Online Generalized Eigenvectors Extraction Via A Fixed-Point Approach
IEEE TRANSACTIONS ON SIGNAL PROCESSING(2021)
Abstract
Generalized principal component analysis (GPCA) has been an active area of research in statistical signal processing for decades. It is used, e.g., for denoising in subspace tracking as the noise of different nature is incorporated into the procedure of maximizing signal-to-noise ratio (SNR). This paper presents a fixed-point approach concerning the principal generalized eigenvector extraction. It is based on the basis iteration for maximizing the generalized Rayleigh quotient (GRQ) with a given matrix pencil. The proposed approach extracts multiple generalized eigenvectors of a matrix pencil by exploiting the orthogonal complement structure of its estimation. It has no requirement to choose the commonly used step size. This enhances its practical applicability, as selecting an appropriate step size is a bottleneck for most gradient flow based algorithms. Our approach is more suitable for online processing because of its easy implementation and low computational complexity. To show the efficacy, efficiency and practical applicability of the proposed algorithm, we conduct several experiments, two of which concern smart antenna and blind source separation applications. Our simulation results show that the proposed algorithm outperforms several existing algorithms in terms of convergence speed as well as computational time.
MoreTranslated text
Key words
Signal processing algorithms, Eigenvalues and eigenfunctions, Principal component analysis, Convergence, Signal to noise ratio, Mathematical model, Discrete cosine transforms, Generalized eigenvalue problem, fixed-point approach, generalized Rayleigh quotient, generalized subspace estimation
AI Read Science
Must-Reading Tree
Example
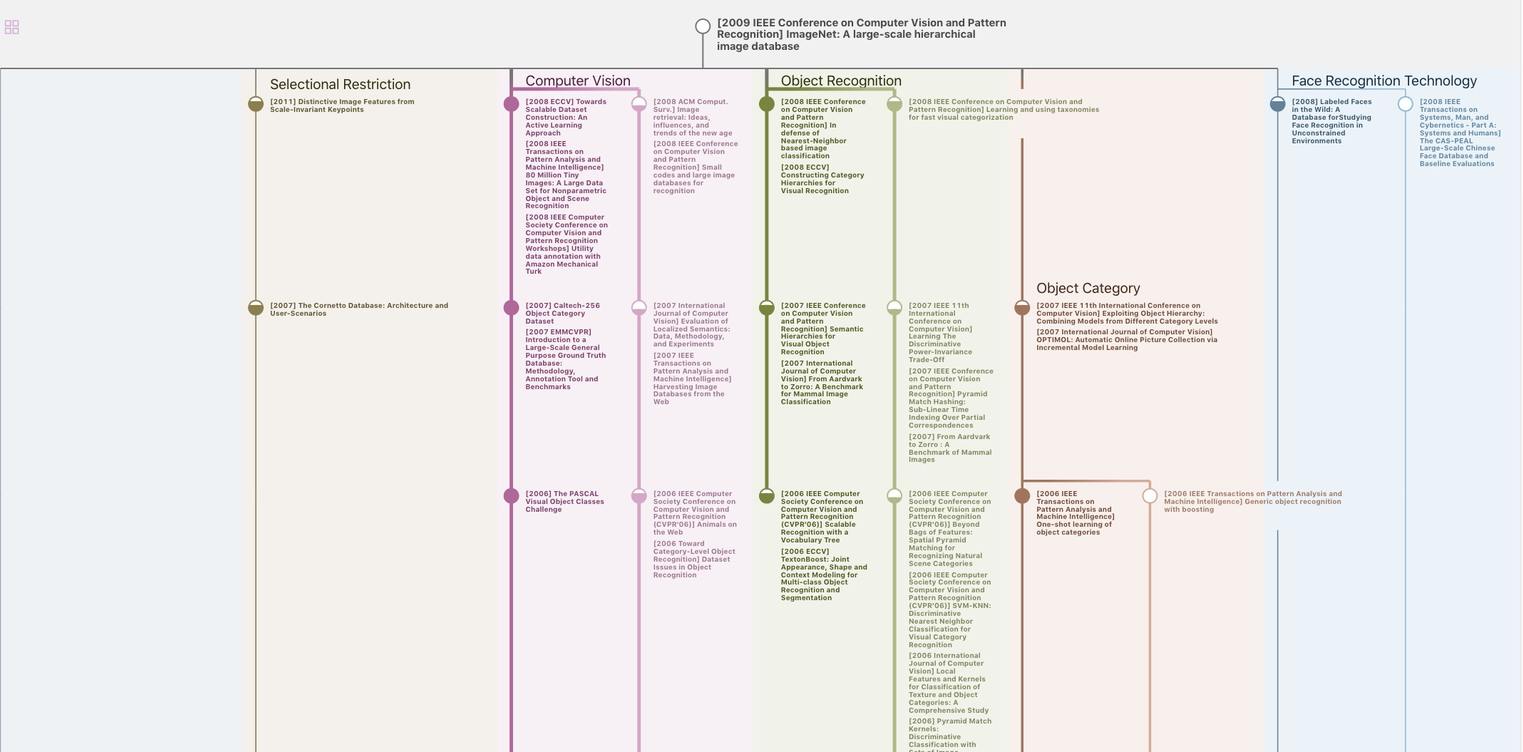
Generate MRT to find the research sequence of this paper
Chat Paper
Summary is being generated by the instructions you defined