Location Selection for Air Quality Monitoring With Consideration of Limited Budget and Estimation Error
IEEE Transactions on Mobile Computing(2022)
摘要
Accurate acquisition of air quality is important for improving human well-being. However, directly monitoring air quality at all locations is costly. The challenge is how we can select a small number of locations to monitor the air quality such that the estimation error of air quality at other locations can be minimized. In this paper, a general location selection strategy is proposed based on active learning, which involves iterations of a selector and an estimator. We implement four instances of this general strategy to embody it: KAL (Active Learning based on Kriging), TAL (Active Learning based on Regression Tree), KMAL (Active Learning based on Kriging and MPGR) and TMAL (Active Learning based on Regression Tree and MPGR). The estimator of KAL or TAL can estimate the air quality at remaining locations from air quality samples at monitoring locations, leveraging spatial or cross-domain correlation of air quality. The selecting indicators of their selectors are designed to measure the uncertainty of unlabeled samples according to their estimators. KMAL and TMAL are upgrades of the former two, respectively, by introducing MPGR (Manifold Preserving Graph Reduction) to also take the representativeness of unlabeled samples into account. The experimental results show that the proposed strategy can achieve a low estimation error with few monitoring locations. Particularly, given the same budget (i.e., the number of monitoring locations), the estimation error is reduced from about 20 percent of baselines to 15 percent by KAL and to 5 percent by KMAL; and TAML likewise.
更多查看译文
关键词
Air quality monitoring,estimation error,active learning,manifold preserving graph reduction
AI 理解论文
溯源树
样例
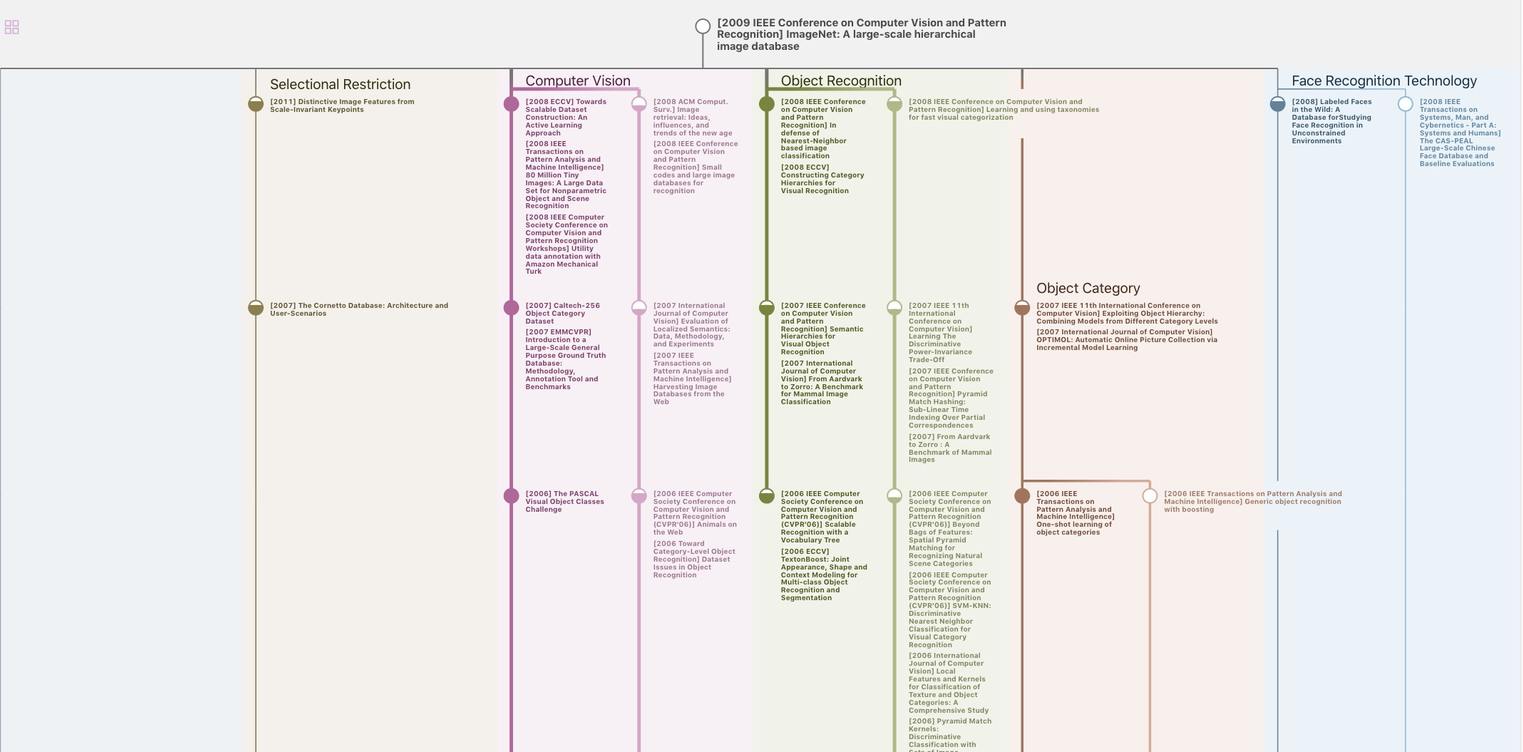
生成溯源树,研究论文发展脉络
Chat Paper
正在生成论文摘要