Learning to increase matching efficiency in identifying additional b-jets in the tt̅bb̅ process
ArXiv(2022)
摘要
The tt̅H(bb̅) process is an essential channel in revealing the Higgs boson properties; however, its final state has an irreducible background from the tt̅bb̅ process, which produces a top quark pair in association with a b quark pair. Therefore, understanding the tt̅bb̅ process is crucial for improving the sensitivity of a search for the tt̅H(bb̅) process. To this end, when measuring the differential cross section of the tt̅bb̅ process, we need to distinguish the b-jets originating from top quark decays and additional b-jets originating from gluon splitting. In this paper, we train deep neural networks that identify the additional b-jets in the tt̅bb̅ events under the supervision of a simulated tt̅bb̅ event data set in which true additional b-jets are indicated. By exploiting the special structure of the tt̅bb̅ event data, several loss functions are proposed and minimized to directly increase matching efficiency, i.e., the accuracy of identifying additional b-jets. We show that, via a proof-of-concept experiment using synthetic data, our method can be more advantageous for improving matching efficiency than the deep learning-based binary classification approach presented in [ 1 ]. Based on simulated tt̅bb̅ event data in the lepton+jets channel from pp collision at √(s) = 13 TeV, we then verify that our method can identify additional b-jets more accurately: compared with the approach in [ 1 ], the matching efficiency improves from 62.1 % to 64.5 % and from 59.9 % to 61.7 % for the leading order and the next-to-leading order simulations, respectively.
更多查看译文
关键词
b-jets
AI 理解论文
溯源树
样例
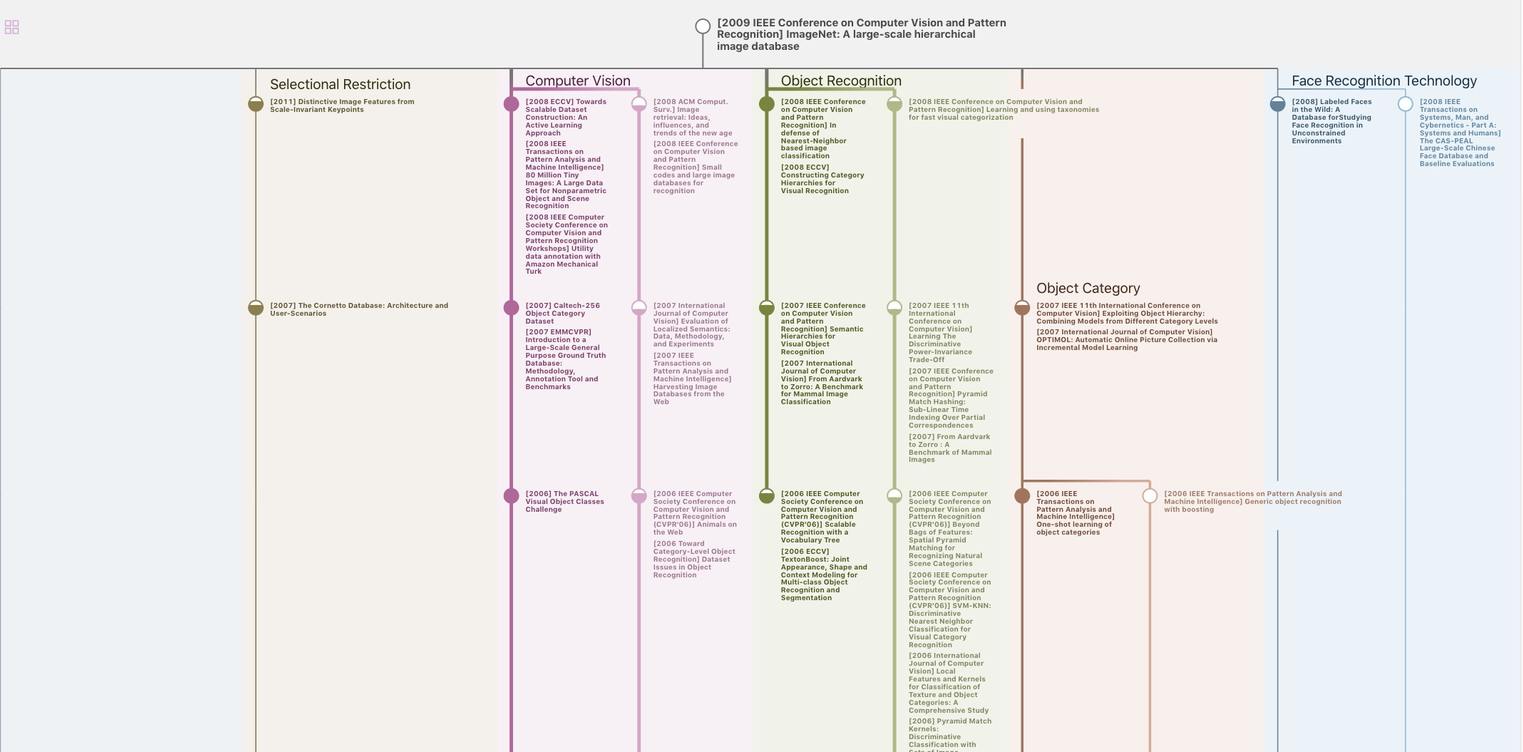
生成溯源树,研究论文发展脉络
Chat Paper
正在生成论文摘要