A symmetrization of the Subspace Gaussian Mixture Model.
ICASSP(2011)
Abstract
Last year we introduced the Subspace Gaussian Mixture Model (SGMM), and we demonstrated Word Error Rate improvements on a fairly small-scale task. Here we describe an extension to the SGMM, which we call the symmetric SGMM. It makes the model fully symmetric between the "speech-state vectors" and "speaker vectors" by making the mixture weights depend on the speaker as well as the speech state. We had previously avoided this as it introduces difficulties for efficient likelihood evaluation and parameter estimation, but we have found a way to overcome those difficulties. We find that the symmetric SGMM can give a very worthwhile improvement over the previously described model. We will also describe some larger-scale experiments with the SGMM, and report on progress toward releasing open-source software that supports SGMMs.
MoreTranslated text
Key words
Gaussian processes,parameter estimation,speech recognition,vectors,SGMM,likelihood evaluation,parameter estimation,speaker vector,speech recognition,speech-state vector,subspace Gaussian mixture model,word error rate improvement,Hidden Markov Models,Speech Recognition,Subspace Gaussian Mixture Models
AI Read Science
Must-Reading Tree
Example
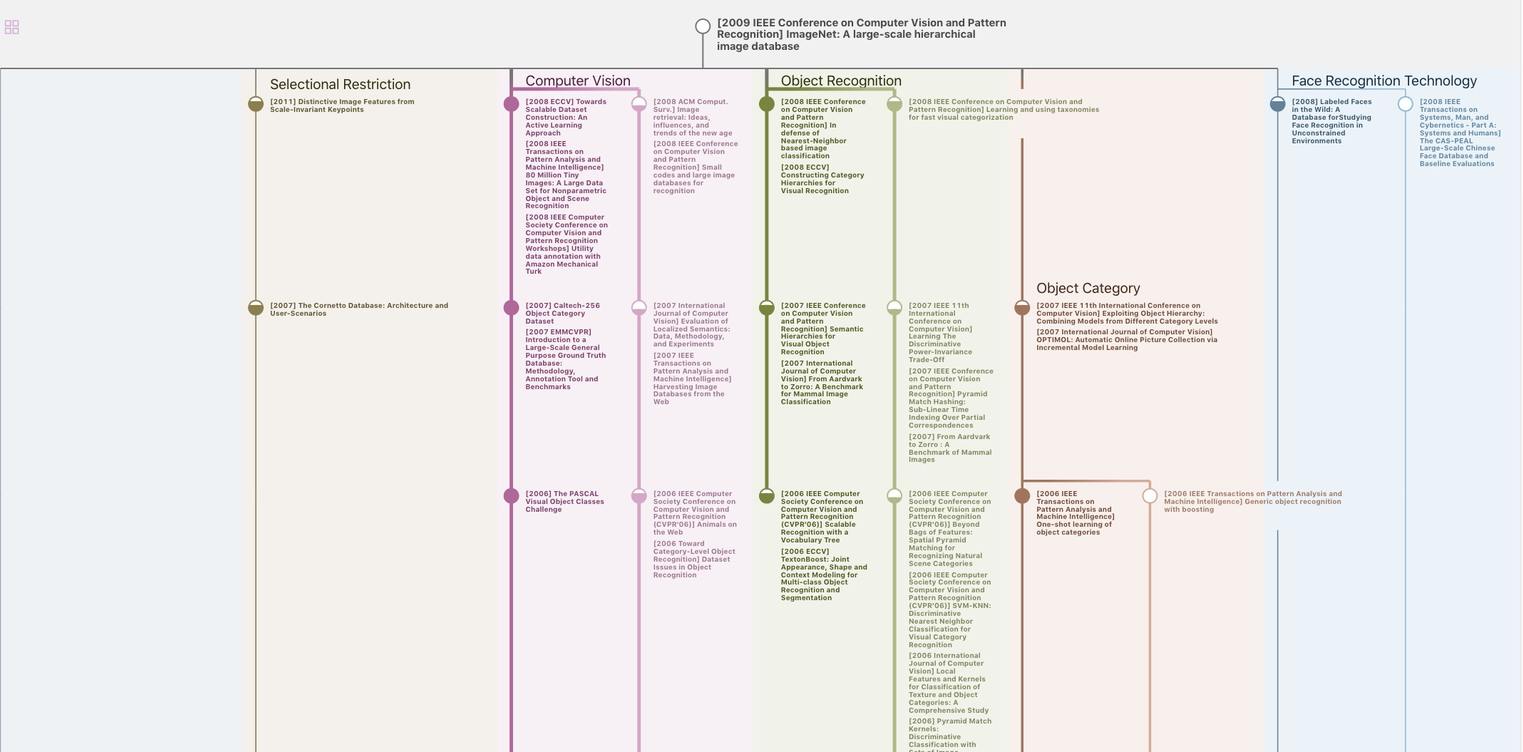
Generate MRT to find the research sequence of this paper
Chat Paper
Summary is being generated by the instructions you defined