S-THAD: a framework for sensor-based temporal human activity detection from continuous data streams
JOURNAL OF AMBIENT INTELLIGENCE AND HUMANIZED COMPUTING(2021)
摘要
With recent evolvement in smart sensing systems, sensor-based activity recognition has endured numerous research studies that mainly emphasize classifying pre-segmented data chunks having a fixed duration. Each data chunk generally involves a single human activity for classification into one of the predefined activity classes. Such activity recognition models, trained with pre-segmented and fixed-size data chunks, cannot adapt well to natural human activities in real-time settings, where the duration of activities varies. Also, the real-time data available from smart devices is in a continuous form and not discrete chunks. As a result, the real-time implementation of activity-aware applications based on the existing models becomes impractical. Therefore, in this paper, a novel framework, i.e., “S-THAD”, is proposed for sensor-based temporal human activity detection from the continuous and untrimmed 3D motion sensor data. The proposed method is capable of detecting when and which activity of interest appears in a continuous data stream. The publicly available PAMAP2 dataset is used to test the proposed scheme, which entails long and untrimmed data streams from the wearable inertial sensors placed at three different body positions, including hand, chest, and ankle. The experimental results indicate that the proposed scheme achieves significant detection performance on this dataset.
更多查看译文
关键词
Activity recognition, Continuous data stream, Machine learning, Proposal classification, Temporal activity detection, Wearable sensor
AI 理解论文
溯源树
样例
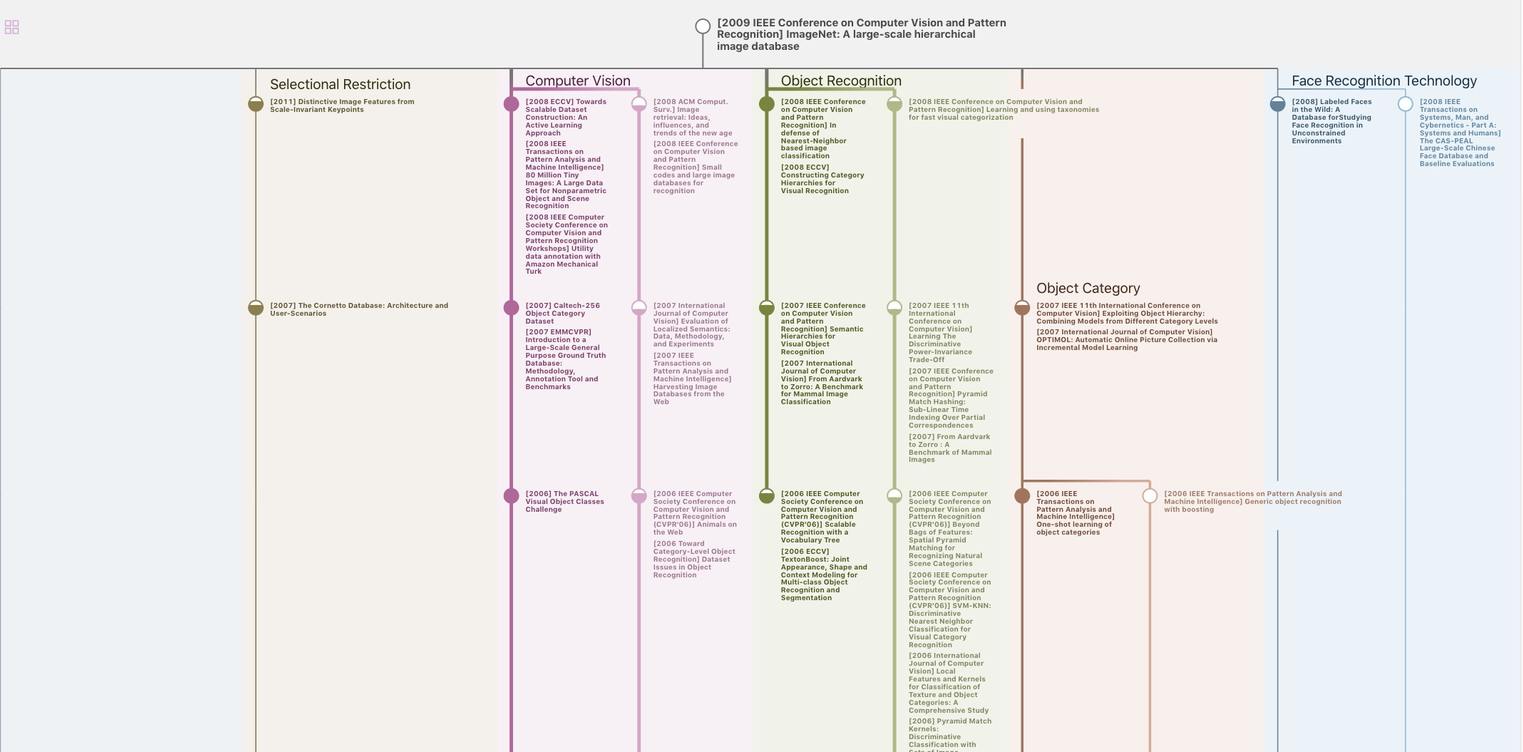
生成溯源树,研究论文发展脉络
Chat Paper
正在生成论文摘要