PieceNet: A Redundant UNet Ensemble.
BrainLes@MICCAI (2)(2020)
摘要
Segmentation of gliomas is essential to aid clinical diagnosis and treatment; however, imaging artifacts and heterogeneous shape complicate this task. In the last few years, researchers have shown the effectiveness of 3D UNets on this problem. They have found success using 3D patches to predict the class label for the center voxel; however, even a single patch-based UNet may miss representations that another UNet could learn. To circumvent this issue, I developed PieceNet, a deep learning model using a novel ensemble of patch-based 3D UNets. In particular, I used uncorrected modalities to train a standard 3D UNet for all label classes as well as one 3D UNet for each individual label class. Initial results indicate this 4-network ensemble is potentially a superior technique to a traditional patch-based 3D UNet on uncorrected images; however, further work needs to be done to allow for more competitive enhancing tumor segmentation. Moreover, I developed a linear probability model using radiomic and non-imaging features that predicts post-surgery survival.
更多查看译文
关键词
UNet,Multilabel,Patches
AI 理解论文
溯源树
样例
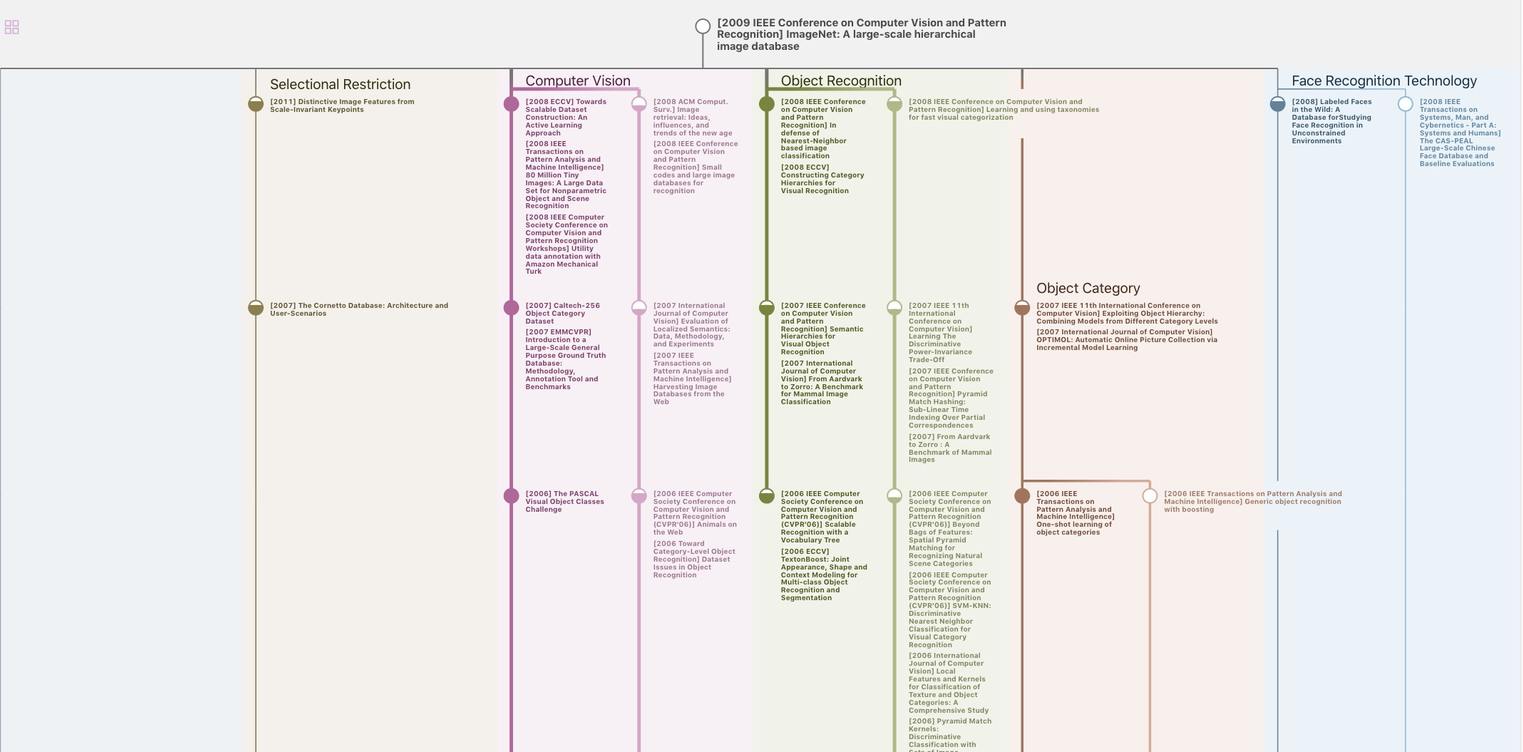
生成溯源树,研究论文发展脉络
Chat Paper
正在生成论文摘要