Deep learning for the estimation of water-levels using river cameras
Hydrology and Earth System Sciences Discussions(2021)
摘要
Abstract. River level estimation is a critical task required for the understanding of flood events, and is often complicated by the scarcity of available data. Recent studies have proposed to take advantage of large networks of river camera images to estimate the river levels, but currently, the utility of this approach remains limited as it requires a large amount of manual intervention (ground topographic surveys and water image annotation). We develop an approach using an automated water semantic segmentation method to ease the process of river level estimation from river camera images. Our method is based on the application of a transfer learning methodology to deep semantic neural networks designed for water segmentation. Using datasets of image series extracted from four river cameras and manually annotated for the observation of a flood event on the Severn and Avon rivers, UK (21 November–5 December 2012), we show that our algorithm is able to automate the annotation process with an accuracy greater than 91 %. Then, we apply our approach to year-long image series from the same cameras observing the Severn and Avon (from 1 June 2019 to 31 May 2020) and compare our results with nearby river-gauge measurements. Given the high correlation (Pearson's Correlation Coefficient > 0.94) between our results and the river-gauge measurements, it is clear that our approach to automation of the water segmentation on river camera images could allow for straightforward, inexpensive observation of flood events, especially at ungauged locations.
更多查看译文
AI 理解论文
溯源树
样例
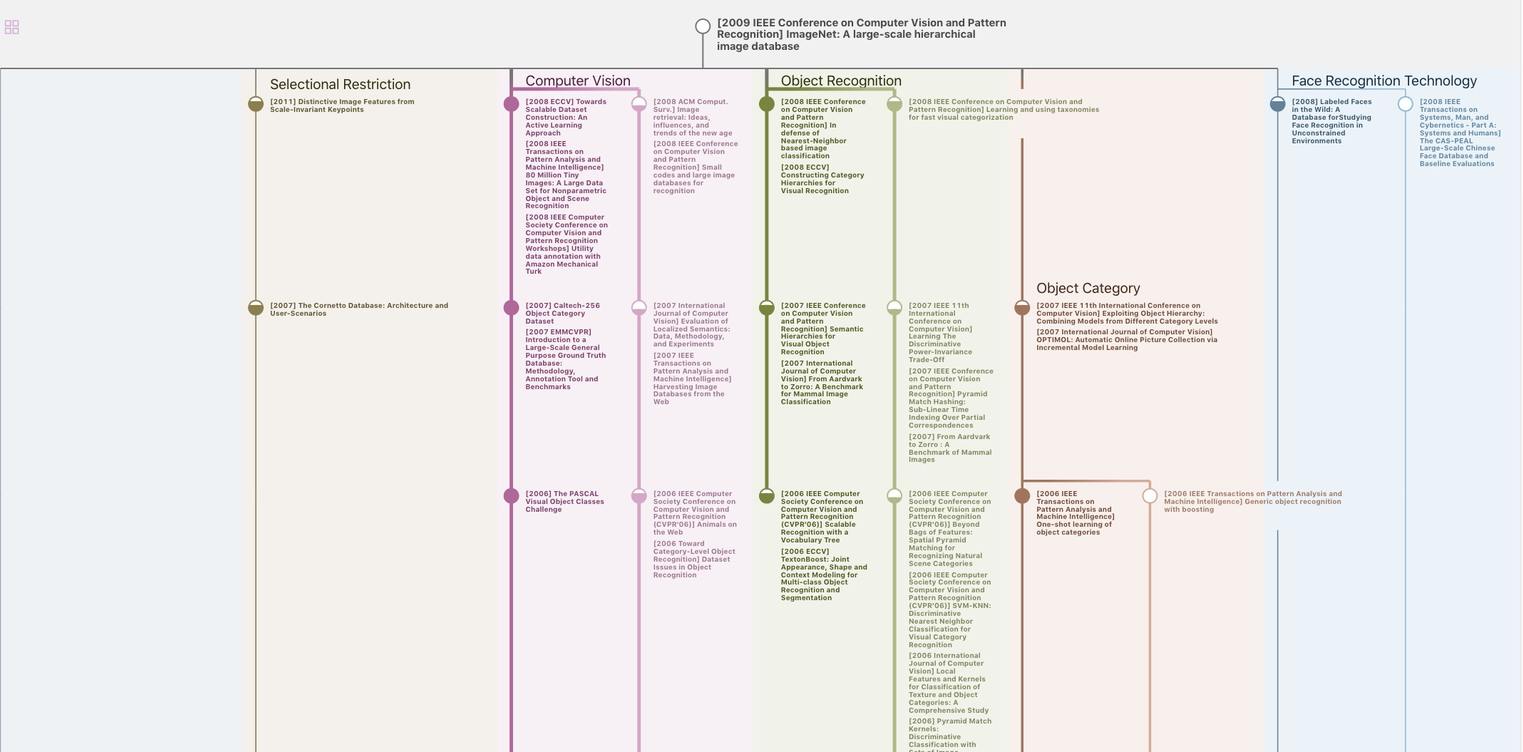
生成溯源树,研究论文发展脉络
Chat Paper
正在生成论文摘要