A United CNN-LSTM Algorithm Combining RR Wave Signals to Detect Arrhythmia in the 5G-Enabled Medical Internet of Things
IEEE Internet of Things Journal(2022)
Abstract
Arrhythmia (ARR) is the defective origin and conduction of heart activity leading to an abnormal frequency and rhythm of heartbeats. ARR can cause chest tightness, weakness, sinoatrial node blockages, tachycardia and even sudden death. ARR, therefore, seriously affects the safety of human life. An electrocardiogram (ECG) can record the changes in electrical activity produced in each heartbeat cycle. Due to its simplicity and noninvasiveness, ECGs are used clinically to diagnose ARRs. However, the diagnosis of ARR by experts is an inefficient diagnostic method. Heart rate variability (HRV) analysis is a common method for analyzing heart-related diseases, especially for the automatic diagnosis of ARR based on RR intervals. In this article, we extracted the linear and nonlinear characteristics collected from the 5G-enabled Medical Internet of Things to construct a time-frequency spectrogram from HRV sequences and used a deep learning model based on the combination of a deep convolutional neural network (CNN) and a long short-term memory (LSTM) network in order to classify normal sinus intervals and ARR intervals. The average accuracy, sensitivity and specificity of this model were 99.06%, 98.29%, and 99.73%, respectively, using a tenfold cross validation strategy. The united CNN-LSTM model can accurately detect ARR and has potential value in clinical applications.
MoreTranslated text
Key words
5G-enabled Medical Internet of Things,arrhythmia (ARR),convolutional neural network (CNN),heart rate variability (HRV),long short-term memory (LSTM) network,RR wave signals
AI Read Science
Must-Reading Tree
Example
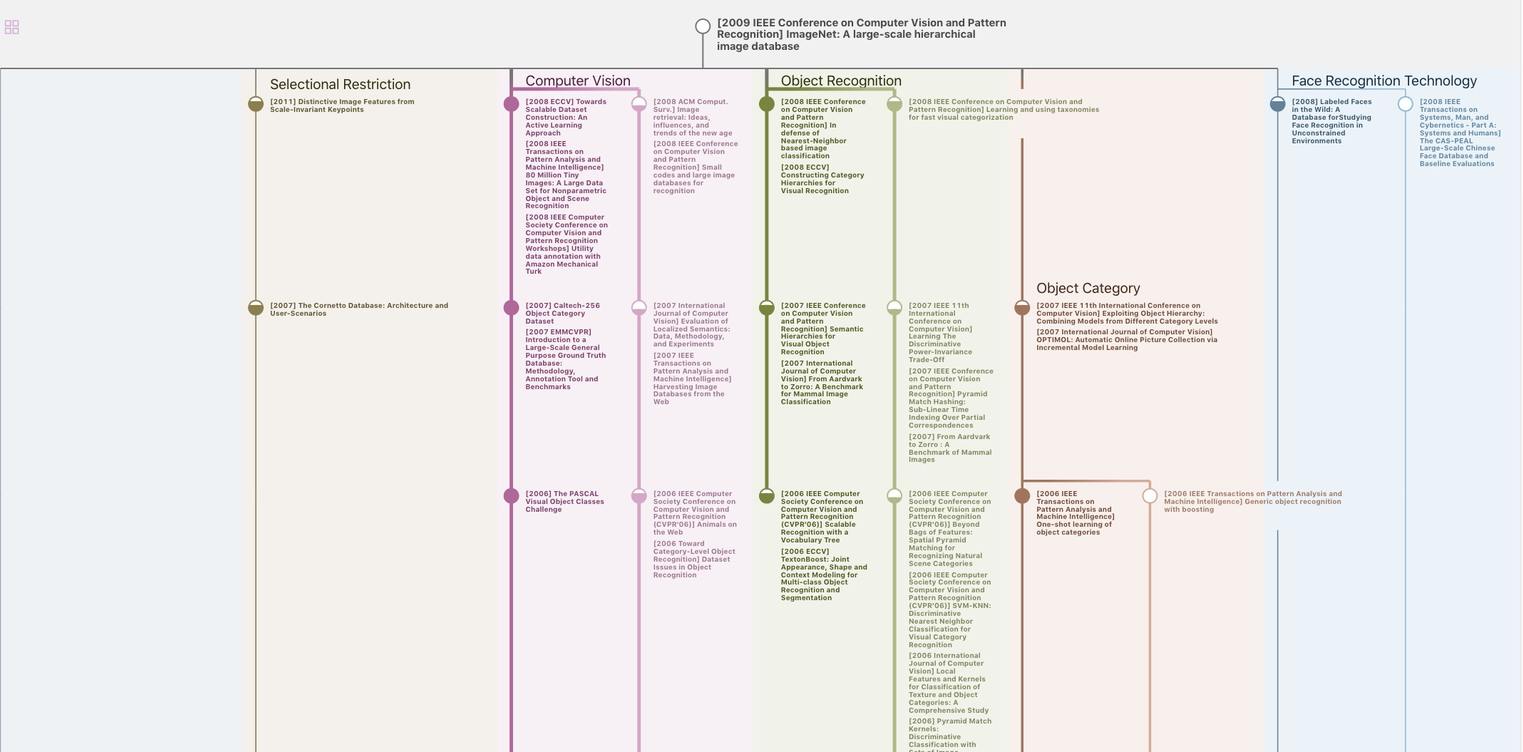
Generate MRT to find the research sequence of this paper
Chat Paper
Summary is being generated by the instructions you defined