An innovative and automated method for characterizing wood defects on trunk surfaces using high-density 3D terrestrial LiDAR data
ANNALS OF FOREST SCIENCE(2021)
摘要
• Key message We designed a novel method allowing to automatically detect and measure defects on the surface of trunks including branches, branch scars, and epicormics from terrestrial LiDAR data by using only high-density 3D information. We could automatically detect and measure the defects with a diameter as small as 0.5 cm on either oak (Quercus petraea (Matt.) Liebl.) or beech (Fagus sylvatica L.) trees that display either rough or smooth bark. • Context Ground-based light detection and ranging (LiDAR) technology describes standing trees with a high level of detail. This provides an opportunity to assess standing tree quality and to use this information in forest inventory. Assuming the availability of a very high level of detail, we could extract information about the surface defects, mainly inherited from past ramification and having a strong impact on wood quality. • Aims Within the general framework of the development of a computing method able to detect, identify, and quantify the defects on the trunk surface described from 3D data produced by a terrestrial LiDAR, this study focuses on the relevance of the whole process for two tree species with contrasted bark roughness ( Quercus petraea (Matt.) Liebl. and Fagus sylvatica L.) in terms of detection, identification of the defects, and comparison with measurements performed manually on the bark surface. • Methods First, a segmentation algorithm detected singularities on the trunk surface. Next, a Random Forests machine learning algorithm identified the most probable defect type and allowed the elimination of false detections. Finally, we estimated the position, horizontal, and vertical dimensions of each defect from 3D data, and we compared them to those observed directly on the trunk by an operator. • Results The defects were detected and classified with a high accuracy with an average F_1 score (harmonic mean of precision and recall) of 0.74. There were differences in computed and observed defect areas, but a much closer agreement for the number of defects. • Conclusion The information about the defects present on the trunk surface measured from terrestrial LiDAR data can be used in an automated procedure for grading standing trees or roundwoods.
更多查看译文
关键词
Tree quality,Roundwood quality,Forest inventory,Wood grading,Machine learning,Bark
AI 理解论文
溯源树
样例
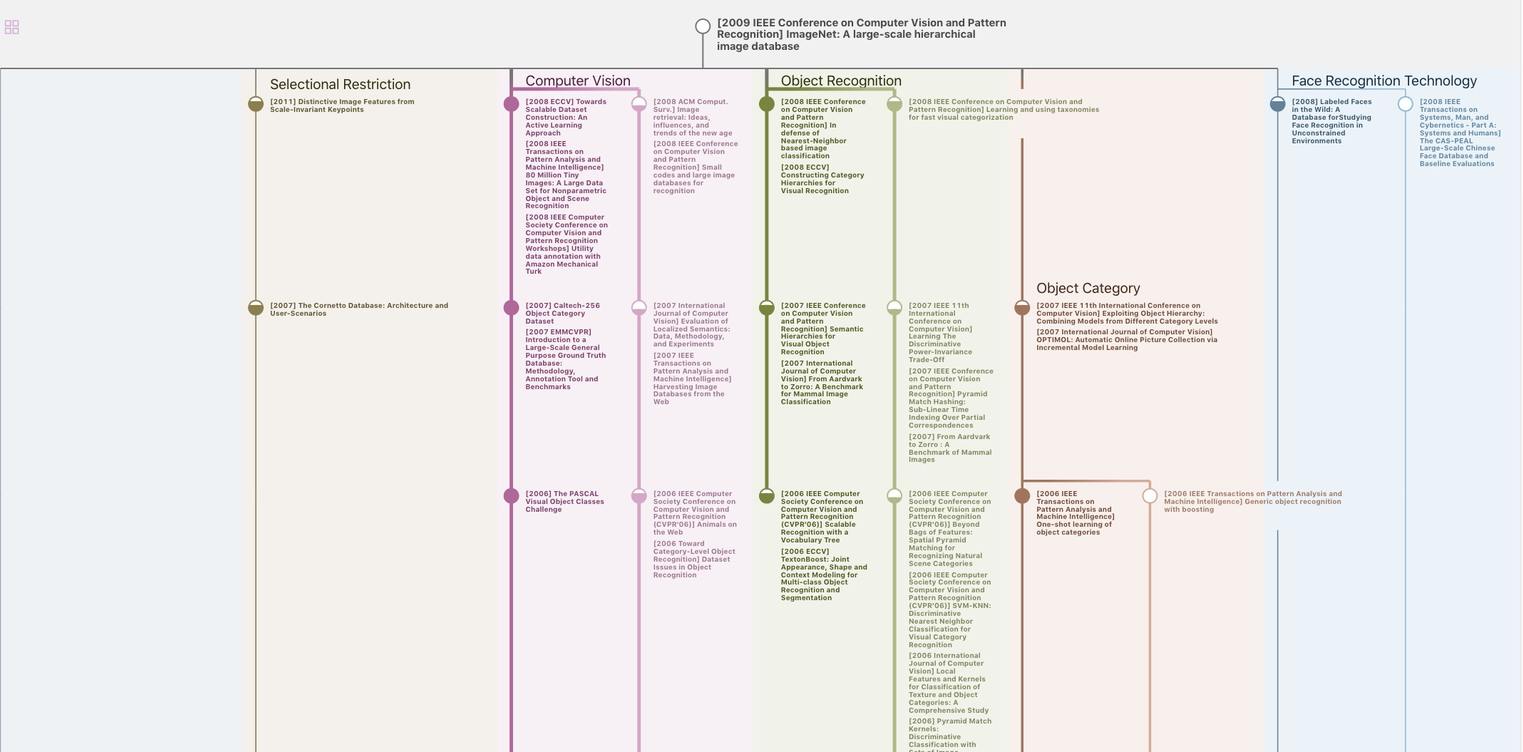
生成溯源树,研究论文发展脉络
Chat Paper
正在生成论文摘要