Multi-view data clustering via non-negative matrix factorization with manifold regularization
INTERNATIONAL JOURNAL OF MACHINE LEARNING AND CYBERNETICS(2021)
摘要
Nowadays, non-negative matrix factorization (NMF) based cluster analysis for multi-view data shows impressive behavior in machine learning. Usually, multi-view data have complementary information from various views. The main concern behind the NMF is how to factorize the data to achieve a significant clustering solution from these complementary views. However, NMF does not focus to conserve the geometrical structures of the data space. In this article, we intensify on the above issue and evolve a new NMF clustering method with manifold regularization for multi-view data. The manifold regularization factor is exploited to retain the locally geometrical structure of the data space and gives extensively common clustering solution from multiple views. The weight control term is adopted to handle the distribution of each view weight. An iterative optimization strategy depended on multiplicative update rule is applied on the objective function to achieve optimization. Experimental analysis on the real-world datasets are exhibited that the proposed approach achieves better clustering performance than some state-of-the-art algorithms.
更多查看译文
关键词
Non-negative matrix factorization, Multi-view clustering, Manifold regularization, Weighted view
AI 理解论文
溯源树
样例
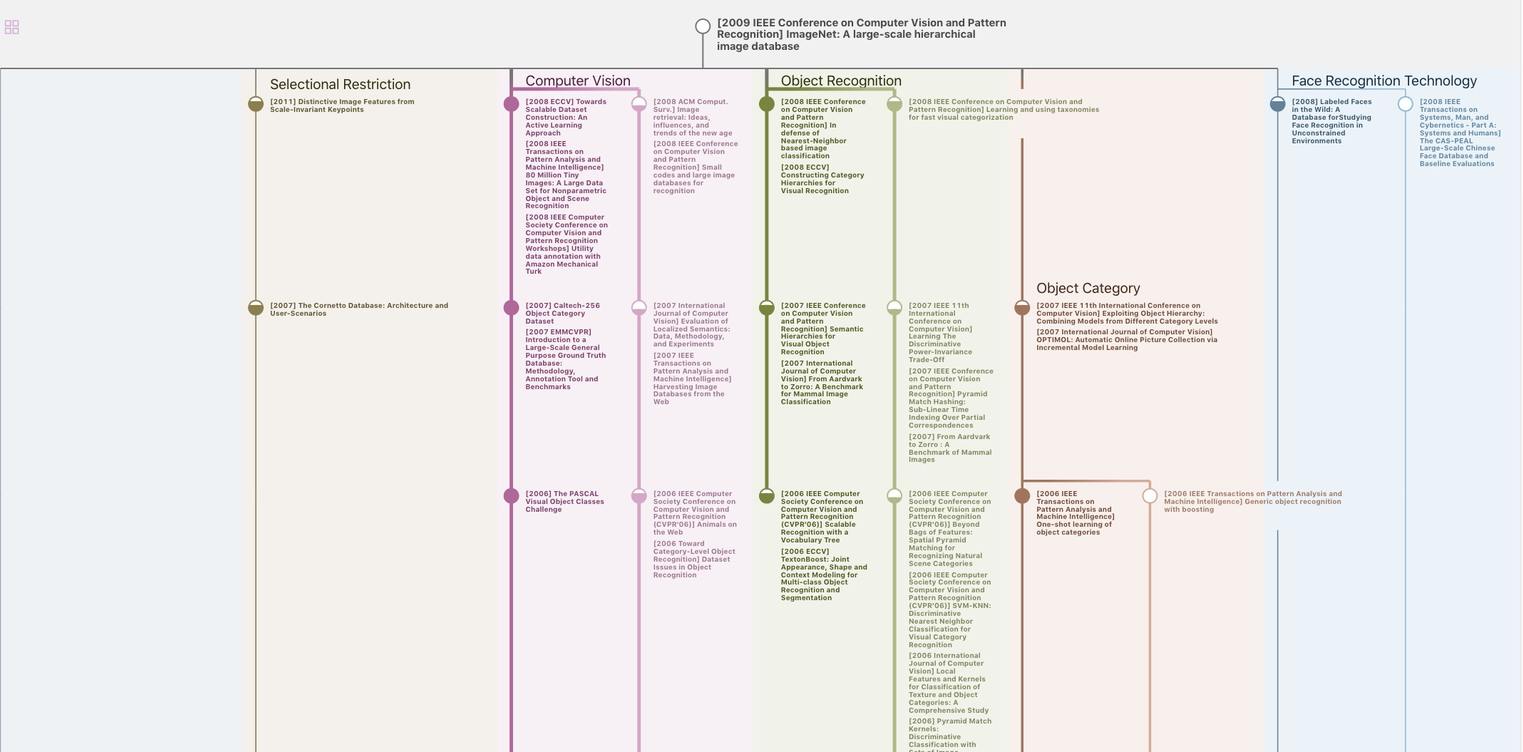
生成溯源树,研究论文发展脉络
Chat Paper
正在生成论文摘要