Neural Guided Visual Slam System With Laplacian Of Gaussian Operator
IET COMPUTER VISION(2021)
摘要
Simultaneous localization and mapping (SLAM) addresses the problem of constructing the map from noisy sensor data and tracking the robot's path within the built map. After decades of development, a lot of mature systems achieve competent results in feature-based implementations. However, there are still problems when migrating the technology to practical applications. One typical example is the accuracy and robustness of SLAM in environment with illuminance and texture variations. To this end, two modules in the existing systems are improved here namely tracking and camera relocalization. In tracking module, image pyramid is processed with Laplacian of Gaussian (LoG) operator in feature extraction for enhanced edges and details. A majority voting mechanism is proposed to dynamically evaluate and redetermine the zero-mean sum of square difference threshold according to the matching error estimation in patch search. In camera relocalization module, full convolutional neural network which focuses on certain parts of the input data is utilized in guiding for accurate output predictions. The authors implement the two modules into OpenvSLAM and propose a neural guided visual SLAM system named LoG-SLAM. Experiments on publicly available datasets show that the accuracy and efficiency increase with LoG-SLAM when compared with other feature-based methods, and relocalization accuracy also improves compared with the recently proposed deep learning pipelines.
更多查看译文
关键词
cameras,feature extraction,learning (artificial intelligence),mobile robots,neural nets,pose estimation
AI 理解论文
溯源树
样例
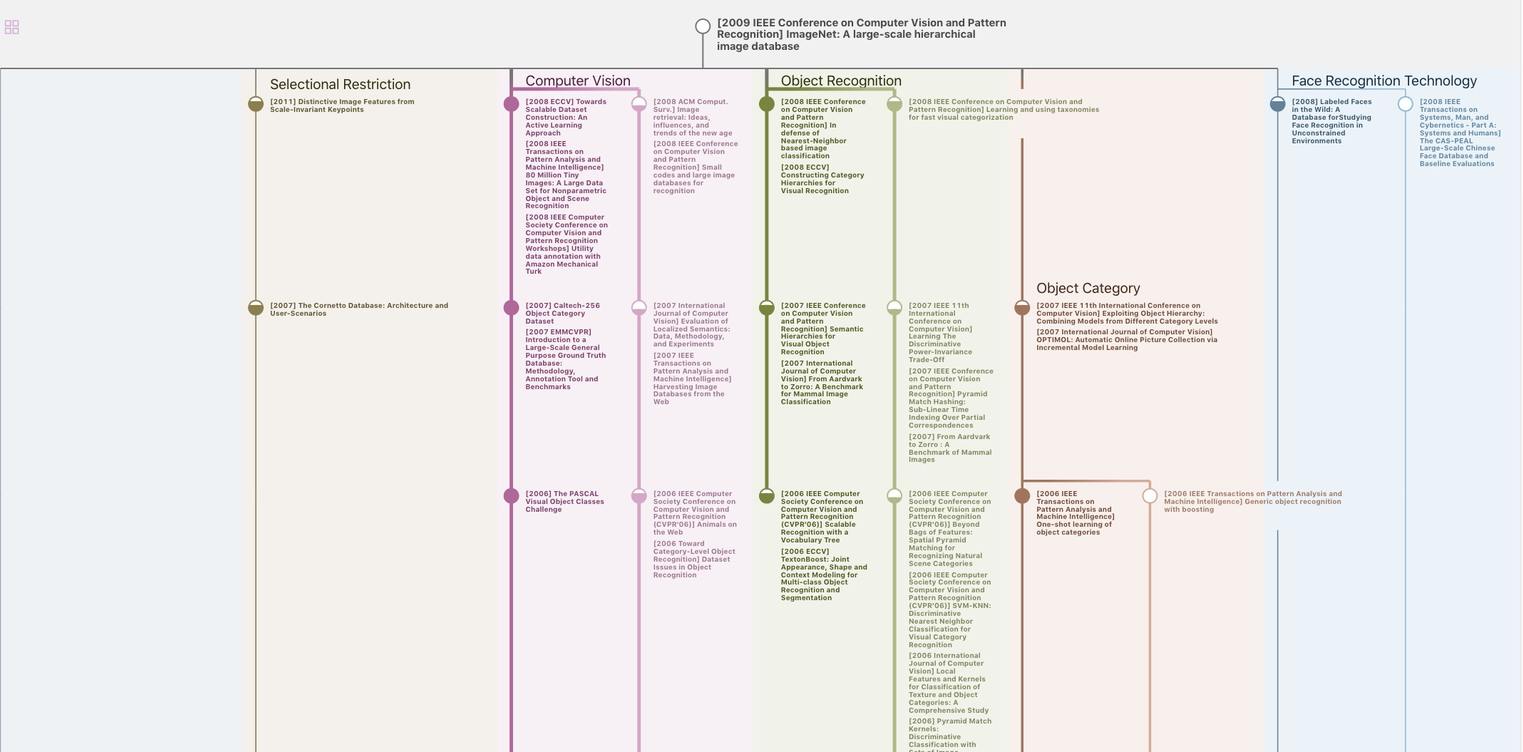
生成溯源树,研究论文发展脉络
Chat Paper
正在生成论文摘要