Motion Blur Removal With Quality Assessment Guidance
IEEE TRANSACTIONS ON MULTIMEDIA(2021)
摘要
Non-uniform blind motion deblurring is a challenging yet fundamental task in the computer vision field, which aims to restore the latent sharp image from the blurry input. Recently, deep-learning-based methods have made significant improvement and progress, on the metric of PSNR. They achieve good results mainly because they adopt Mean Squared Error (MSE) as the optimization objective, in addition to their good model design. However, simple adoption of the PSNR metric and the MSE loss, has non-ignorable disadvantages. PSNR cannot always succeed in assessing the deblurred quality in accordance with the human visual system (HVS), and MSE guides the network to generate over-smoothed images. To address these problems, we are the first to propose the deep-learning-based multi-scale non-reference quality assessment network (Deep DEBLUR-IQA) for assessing the quality of deblurred results. Moreover, a deblurring network of high efficiency is presented. It is more than 50 times faster than other SOTA multi-scale Convolution Neural Network (CNN) methods, with the newly propose Residual Dilated Block (RDB) and Light ResBlock (LRB). The deblurring network's performance can be further boosted with Multiple Dilation Block (MDB), with an acceptable speed decrease. Finally, and most importantly, we are the first to let Deep DEBLUR-IQA guide the deblurring network's optimization. This IQA-guided enhancement paradigm can significantly improve the deblurring results' subjective quality while achieving excellent PSNR. Experimental results demonstrate that the proposed method performs favorably against state-of-the-art methods quantitatively and qualitatively.
更多查看译文
关键词
Task analysis, Image restoration, Measurement, Kernel, Optimization, Quality assessment, Convolution, Deep learning, image quality assessment (IQA), low-level vision, single image deblurring, task-driven
AI 理解论文
溯源树
样例
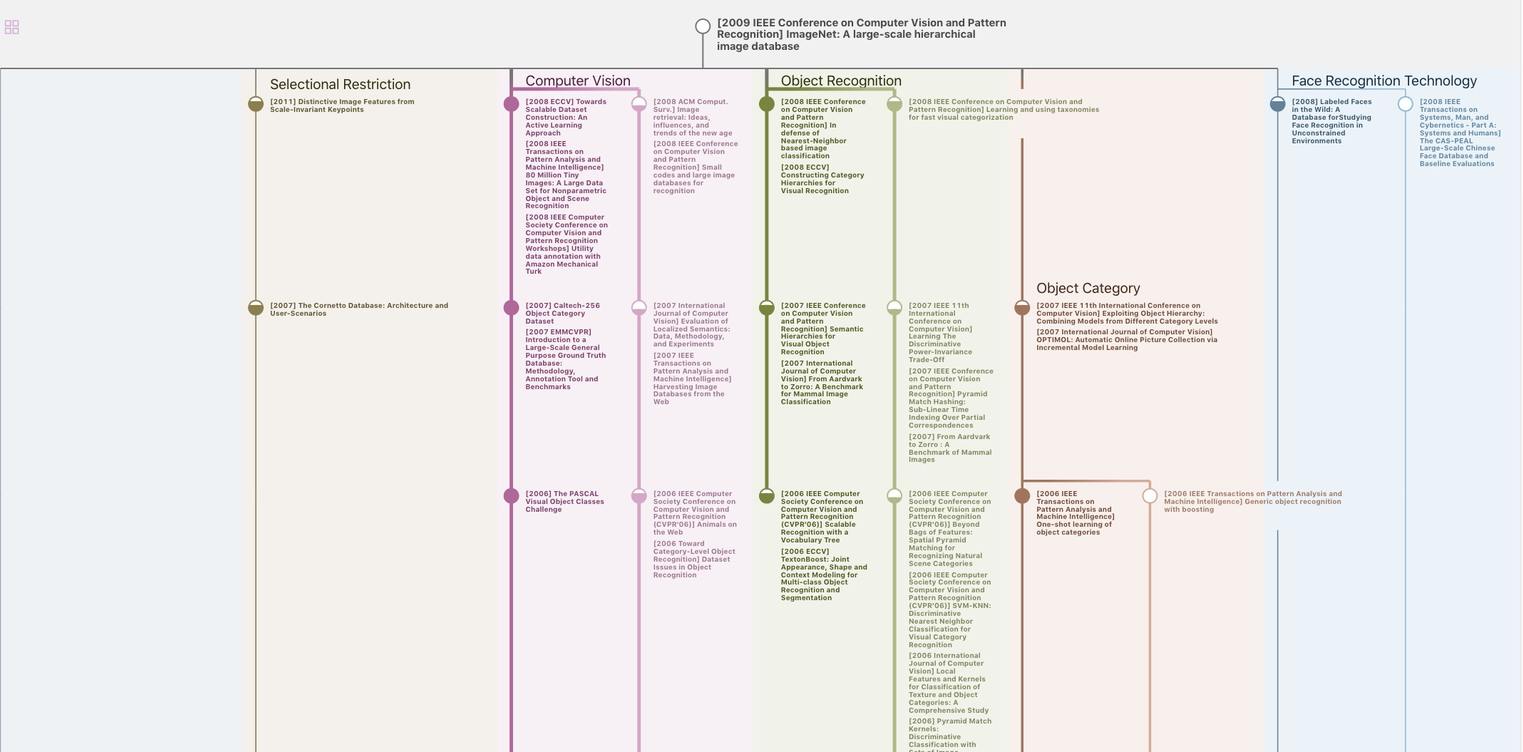
生成溯源树,研究论文发展脉络
Chat Paper
正在生成论文摘要