Enhancing Factual Consistency of Abstractive Summarization
NAACL-HLT(2021)
摘要
Automatic abstractive summaries are found to often distort or fabricate facts in the article. This inconsistency between summary and original text has seriously impacted its applicability. We propose a fact-aware summarization model FASUM to extract and integrate factual relations into the summary generation process via graph attention. We then design a factual corrector model FC to automatically correct factual errors from summaries generated by existing systems. Empirical results' show that the fact-aware summarization can produce abstractive summaries with higher factual consistency compared with existing systems, and the correction model improves the factual consistency of given summaries via modifying only a few keywords.
更多查看译文
关键词
Machine Translation,Semantic Similarity,Statistical Machine Translation,Word Representation,Language Modeling
AI 理解论文
溯源树
样例
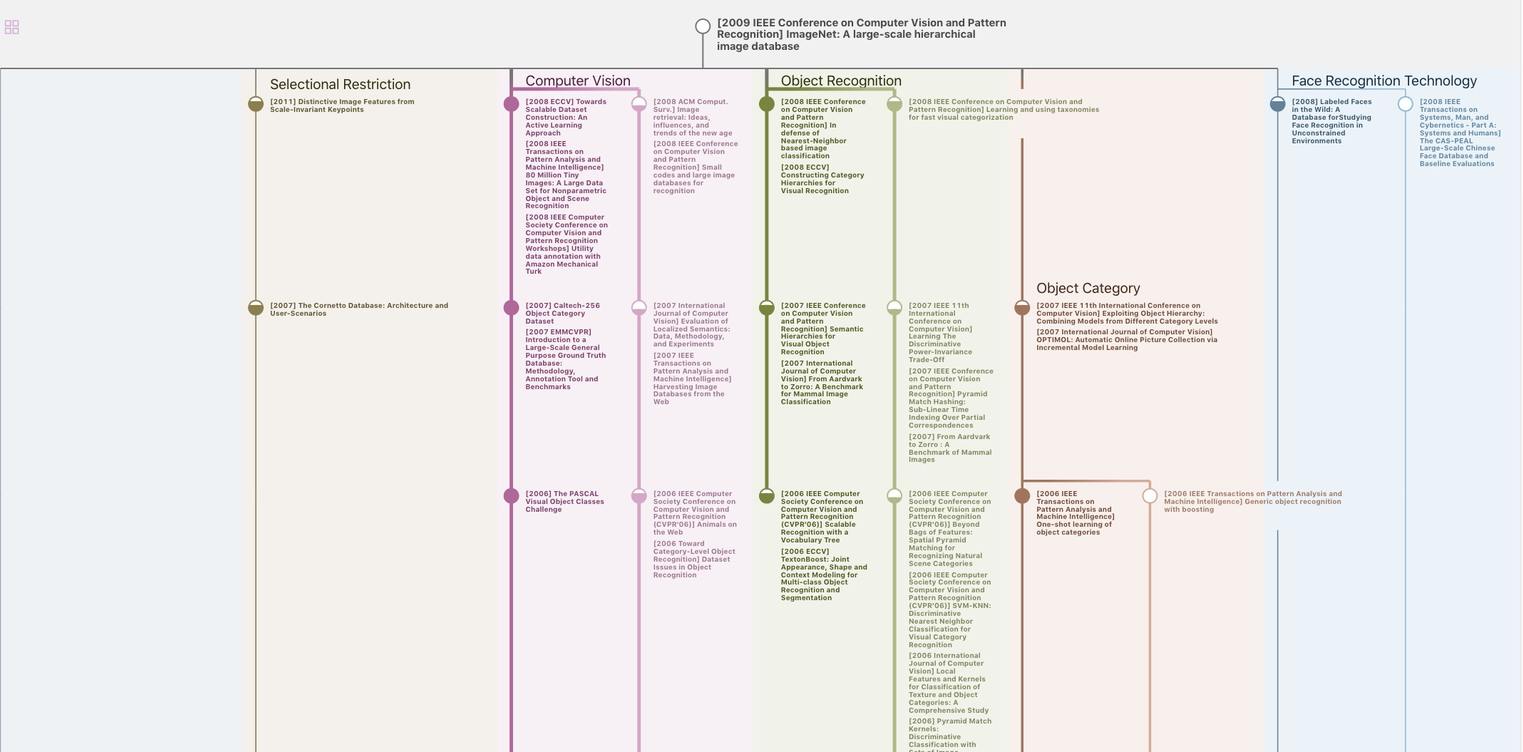
生成溯源树,研究论文发展脉络
Chat Paper
正在生成论文摘要