Lattice-Point Mutually Guided Ground-To-Aerial Feature Matching For Urban Scene Images
IEEE JOURNAL OF SELECTED TOPICS IN APPLIED EARTH OBSERVATIONS AND REMOTE SENSING(2021)
Abstract
Ground-to-aerial feature matching bridges information from cross-view images, which enables optimized urban applications, e.g., pixel-level geolocating and complete urban 3-D reconstruction. However, urban ground and aerial images typically suffer from drastic changes in viewpoint, scale, and illumination, together with repetitive patterns. Thus, direct matching of local features between ground and aerial images is particularly difficult because of the low similarity of local descriptors and high ambiguity in true-false match discrimination. For this challenging task, we propose a novel lattice-point mutually guided matching (LPMG) method in this article. We specifically address two key issues: 1) reducing descriptor variance and 2) enhancing true-false match discriminability. The former is solved by recovering the geometry and appearance of the underlying image region in 3-D through automatic view rectification on ground and aerial images. The latter is circumvented by replacing the conventional mismatch removal with an LPMG strategy. In this strategy, the topology structure of repeated facade elements (i.e., lattice), and the high reliable point matching seeds, are first extracted from the rectified ground and aerial images. Then, the point matching seeds guide the self-similar lattice tiles from two views to be precisely aligned, thereby estimating an accurate transformation model from lattice tile correspondences. Finally, the estimated model powerfully supervises the differentiation of true and false matches from the entire putative match set. Extensive experiments conducted on several datasets show that our method can obtain a considerable number of nearly pure correct matches from urban ground and aerial images, significantly outperforming those existing methods.
MoreTranslated text
Key words
Lattices, Feature extraction, Geology, Three-dimensional displays, Transforms, Task analysis, Reliability, Aerial oblique imagery, feature matching, ground imagery, ground-to-aerial image matching, repetitive pattern
AI Read Science
Must-Reading Tree
Example
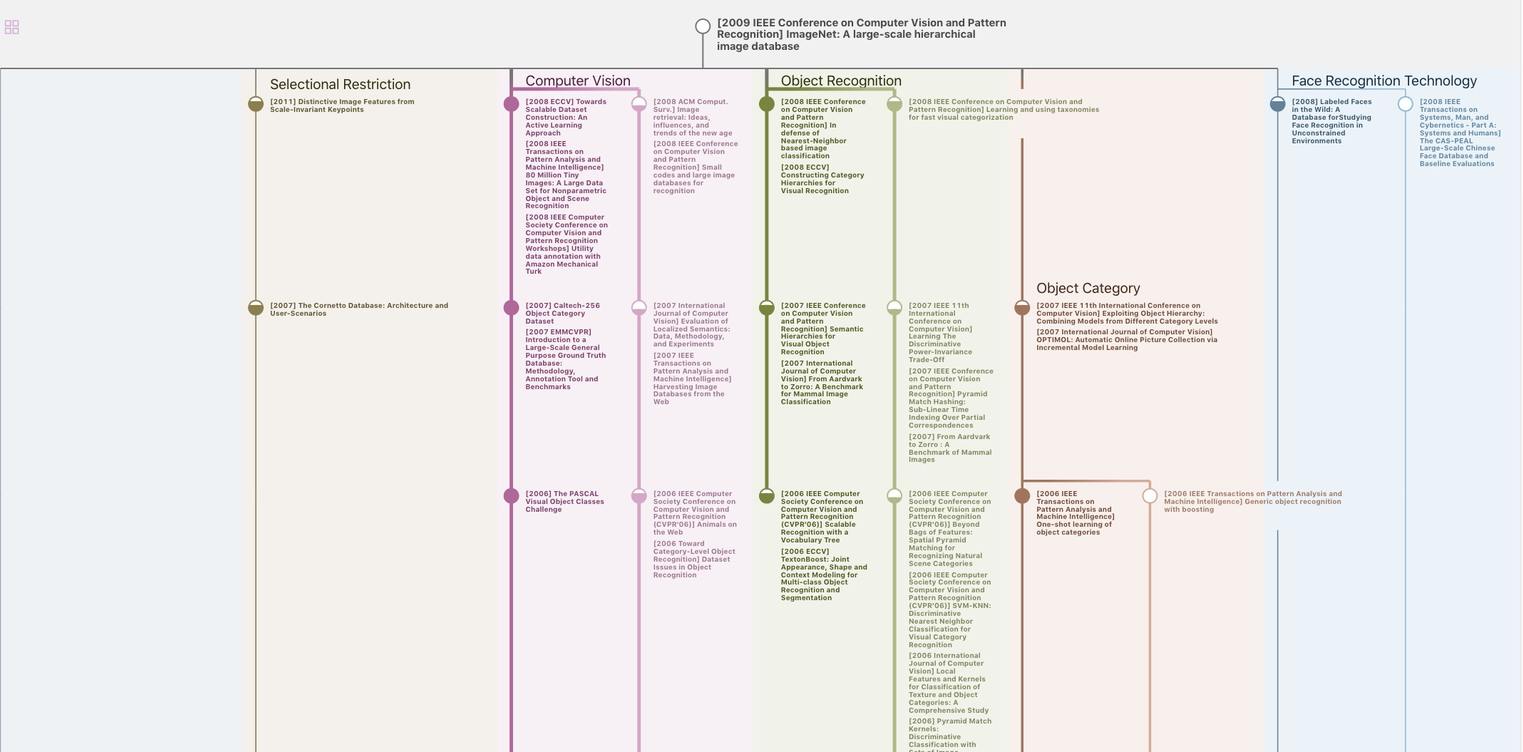
Generate MRT to find the research sequence of this paper
Chat Paper
Summary is being generated by the instructions you defined