A Bayesian assessment of tumour prevalence in brown bullhead and white sucker from the Canadian waters of the Great Lakes
Journal of Great Lakes Research(2021)
摘要
Liver and skin tumours in brown bullhead (Ameiurus nebulosus) and white sucker (Catostomus commersoni) have been associated with contamination of the aquatic environment. In this study, we present a Bayesian hierarchical logistic-Bernoulli framework that considers the role of fish covariates (e.g., age, total weight, gonad weight, liver weight, fork length) on tumour prevalence to identify the presence of “hot-spots” around the Canadian waters of the Great Lakes, where high fish tumour frequencies are registered. We developed methods to discern the degree of impairment that are either based on the comparison of tumour frequencies in contaminated (or impacted) sites against those predicted in their corresponding reference areas, or the assessment of the prevailing conditions in impaired sites independently, without the need to establish baseline conditions for comparison purposes. Our modelling study predicts low frequencies of neoplastic tumours in all the impacted locations. In contrast, the same comparisons with the preneoplastic lesions provided evidence of distinct differences between impacted and reference sites in Jackfish Bay, St. Mary’s River, Niagara River, Hamilton Harbour, and Bay of Quinte. We also found weak to moderately strong relationships between tumour occurrence and fish physical characteristics that varied considerably in terms of their strength and nature (sign) among the different locations. Our study concludes that the prevalence of neoplastic tumours appears to have reached acceptable levels around the Great Lakes, but the distinctly higher levels of preneoplasms in several impacted locations underscore the need to improve our understanding of the lesions that may lead to carcinogenesis.
更多查看译文
关键词
Fish tumours,Bayesian inference,Delisting criteria,Great lakes,Areas of concern
AI 理解论文
溯源树
样例
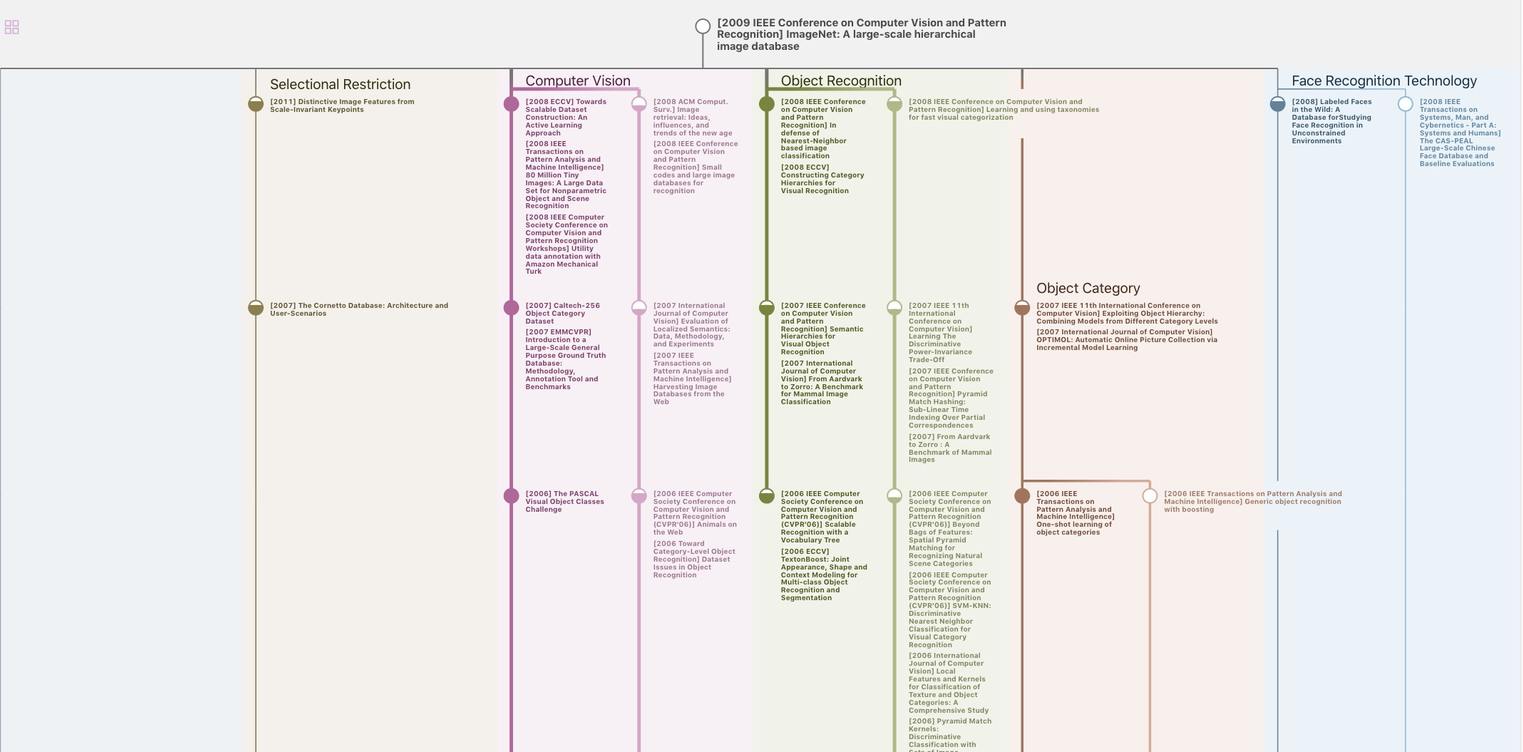
生成溯源树,研究论文发展脉络
Chat Paper
正在生成论文摘要