Statistical Downscaling Using Principal Component Regression For Climate Change Impact Assessment At The Cauvery River Basin
JOURNAL OF WATER AND CLIMATE CHANGE(2021)
摘要
Climate change impact studies are generally carried out with higher resolution general circulation model (GCM) outputs, which are usually for a global scale, and it is difficult to use the same for a regional scale. GCM simulations require downscaling to get a courser scale output for local climate impact studies. In this study, an improvised principal component regression (PCR) downscaling technique is adapted to downscale 26 Coupled Model Intercomparison Project Phase 5 (CMIP5) GCM historical outputs. A massive river basin named Cauvery with 35 observation stations is categorized into three subbasin to study the regional climate impacts. In this case, the PCR model performed remarkably well compared to other conventional machine learning models with half the computational time than usual. The test statistics state that the validation of the proposed model illustrates a variance in calibration results of the PCR model, which is ranged between 2 and 5%, and a variance in validation, which is less than 7% throughout the study area. Since it is desired to prioritize GCMs to choose the merely suitable models for a strategic climate study, the models were selected based on the PCR model performance. Furthermore, CCSM4, inmcm4, and EC-EARTH model's performance in recreating precipitation statistics over the study area are exceptional.
更多查看译文
关键词
climate change, performance evaluation, statistical downscaling
AI 理解论文
溯源树
样例
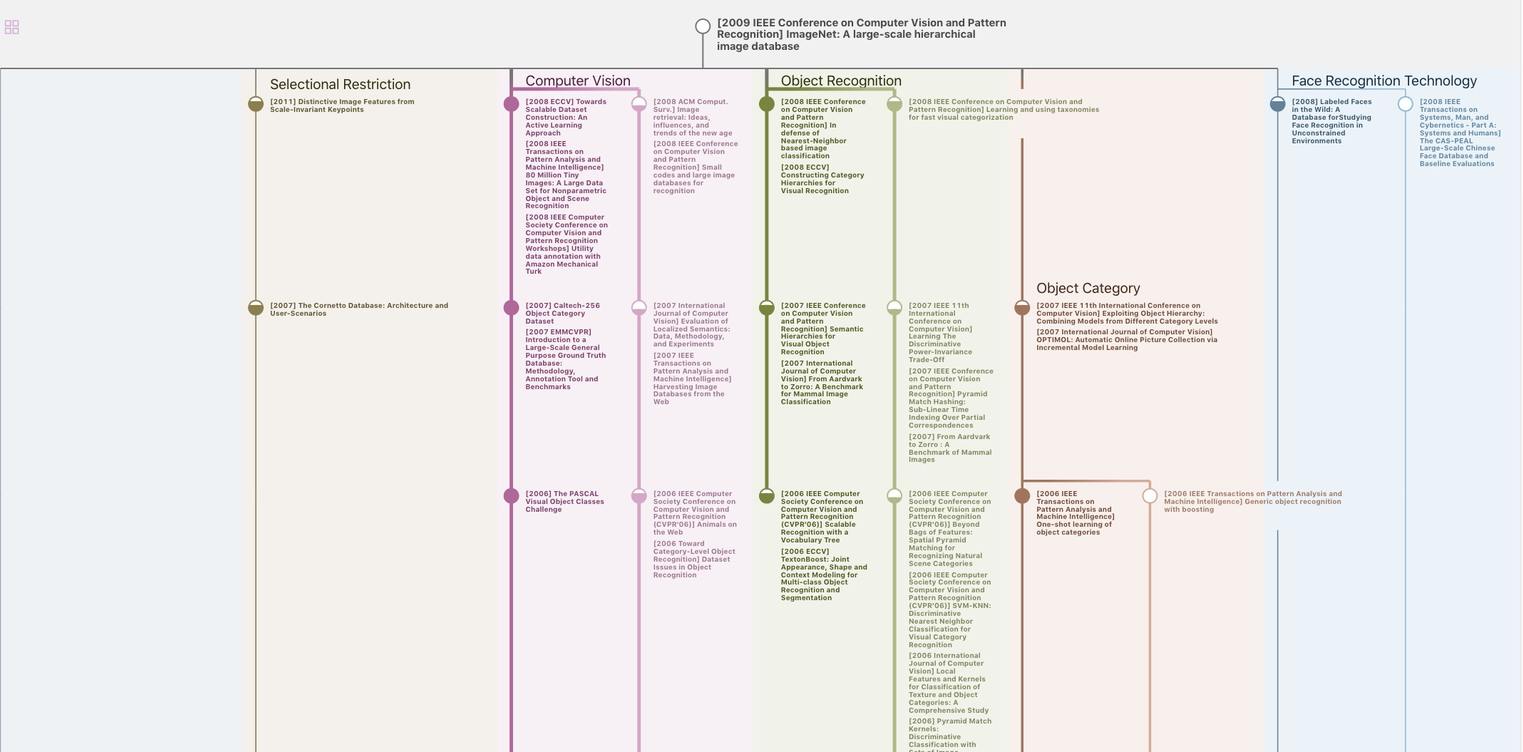
生成溯源树,研究论文发展脉络
Chat Paper
正在生成论文摘要