Self-supervised Domain Adaptation for Satellite Imagery Classification via Representation Learning
international conference on big data(2021)
摘要
Hyperspectral image (HSI) classification has been attracting widespread attention in the field of computer vision and achieved remarkable results due to the rapid development of deep learning. All existing HSI classification models are based on a closed set assumption where both the training and testing data are drawn from a known common domain. However, since the satellite imagery covers an extremely vast area, it is almost impossible to guarantee the representativeness of the training samples. To tackle the aforementioned problem, we propose a self-supervised domain adaptation model that recognizes the instances coming from unknown classes while maintaining accuracy on known classes. First, we employ a novel neighborhood representation learning to learn a discriminative representation in an unsupervised way. Second, we utilize an entropy separation loss to either match each target instance with a source prototype, or reject it as “unknown”. Experiments on multiple HSI benchmarks demonstrate the effectiveness and superiority of the proposed method.
更多查看译文
关键词
hyperspectral image,deep learning,self-supervised learning,remote sensing
AI 理解论文
溯源树
样例
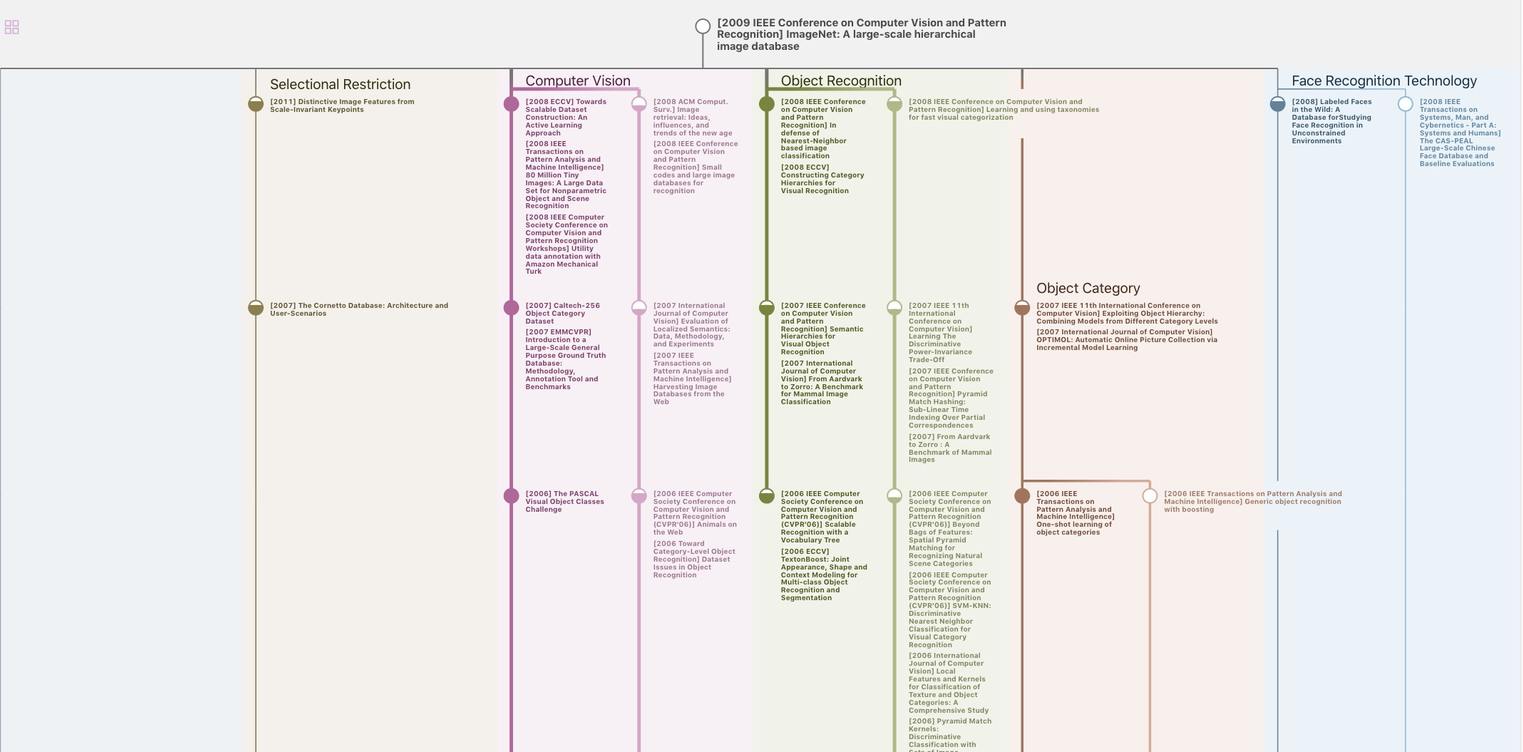
生成溯源树,研究论文发展脉络
Chat Paper
正在生成论文摘要