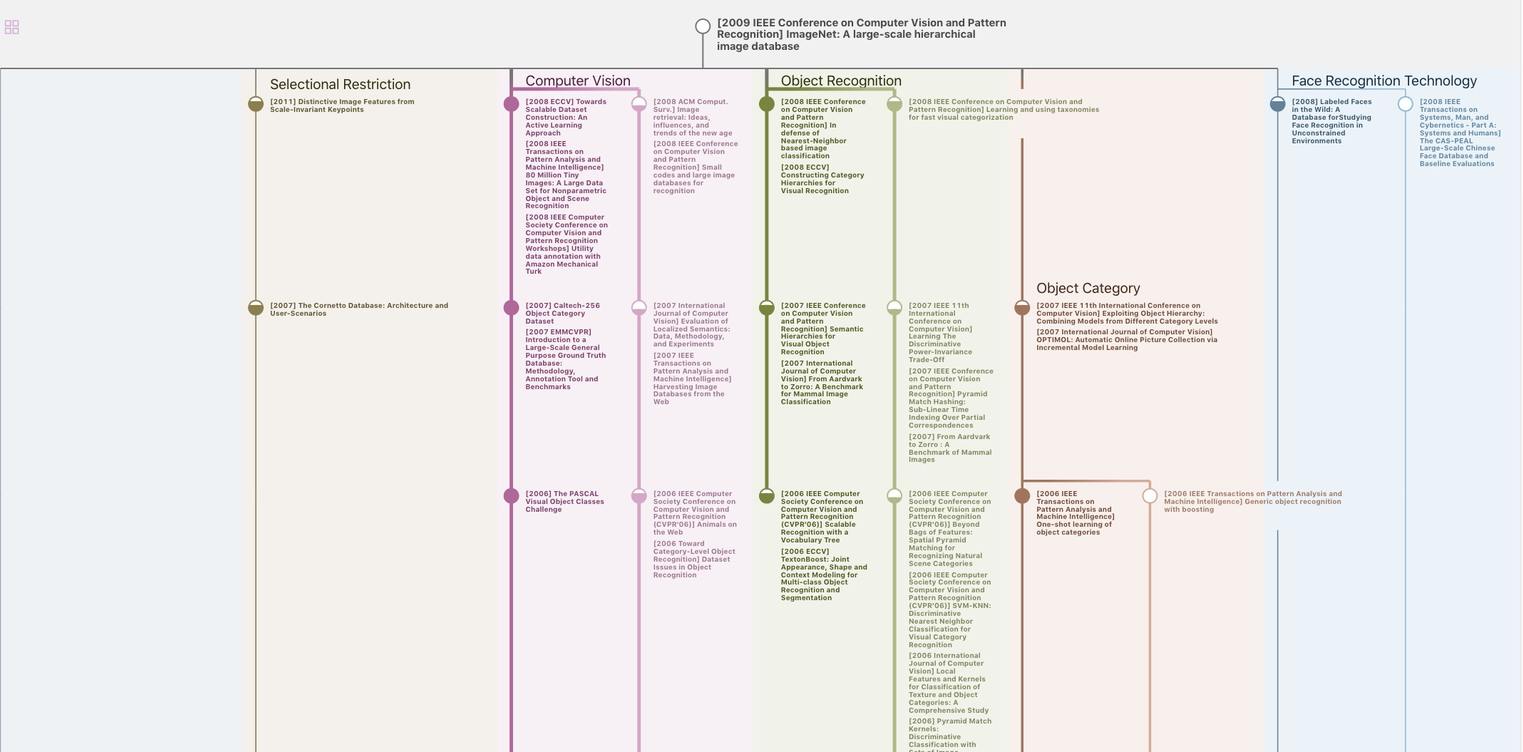
生成溯源树,研究论文发展脉络
Chat Paper
正在生成论文摘要