Forecasting Charging Demand of Electric Vehicles Using Time-Series Models
ENERGIES(2021)
摘要
This study compared the methods used to forecast increases in power consumption caused by the rising popularity of electric vehicles (EVs). An excellent model for each region was proposed using multiple scaled geographical datasets over two years. EV charging volumes are influenced by various factors, including the condition of a vehicle, the battery's state-of-charge (SOC), and the distance to the destination. However, power suppliers cannot easily access this information due to privacy issues. Despite a lack of individual information, this study compared various modeling techniques, including trigonometric exponential smoothing state space (i.e., Trigonometric, Box-Cox, Auto-Regressive-Moving-Average (ARMA), Trend, and Seasonality (TBATS)), autoregressive integrated moving average (ARIMA), artificial neural networks (ANN), and long short-term memory (LSTM) modeling, based on past values and exogenous variables. The effect of exogenous variables was evaluated in macro- and micro-scale geographical areas, and the importance of historic data was verified. The basic statistics regarding the number of charging stations and the volume of charging in each region are expected to aid the formulation of a method that can be used by power suppliers.
更多查看译文
关键词
electric vehicle,charging demand,charging stations,TBATS,ARIMA,ANN,LSTM
AI 理解论文
溯源树
样例
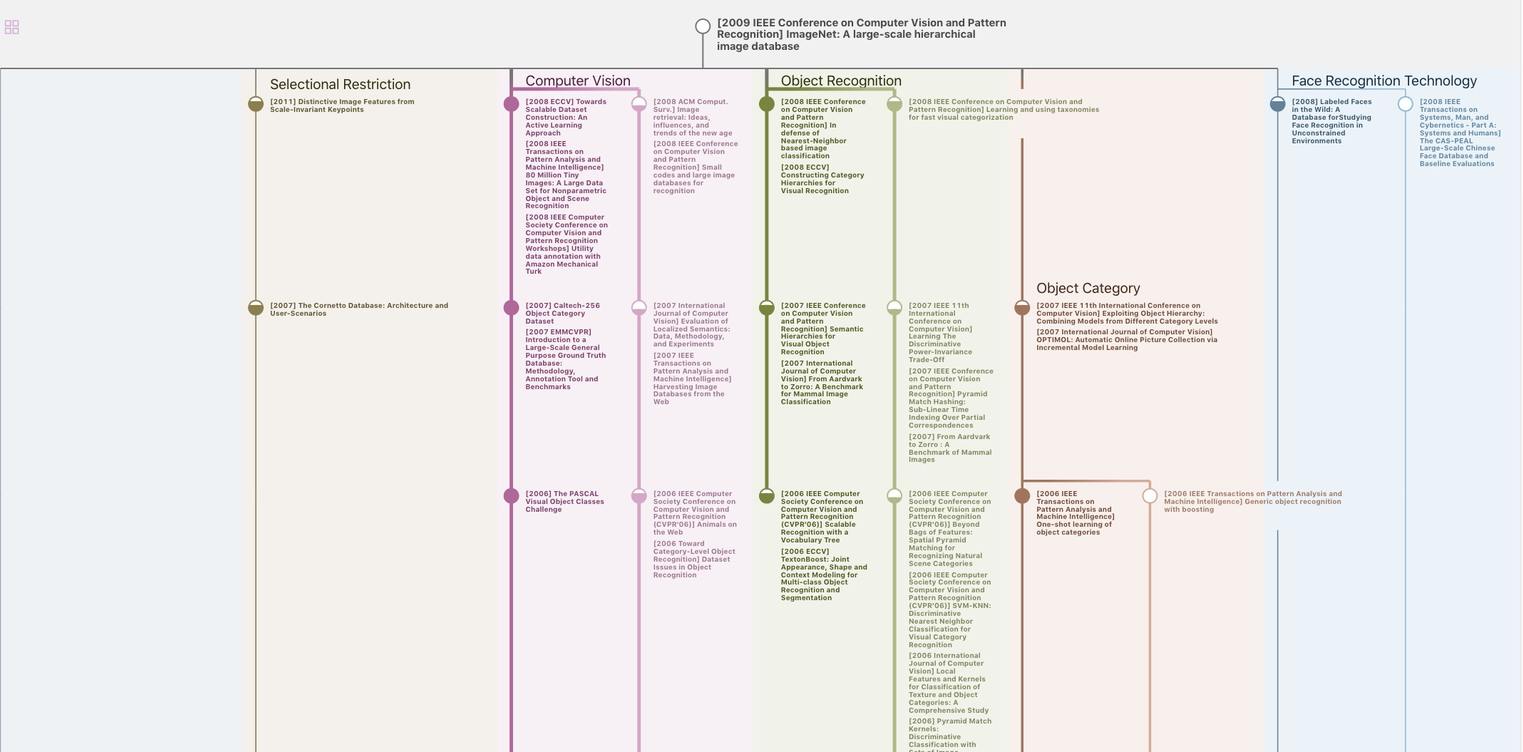
生成溯源树,研究论文发展脉络
Chat Paper
正在生成论文摘要