Assessment Of Machine Learning Algorithms For Modeling The Spatial Distribution Of Bark Beetle Infestation
FORESTS(2021)
摘要
Machine learning algorithms (MLAs) are used to solve complex non-linear and high-dimensional problems. The objective of this study was to identify the MLA that generates an accurate spatial distribution model of bark beetle (Ips typographus L.) infestation spots. We first evaluated the performance of 2 linear (logistic regression, linear discriminant analysis), 4 non-linear (quadratic discriminant analysis, k-nearest neighbors classifier, Gaussian naive Bayes, support vector classification), and 4 decision trees-based MLAs (decision tree classifier, random forest classifier, extra trees classifier, gradient boosting classifier) for the study area (the Horni Plana region, Czech Republic) for the period 2003-2012. Each MLA was trained and tested on all subsets of the 8 explanatory variables (distance to forest damage spots from previous year, distance to spruce forest edge, potential global solar radiation, normalized difference vegetation index, spruce forest age, percentage of spruce, volume of spruce wood per hectare, stocking). The mean phi coefficient of the model generated by extra trees classifier (ETC) MLA with five explanatory variables for the period was significantly greater than that of most forest damage models generated by the other MLAs. The mean true positive rate of the best ETC-based model was 80.4%, and the mean true negative rate was 80.0%. The spatio-temporal simulations of bark beetle-infested forests based on MLAs and GIS tools will facilitate the development and testing of novel forest management strategies for preventing forest damage in general and bark beetle outbreaks in particular.
更多查看译文
关键词
machine learning, classifier, spatial distribution model, geographical information system, forest damage, bark beetle
AI 理解论文
溯源树
样例
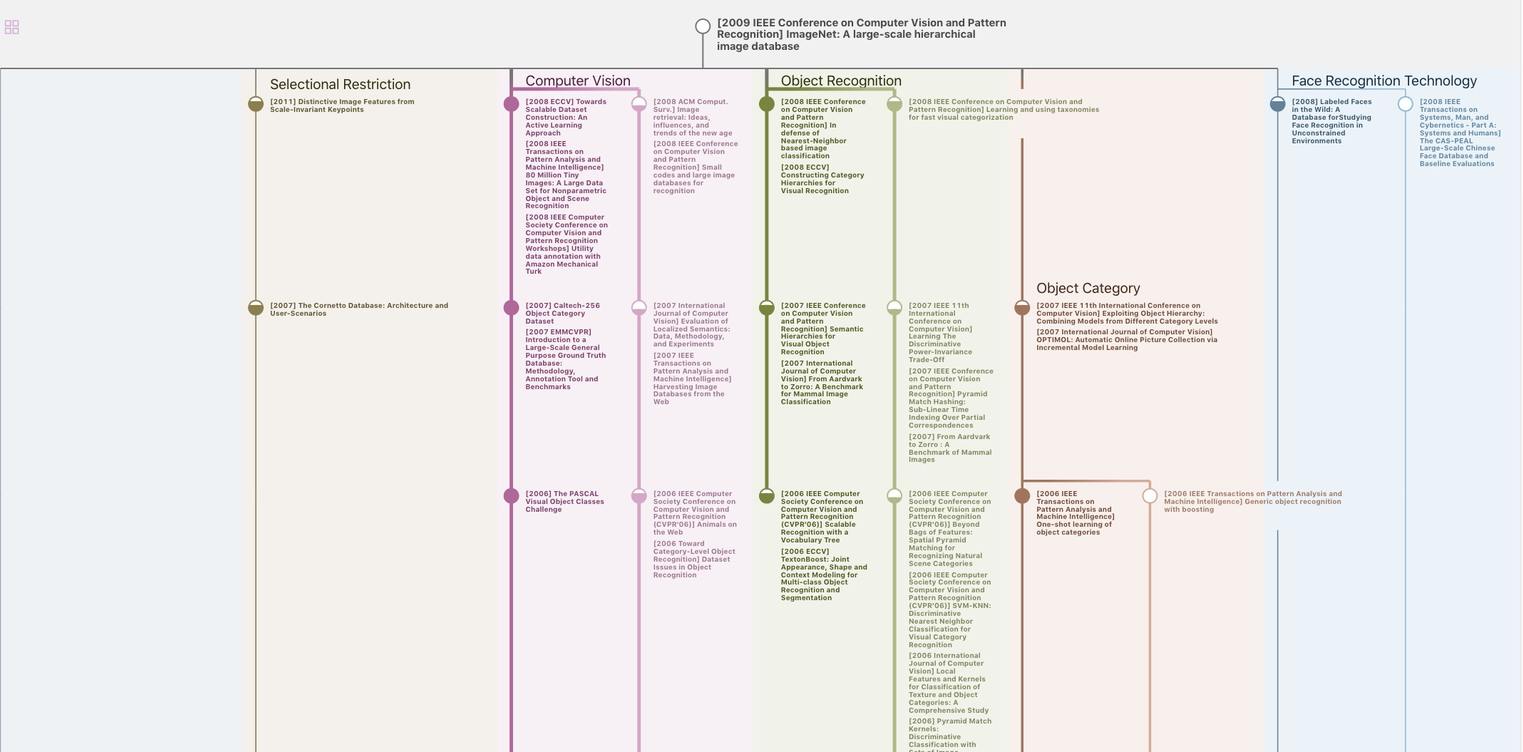
生成溯源树,研究论文发展脉络
Chat Paper
正在生成论文摘要