Adversarial Robustness Via Attention Transfer
PATTERN RECOGNITION LETTERS(2021)
摘要
Deep neural networks are known to be vulnerable to adversarial attacks. The empirical analysis in our study suggests that attacks tend to induce diverse network architectures to shift the attention to irrel-evant regions. Motivated by this observation, we propose a regularization technique which enforces the attentions to be well aligned via the knowledge transfer mechanism, thereby encouraging the robustness. Resultant model exhibits unprecedented robustness, securing 63.81 % adversarial accuracy where the prior art is 51.59 % on CIFAR-10 dataset under PGD attacks. In addition, we go beyond performance to analyti-cally investigate the proposed method as an effective def ense. Significantly flattened loss landscape can be observed, demonstrating the promise of the proposed method for improving robustness and thus the deployment in security-sensitive settings.(c) 2021 Elsevier B.V. All rights reserved.
更多查看译文
关键词
Adversarial defense, Robustness, Representation learning, Visual attention, Transfer learning
AI 理解论文
溯源树
样例
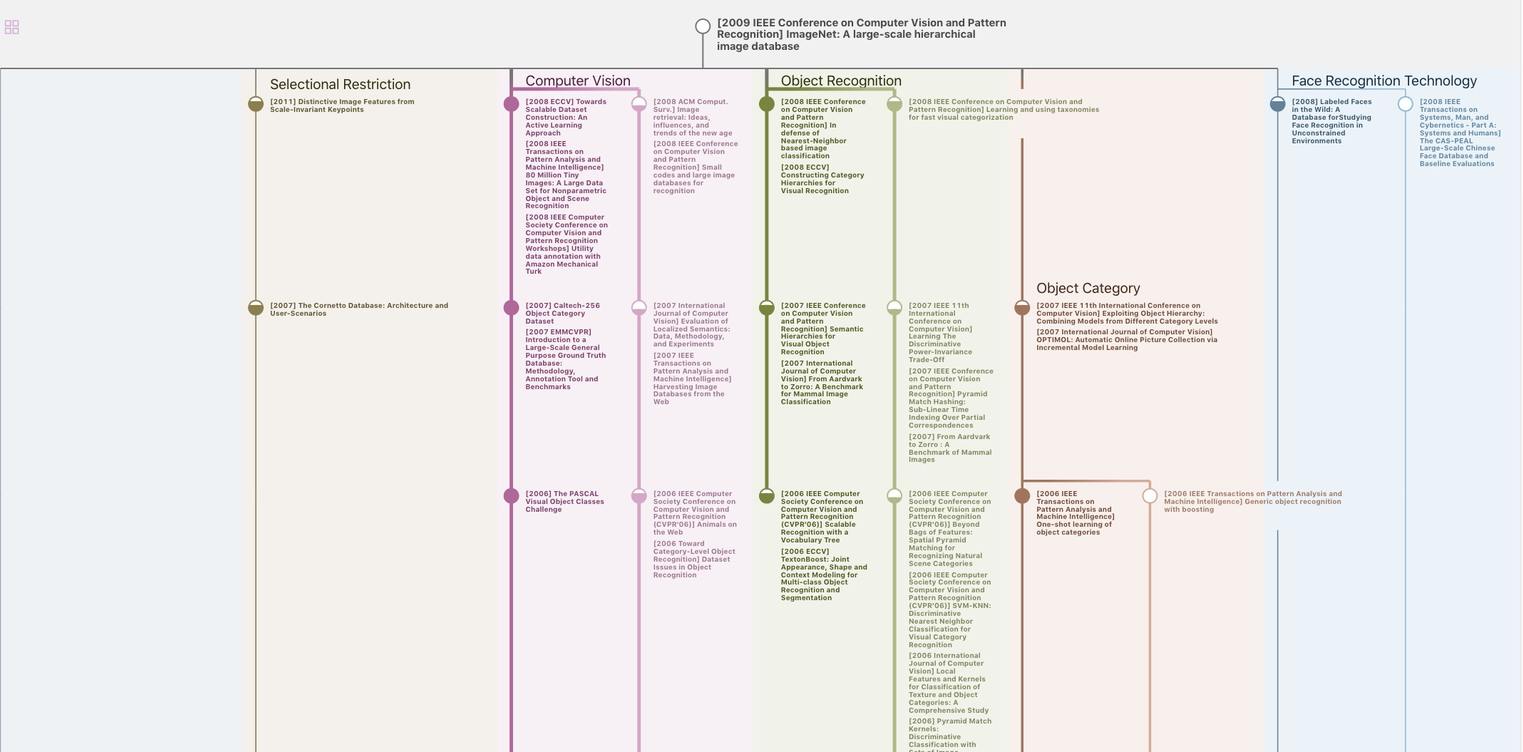
生成溯源树,研究论文发展脉络
Chat Paper
正在生成论文摘要