Distributed Convex Optimization With State-Dependent (Social) Interactions And Time-Varying Topologies
IEEE TRANSACTIONS ON SIGNAL PROCESSING(2021)
摘要
In this paper, an unconstrained collaborative optimization of a sum of convex functions is considered where agents make decisions using local information from their neighbors. The communication between nodes are described by a time-varying sequence of possibly state-dependent weighted networks. A new framework for modeling multi-agent optimization problems over networks with state-dependent interactions and time-varying topologies is proposed. A gradient-based discrete-time algorithm using diminishing step size is proposed for converging to the optimal solution under suitable assumptions. The algorithm is totally asynchronous without requiring B-connectivity assumption for convergence. The algorithm still works even if the weighted matrix of the graph is periodic and irreducible in synchronous protocol. Finally, a case study on a network of robots in an automated warehouse is provided in order to demonstrate the results.
更多查看译文
关键词
Distributed optimization, convex optimization, state-dependent networks, time-varying topologies
AI 理解论文
溯源树
样例
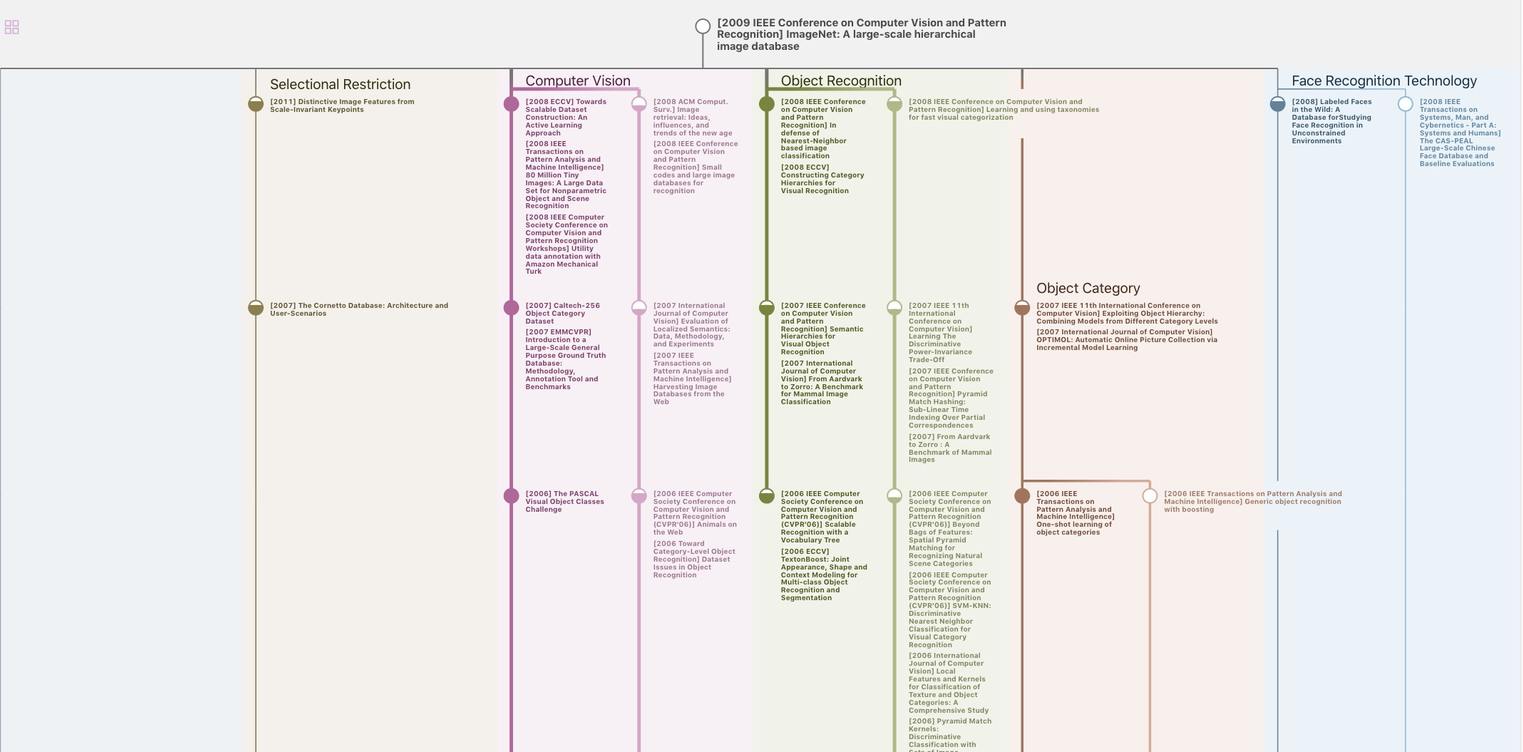
生成溯源树,研究论文发展脉络
Chat Paper
正在生成论文摘要