Learning Device-invariant And Location-invariant Embedding For Speaker Verification Using Adversarial Multi-task Training
2020 International Conference on Internet of Things and Intelligent Applications (ITIA)(2020)
摘要
Deep speaker embedding model has achieved the satisfactory performance in close-talking speaker verification. However, in the real home environment, the device used to record voice may be different and the distance between speaker and device is constantly changing. These will make performance degradation. In this paper, a novel approach of adversarial multitask training is proposed to solve the problem of device mismatch and location variation by learning device-invariant and location-invariant embeddings. A gradient reversal layer and a device-and-location classifier are added to the speaker validation model in order to build an auxiliary adversarial task. Experiments are conducted on the far-field text-dependent speaker verification database called HI-MIA, the proposed approach achieves 3.38% Equal Error Rate (EER) in close-talking enrollment task and achieves 3.36% EER in far-field enrollment task.
更多查看译文
关键词
speaker verification,deep speaker embedding,adversarial multi-task training
AI 理解论文
溯源树
样例
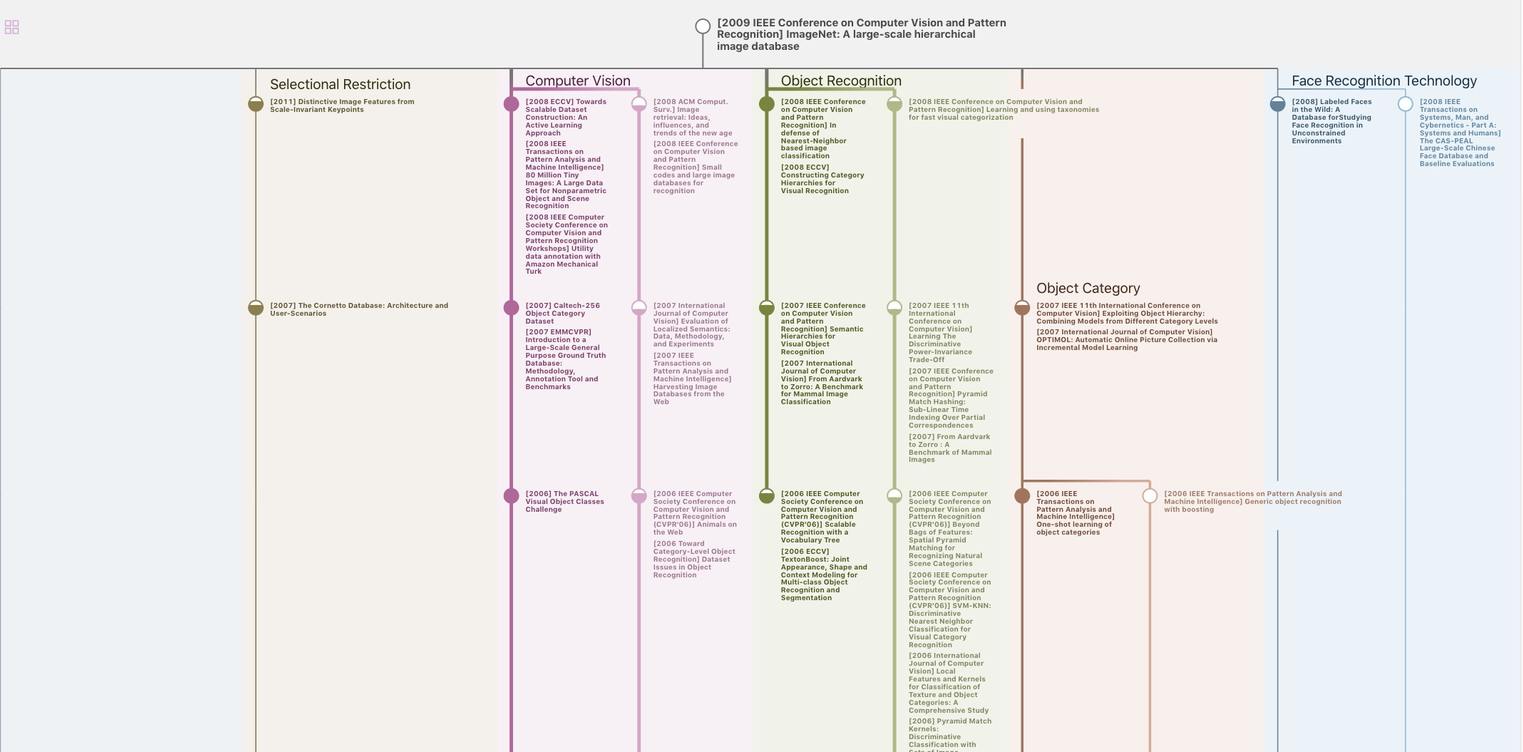
生成溯源树,研究论文发展脉络
Chat Paper
正在生成论文摘要