Machine Learning Approach For The Prediction Of Electron Inelastic Mean Free Paths
PHYSICAL REVIEW MATERIALS(2021)
摘要
The prediction of electron inelastic mean free paths (IMFPs) from simple material parameters is a challenging problem in studies using electron spectroscopy and microscopy. Herein, we propose a machine learning (ML) approach to predict IMFPs from some basic material property data. The ML model showed excellent performance based on the calculated IMFPs for a group of 41 elemental materials [Li, Be, C (graphite), C (diamond), C (glassy), Na, Mg, Al, Si, K, Sc, Ti, V, Cr, Fe, Co, Ni, Cu, Ge, Y, Nb, Mo, Ru, Rh, Pd, Ag, In, Sn, Cs, Gd, Tb, Dy, Hf, Ta, W, Re, Os, Ir, Pt, Au, and Bi] from a previous paper [Shinotsuka et al., Surf. Interface Anal. 47, 871 (2015); 47, 1132 (2015)]. which was comparable with that of the robust Tanuma-Powell-Penn (TPP-2M) formula. The developed ML model was then extended to materials that do not have reported IMFPs in the database. The IMFPs for 18 transition and lanthanide metals (Mn, Zn, Zr, Tc, Cd, La, Ce, Pr, Nd, Pm, Sm, Eu, Ho, Er, Tm, Yb, Lu, and Hg) were predicted by theML model. In the comparison with full-Penn algorithm-calculated IMFPs through two newly found experimental energy loss functions (ELFs), i.e., Mn and Zr, the Gaussian process regression-predicted IMFPs not only agreed well with those calculated using the TPP-2M formula in the energy range >50 eV but were also consistent with the trend of IMFPs calculated through experimental ELFs in the range of 2.7-50 eV, where the TPP-2M formula cannot be used. Our findings suggest that ML is very powerful and efficient and has great potential to complete a database of IMFPs for materials that can prove solutions closer to reality than empirical models on materials with similar physical and chemical properties and can be applied to other different situations for correlated information prediction.
更多查看译文
AI 理解论文
溯源树
样例
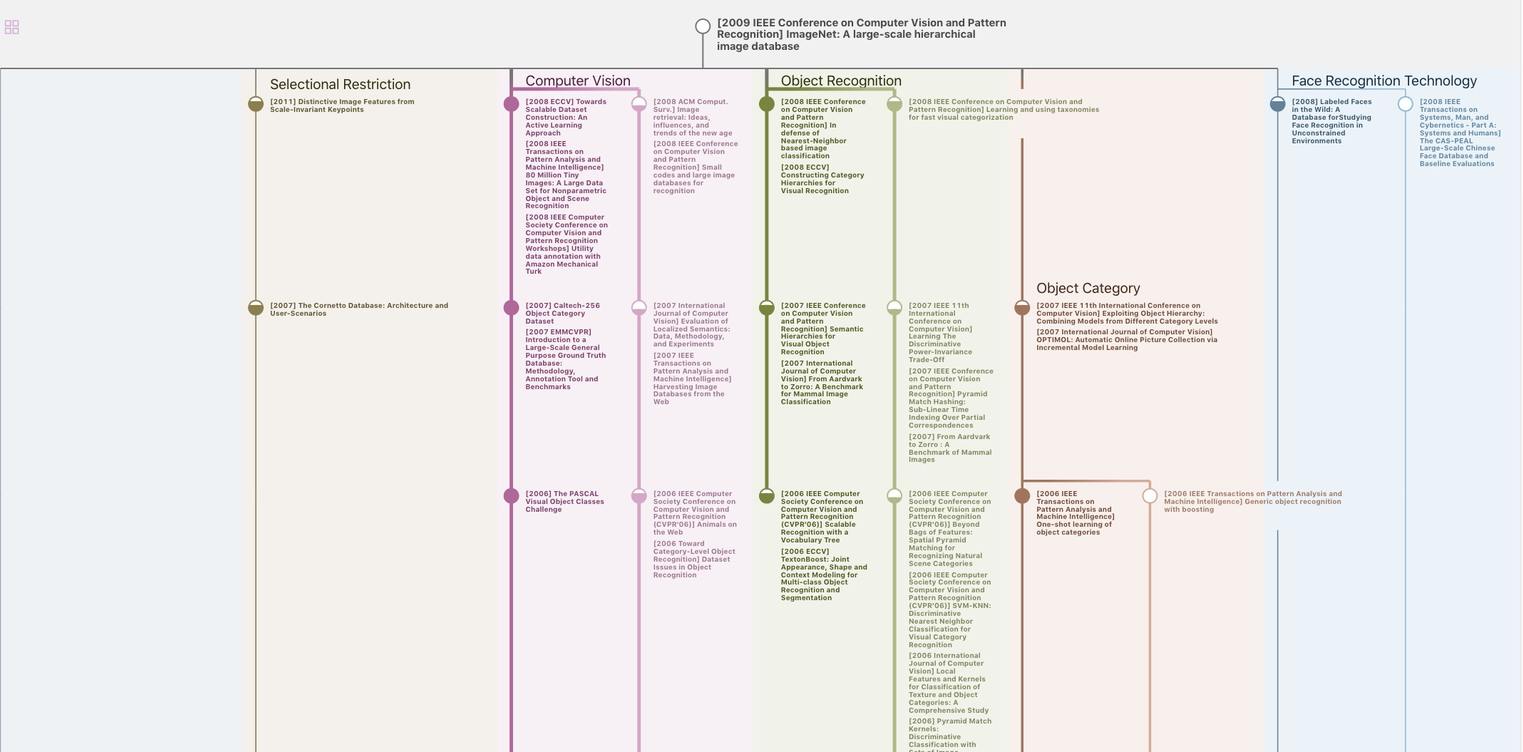
生成溯源树,研究论文发展脉络
Chat Paper
正在生成论文摘要