Experimental analysis of machine learning methods for credit score classification
PROGRESS IN ARTIFICIAL INTELLIGENCE(2021)
摘要
Credit scoring concerns with emerging empirical model to assist the financial institutions for financial decision-making process. Credit risk analysis plays a vital role for decision-making process; statistical and machine learning approaches are utilized to estimate the risk associated with a credit applicant. Enhancing the performance of credit scoring model, particularly toward non-trustworthy “or non-creditworthy” group, may result incredible effect for financial institution. However, credit scoring data may have excess and unimportant data and features which degrades the performance of model. So, selection of important features (or reduction in irrelevant and redundant features) may play the key role for improving the effectiveness and reducing the complexity of the model. This study presents a experimental results analysis of various combinations of feature selection approaches with various classification approaches and impact of feature selection approaches. For experimental results analysis, nine feature selection and sixteen classification state-of-the-art approaches have been applied on seven benched marked credit scoring datasets.
更多查看译文
关键词
Classification,Credit scoring,Feature selection
AI 理解论文
溯源树
样例
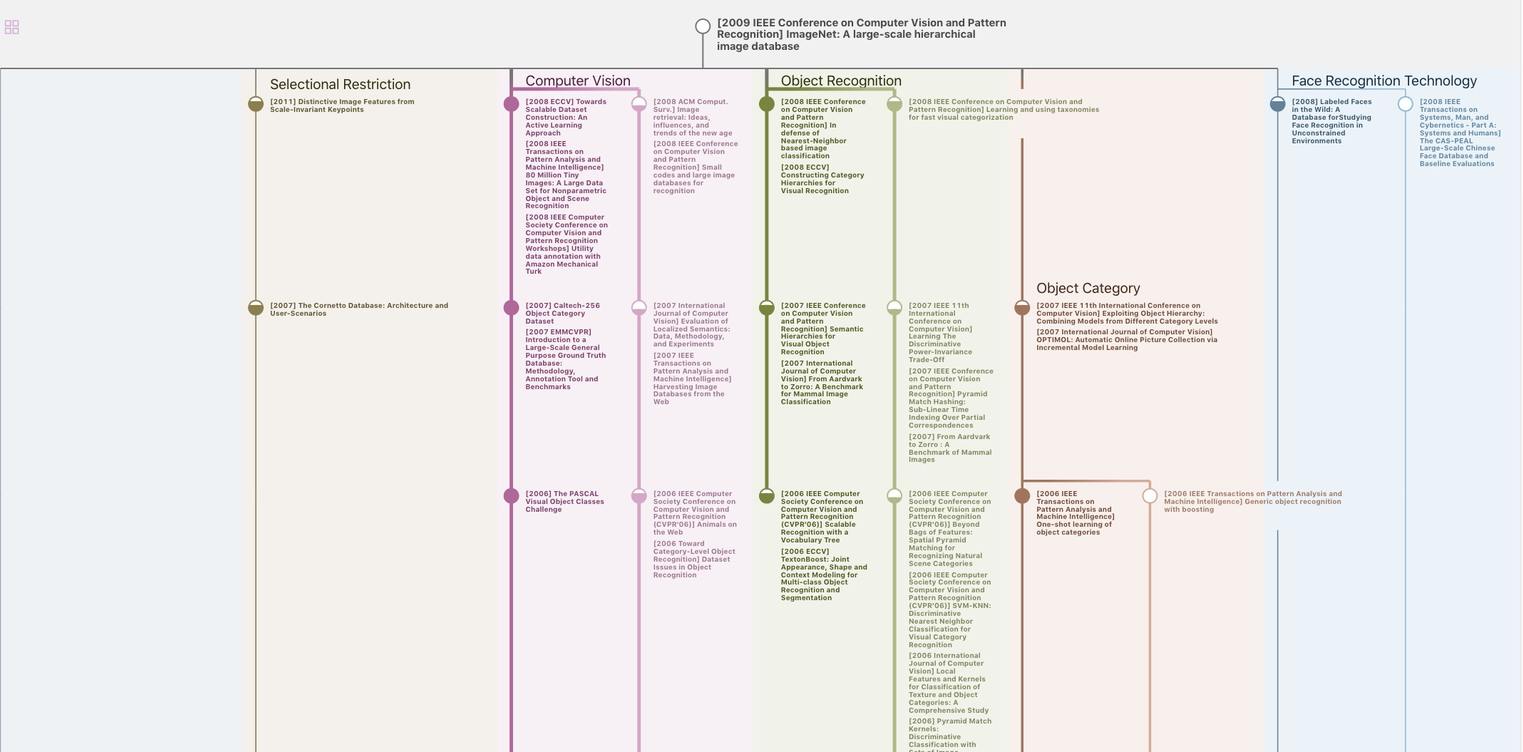
生成溯源树,研究论文发展脉络
Chat Paper
正在生成论文摘要