Revisiting Convolutional Neural Networks for Citywide Crowd Flow Analytics
MACHINE LEARNING AND KNOWLEDGE DISCOVERY IN DATABASES, ECML PKDD 2020, PT I(2021)
摘要
Citywide crowd flow analytics is of great importance to smart city efforts. It aims to model the crowd flow (e.g., inflow and outflow) of each region in a city based on historical observations. Nowadays, Convolutional Neural Networks (CNNs) have been widely adopted in rasterbased crowd flow analytics by virtue of their capability in capturing spatial dependencies. After revisiting CNN-based methods for different analytics tasks, we expose two common critical drawbacks in the existing uses: 1) inefficiency in learning global spatial dependencies, and 2) overlooking latent region functions. To tackle these challenges, in this paper we present a novel framework entitled DeepLGR that can be easily generalized to address various citywide crowd flow analytics problems. This framework consists of three parts: 1) a local feature extraction module to learn representations for each region; 2) a global context module to extract global contextual priors and upsample them to generate the global features; and 3) a region-specific predictor based on tensor decomposition to provide customized predictions for each region, which is very parameter-efficient compared to previous methods. Extensive experiments on two typical crowd flow analytics tasks demonstrate the effectiveness, stability, and generality of our framework.
更多查看译文
关键词
citywide crowd flow
AI 理解论文
溯源树
样例
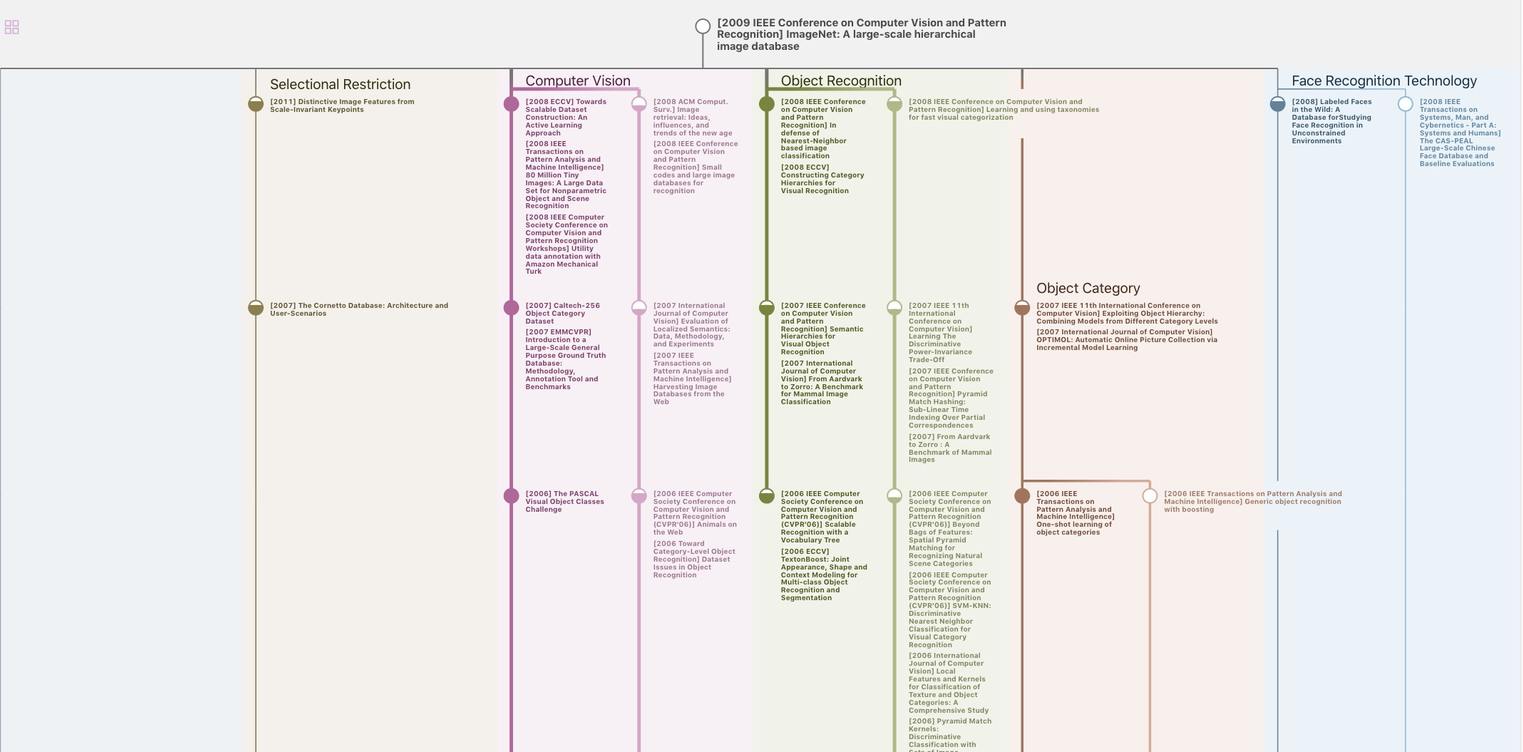
生成溯源树,研究论文发展脉络
Chat Paper
正在生成论文摘要