New Algorithms for the Estimation of Two-Dimensional Cyclic Spectral Information Based on Tensor Equations.
J. Signal Process. Syst.(2021)
摘要
The hidden periodicity detection using a cyclic spectral function (CSF) is one of the well-known methods for cyclostationary signals analysis. Many two-dimensional signals (2D-signals), such as textures, can be considered cyclostationary or semi-cyclostationary. Applying such an assumption provides a new effective field for the analysis of 2D-signals. This paper presents two new algorithms for two-dimensional CSF (2D-CSF) estimation, namely two-dimensional tensor-based FFT accumulation method (2DT-FAM) and two-dimensional tensor-based strip spectral correlation analyzer (2DT-SSCA). These algorithms are fast and parallel. Moreover, they are based on tensor equations and linear-algebra that provide many advantages in the computational efficiency. Furthermore, the proposed schemes produce more information by preserving the information between pixels of an image using a two-dimensional window that improves classification accuracy and noise resistance property. To evaluate the performance of proposed algorithms, they are employed on two popular databases in texture analysis. 2DT-FAM as the unused promising texture analyzer and the new implementation of two-dimensional strip spectral correlation analyzer 2D-SSCA are compared with other state-of-the-art methods in terms of processing time, noise resistance, and classification accuracy. Experiment results show a 2% increase in correct classification rate and 10 times reduction in input feature dimensions in comparison with other studies and a 0.24 s decrease in processing times in comparison with the ordinary two-dimensional SSCA.
更多查看译文
关键词
2D-CSF,Tensor,Texture analysis,2DT-FAM,2DT-SSCA
AI 理解论文
溯源树
样例
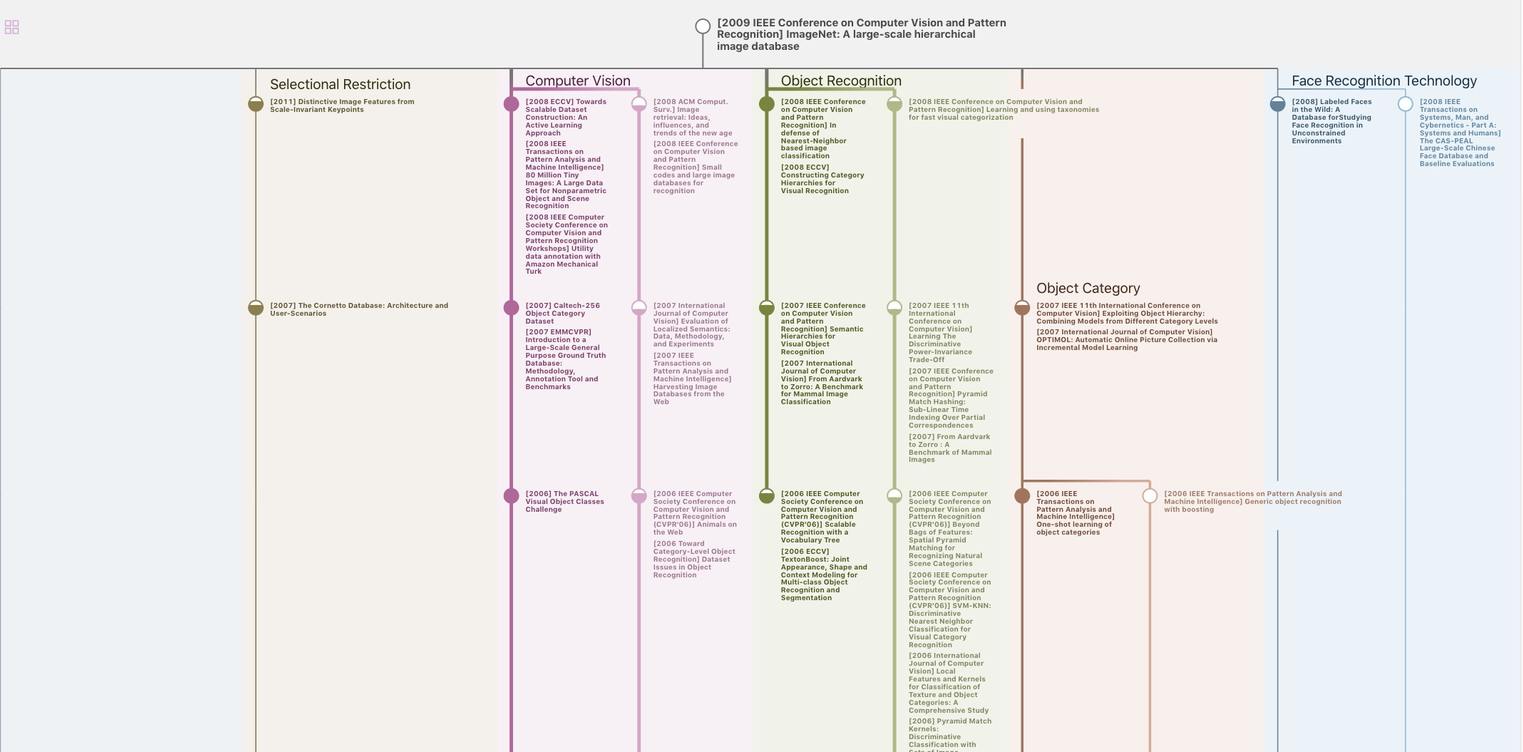
生成溯源树,研究论文发展脉络
Chat Paper
正在生成论文摘要