Making Costly Manufacturing Smart With Transfer Learning Under Limited Data: A Case Study On Composites Autoclave Processing
JOURNAL OF MANUFACTURING SYSTEMS(2021)
摘要
The integration of advanced manufacturing processes with ground-breaking Artificial Intelligence methods continue to provide unprecedented opportunities towards modern cyber-physical manufacturing processes, known as smart manufacturing or Industry 4.0. However, the "smartness" level of such approaches closely depends on the degree to which the implemented predictive models can handle uncertainties and production data shifts in the factory over time. In the case of change in a manufacturing process configuration with no sufficient new data, conventional Machine Learning (ML) models often tend to perform poorly. In this article, a transfer learning (TL) framework is proposed to tackle the aforementioned issue in modeling smart manufacturing. Namely, the proposed TL framework is able to adapt to probable shifts in the production process design and deliver accurate predictions without the need to re-train the model. Armed with sequential unfreezing and early stopping methods, the model demonstrated the ability to avoid catastrophic forgetting in the presence of severely limited data. Through the exemplified industry-focused case study on autoclave composite processing, the model yielded a drastic (88%) improvement in the generalization accuracy compared to the conventional learning, while reducing the computational and temporal cost by 56%.
更多查看译文
关键词
Intelligent manufacturing, Transfer learning, Limited data, Autoclave processing, Aerospace composites
AI 理解论文
溯源树
样例
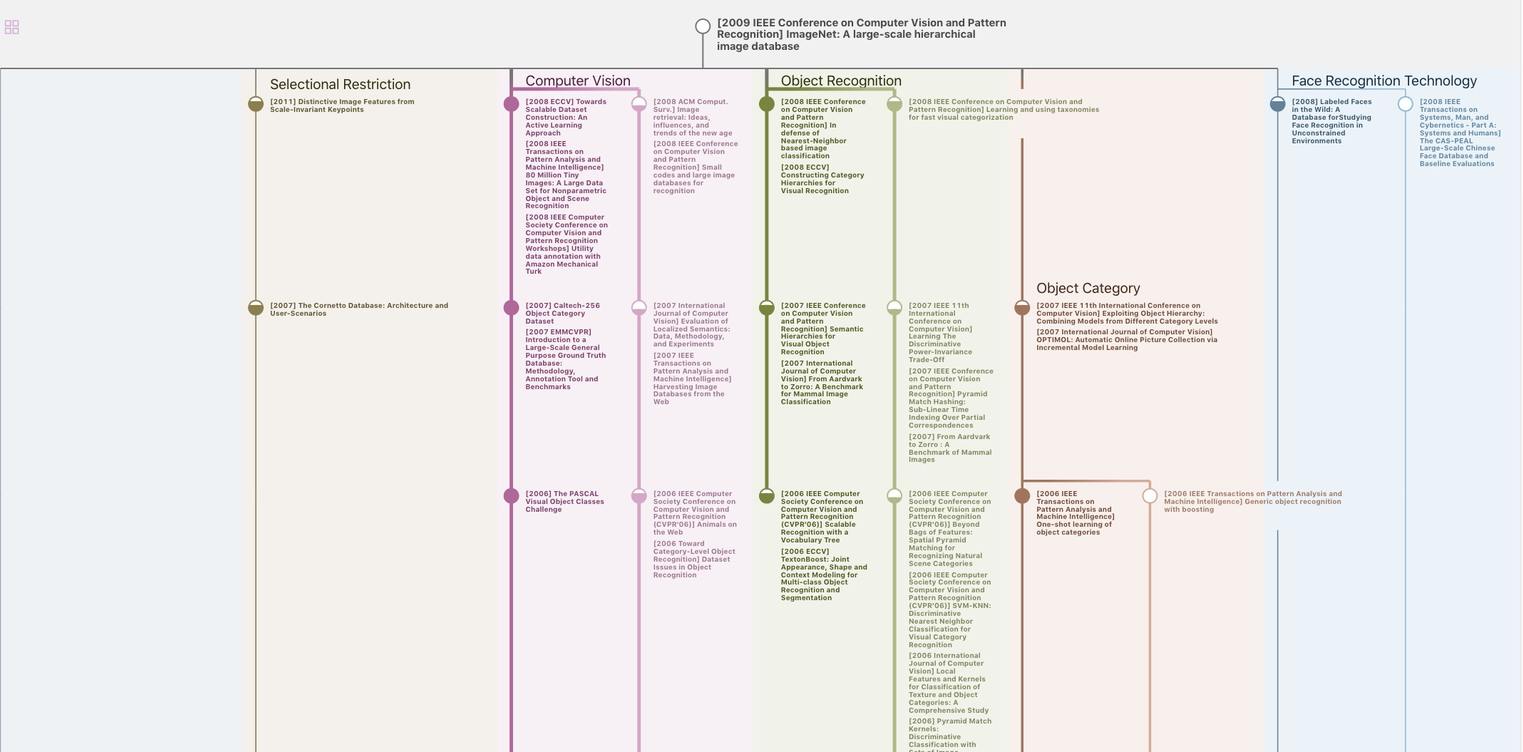
生成溯源树,研究论文发展脉络
Chat Paper
正在生成论文摘要