Chempix: Automated Recognition Of Hand-Drawn Hydrocarbon Structures Using Deep Learning
ChemRxiv(2021)
摘要
Inputting molecules into chemistry software, such as quantum chemistry packages, currently requires domain expertise, expensive software and/or cumbersome procedures. Leveraging recent breakthroughs in machine learning, we develop ChemPix: an offline, hand-drawn hydrocarbon structure recognition tool designed to remove these barriers. A neural image captioning approach consisting of a convolutional neural network (CNN) encoder and a long short-term memory (LSTM) decoder learned a mapping from photographs of hand-drawn hydrocarbon structures to machine-readable SMILES representations. We generated a large auxiliary training dataset, based on RDKit molecular images, by combining image augmentation, image degradation and background addition. Additionally, a small dataset of similar to 600 hand-drawn hydrocarbon chemical structures was crowd-sourced using a phone web application. These datasets were used to train the image-to-SMILES neural network with the goal of maximizing the hand-drawn hydrocarbon recognition accuracy. By forming a committee of the trained neural networks where each network casts one vote for the predicted molecule, we achieved a nearly 10 percentage point improvement of the molecule recognition accuracy and were able to assign a confidence value for the prediction based on the number of agreeing votes. The ensemble model achieved an accuracy of 76% on hand-drawn hydrocarbons, increasing to 86% if the top 3 predictions were considered.
更多查看译文
关键词
hydrocarbon structures,deep learning,recognition,hand-drawn
AI 理解论文
溯源树
样例
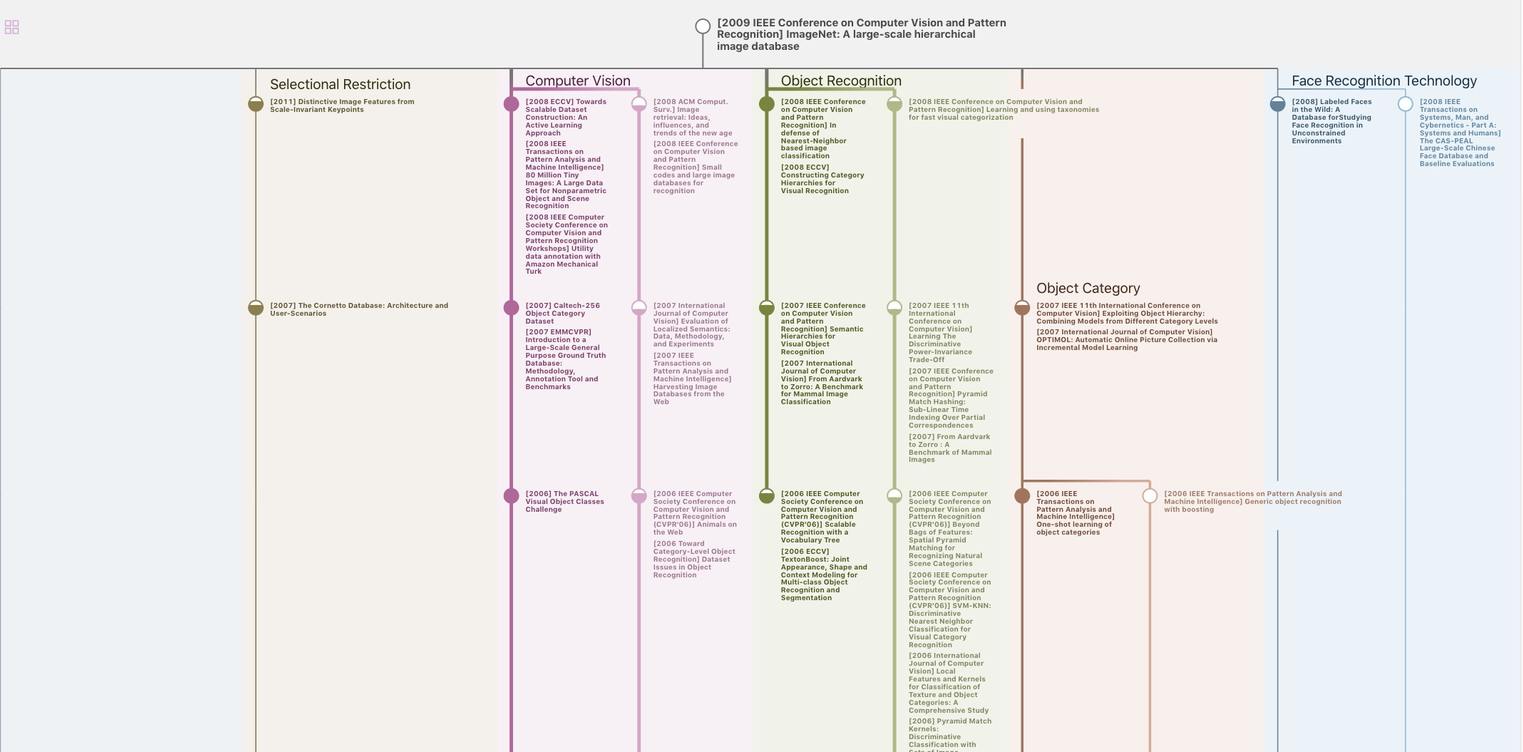
生成溯源树,研究论文发展脉络
Chat Paper
正在生成论文摘要