Price elasticity estimation for deep learning-based choice models: an application to air itinerary choices
JOURNAL OF REVENUE AND PRICING MANAGEMENT(2021)
摘要
One of the most popular approaches to model choices in the airline industry is the multinomial logit (MNL) model and its variations because it has key properties for businesses: acceptable accuracy and high interpretability. On the other hand, recent research has proven the interest of considering choice models based on deep neural networks as these provide better out-of-sample predictive power. However, these models typically lack direct business interpretability. One useful way to get insights for consumer behavior is by estimating and studying the price elasticity in different choice situations. In this research, we present a new methodology to estimate price elasticity from Deep Learning-based choice models. The approach leverages the automatic differentiation capabilities of deep learning libraries. We test our approach on data extracted from a global distribution system (GDS) on European market data. The results show clear differences in price elasticity between leisure and business trips. Overall, the demand for trips is price elastic for leisure and inelastic for the business segment. Moreover, the approach is flexible enough to study elasticity on different dimensions, showing that the demand for business trips could become highly elastic in some contexts like departures during weekends, international destinations, or when the reservation is done with enough anticipation. All these insights are of a particular interest for travel providers (e.g., airlines) to better adapt their offer, not only to the segment but also to the context.
更多查看译文
关键词
Price elasticity,Discrete choice modeling,Deep learning,Interpretability,Automatic differentiation,Travel industry
AI 理解论文
溯源树
样例
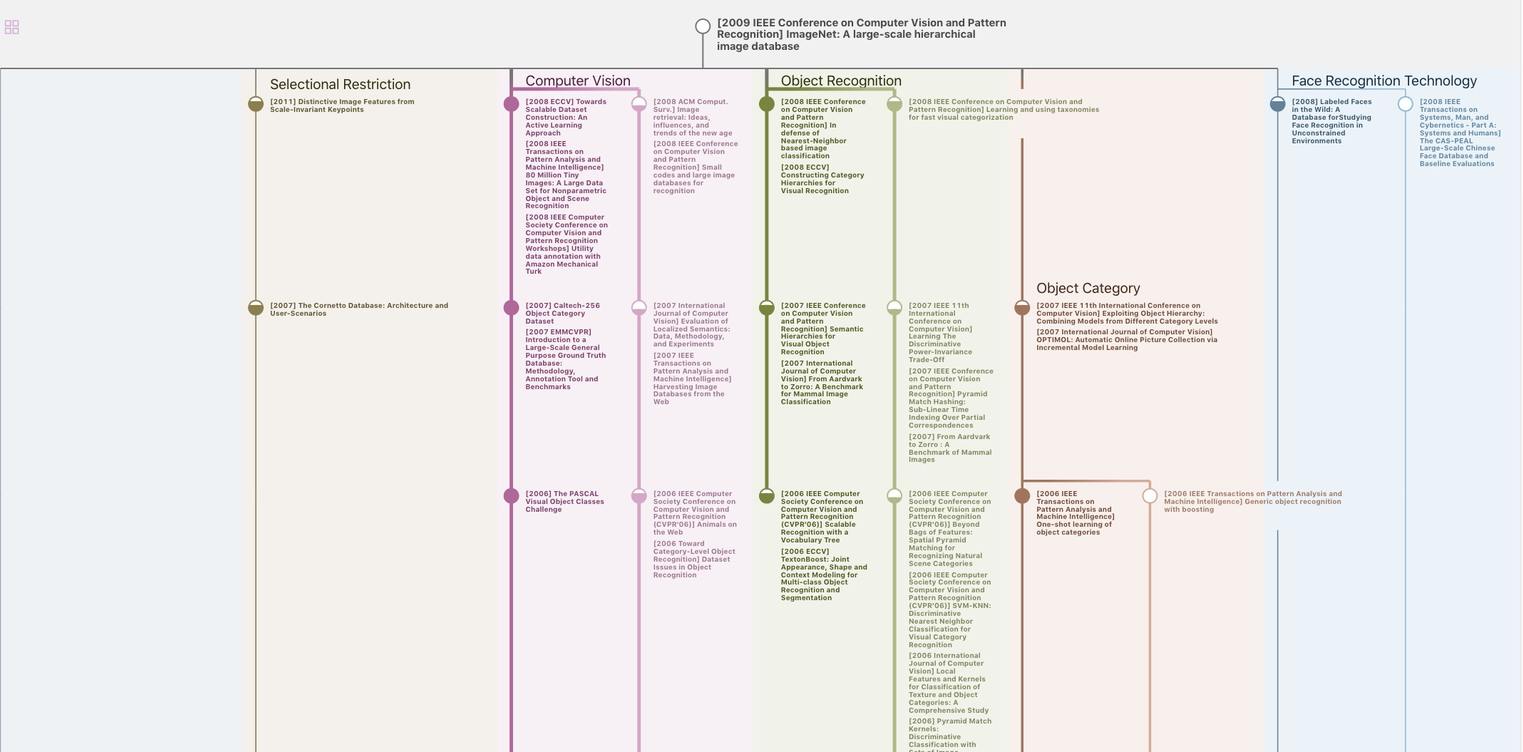
生成溯源树,研究论文发展脉络
Chat Paper
正在生成论文摘要