Single Shot Multibox Detector With Deconvolutional Region Magnification Procedure
IEEE ACCESS(2021)
Abstract
In this paper, we make an effort to improve the accuracy of small and medium object detections of SSD (Single Shot Multibox Detector). To this end, we introduce a deconvolutional region magnification procedure in which the existing layers in SSD play a role in the region proposal network and the proposed regions are magnified for recognition. Moreover, features are also extracted from a shallow layer and a new feature pyramid is constructed on top of these structures. Then, features are contacted and fed into classification and regression modules as in SSD. The weights of the present model are obtained via a pre-training-re-training strategy. By evaluating the model performance on a test set assembled by the samples in the PASCAL VOC and MS COCO datasets, the present model shows that the mAPs (mean average precisions) of small and medium object detections are 42.4% and 74.7% respectively, which are 27.1% and 15.6% better than SSD. This proves the effectiveness of our proposed method.
MoreTranslated text
Key words
Feature extraction, Object detection, Proposals, Detectors, Deconvolution, Semantics, Image color analysis, Deep learning, object detection, SSD, deconvolution
AI Read Science
Must-Reading Tree
Example
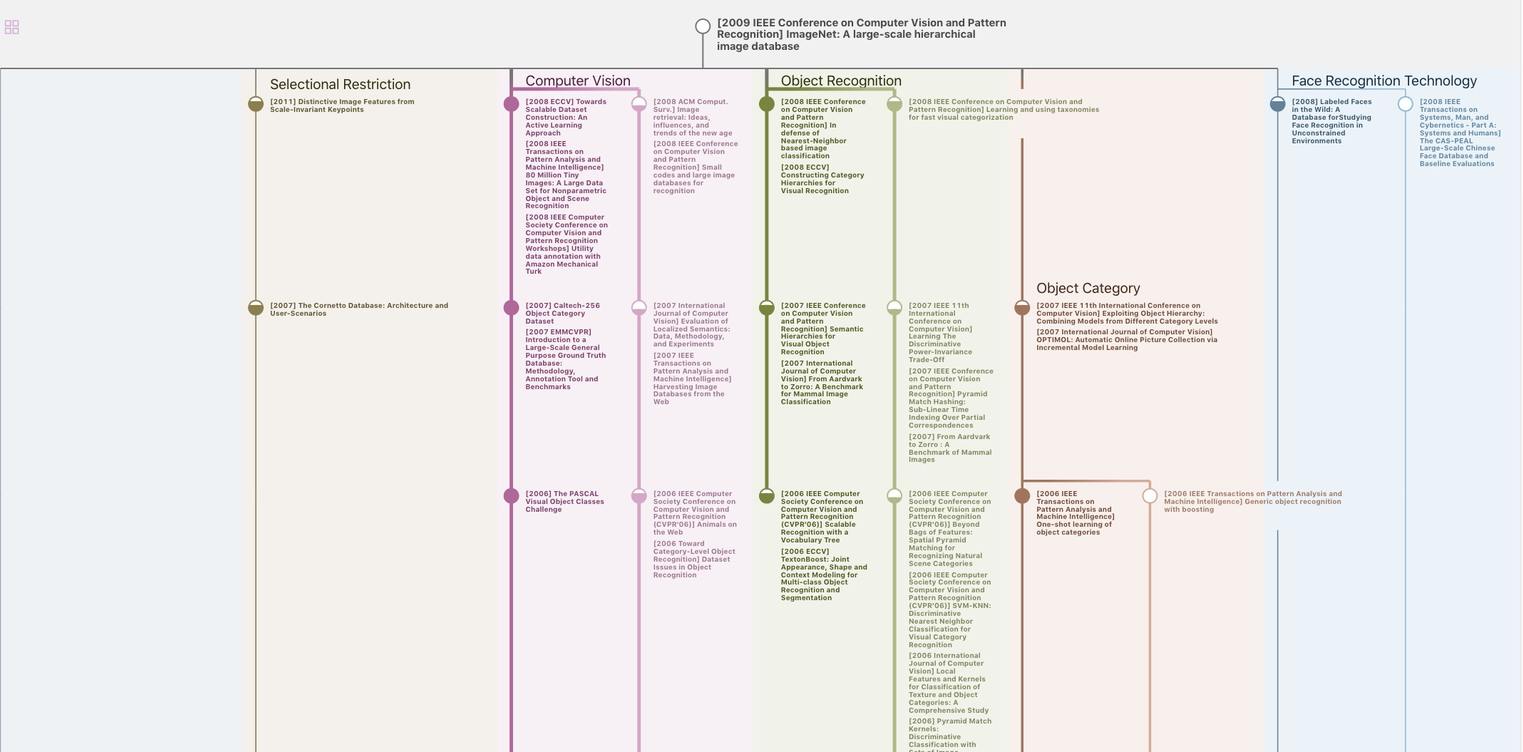
Generate MRT to find the research sequence of this paper
Chat Paper
Summary is being generated by the instructions you defined