An Energy Efficient Computing-in-Memory Accelerator With 1T2R Cell and Fully Analog Processing for Edge AI Applications
IEEE Transactions on Circuits and Systems II: Express Briefs(2021)
摘要
In this work, a ReRAM-based energy-efficient CIM accelerator is presented with two techniques for edge AI applications. Firstly, a circuit-algorithm co-design scheme is proposed to realize fully analog processing, which improves the energy efficiency and the throughput of neural network. To deal with the I-V nonlinearity of ReRAM, a nonlinear-aware training algorithm is proposed to improve the network accuracy. Secondly, a 1T2R cell is proposed to replace previous 2T2R for weight storage with 35% area saving. For evaluation, a neural network with two fully connected layers and one ReLU layer is built for the MNIST dataset. The error rate can be reduced by >46% and the energy efficiency is 99 TOPS/W@200 MHz, 2.6X improvement over the digital method.
更多查看译文
关键词
ReRAM,1T2R,neural network (NN),computing-in-memory (CIM),machine learning (ML)
AI 理解论文
溯源树
样例
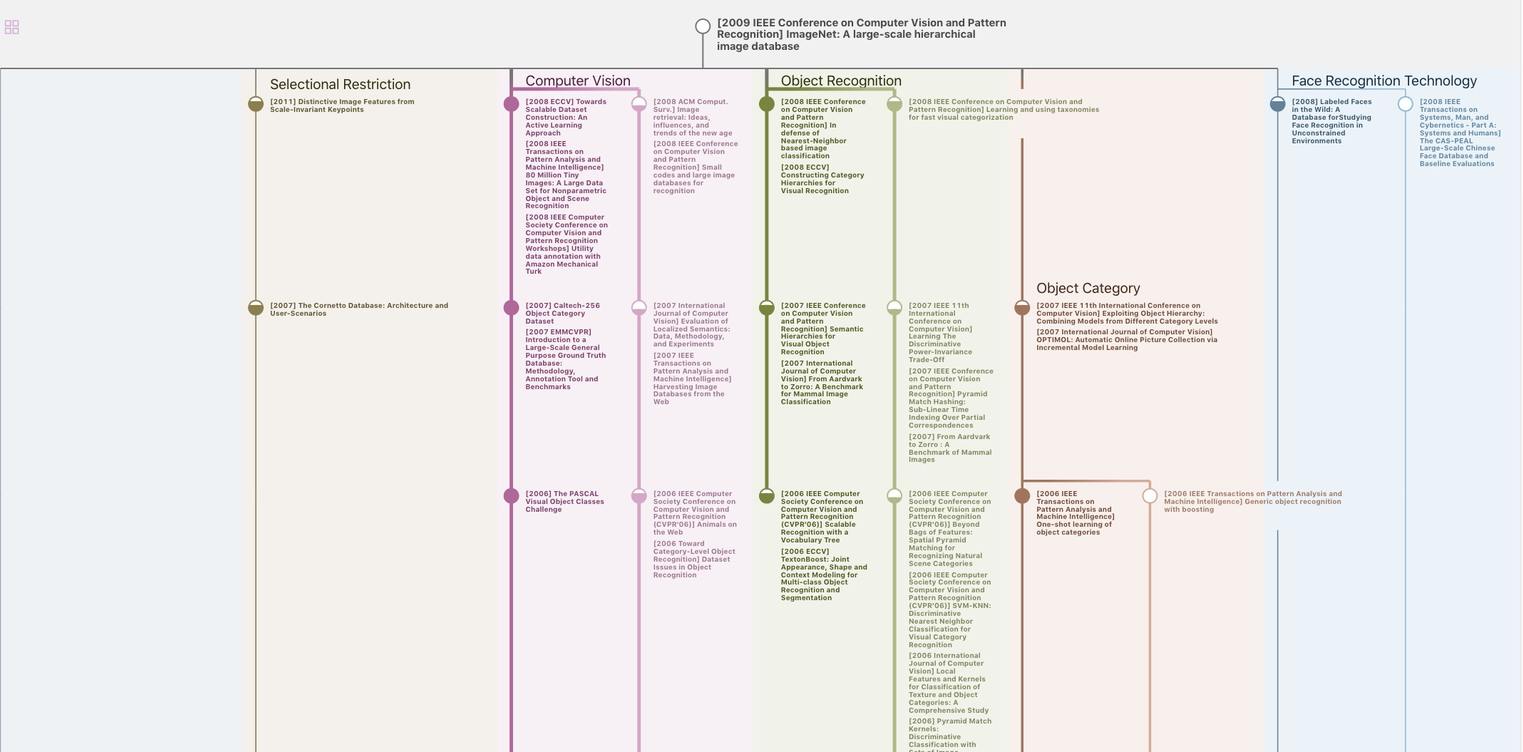
生成溯源树,研究论文发展脉络
Chat Paper
正在生成论文摘要