Estimation of Ground NO2 Measurements from Sentinel-5P Tropospheric Data through Categorical Boosting
MetroXRAINE(2023)
摘要
Atmospheric pollution has been largely considered by the scientific community as a primary threat to human health and ecosystems, above all for its impact on climate change. Therefore, its containment and reduction are gaining interest and commitment from institutions and researchers, although the solutions are not immediate. It becomes of primary importance to identify the distribution of air pollutants and evaluate their concentration levels in order to activate the right countermeasures. Among other tools, satellite-based measurements have been used for monitoring and obtaining information on air pollutants, and over the years their performance has increased in terms of both resolution and data reliability. This study aims to analyze the NO2 pollution in the Emilia Romagna Region (Northern Italy) during 2019, with the help of satellite retrievals from the {\nobreak Sentinel\nobreak-5P} mission of the European Copernicus Programme and ground-based measurements, obtained from the ARPA site (Regional Agency for the Protection of the Environment). The final goal is the estimation of ground NO2 measurements when only satellite data are available. For this task, we used a Machine Learning (ML) model, Categorical Boosting, which was demonstrated to work quite well and allowed us to achieve a Root-Mean-Square Error (RMSE) of 0.0242 over the 43 stations utilized to get the Ground Truth values. This procedure, applicable to other areas of Italy and the world and on longer timelines, represents the starting point to understand which other actions must be taken to improve its final performance.
更多查看译文
关键词
Earth Observation,Machine Learning,Cat-egorical Boosting,NO2,TropOMI,Sentinel-5P,near-surface pollution
AI 理解论文
溯源树
样例
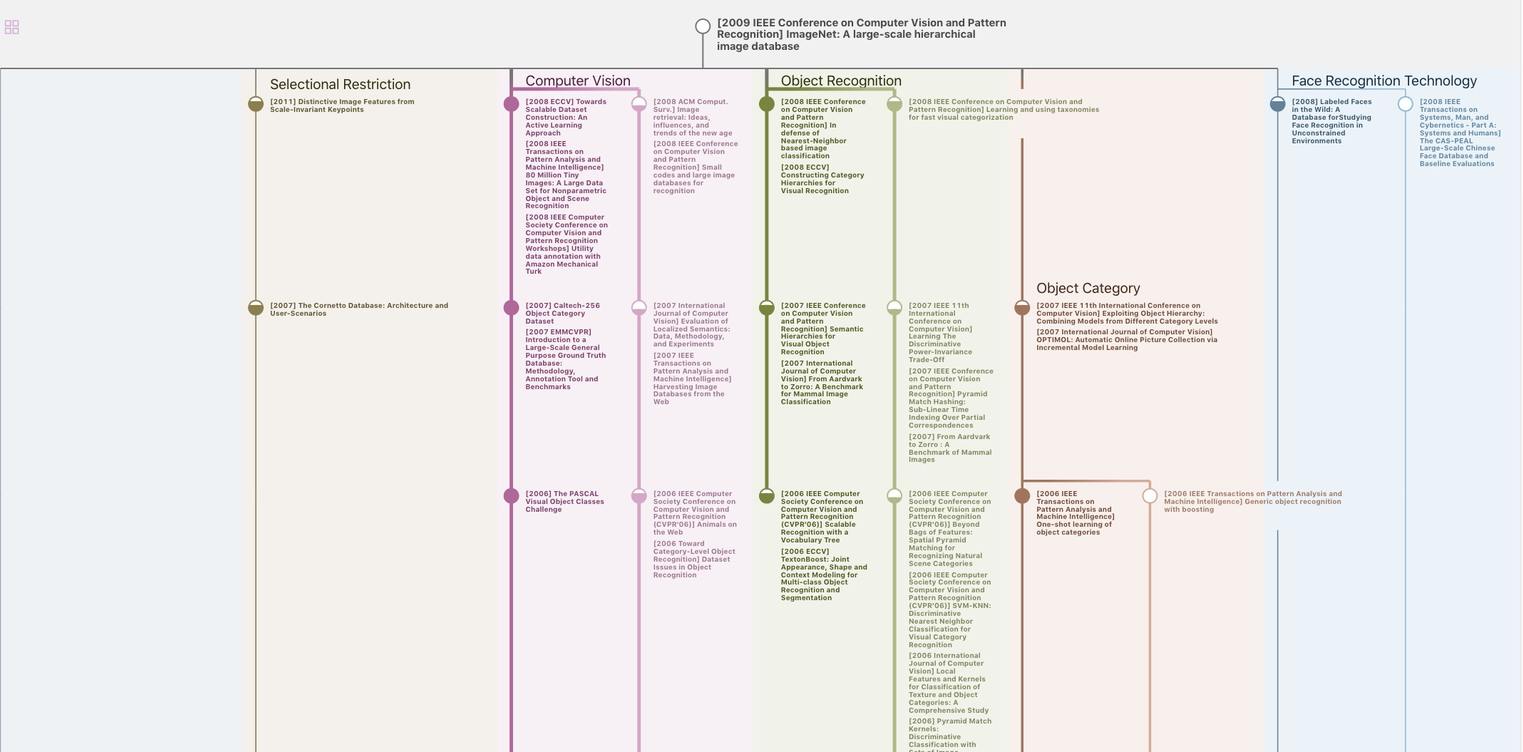
生成溯源树,研究论文发展脉络
Chat Paper
正在生成论文摘要