p Joint Estimation and Robustness Optimization
MANAGEMENT SCIENCE(2022)
摘要
Many real-world optimization problems have input parameters estimated from data whose inherent imprecision can lead to fragile solutions that may impede desired objectives and/or render constraints infeasible. We propose a joint estimation and robustness optimization (JERO) framework to mitigate estimation uncertainty in optimization problems by seamlessly incorporating both the parameter estimation procedure and the optimization problem. Toward that end, we construct an uncertainty set that incorporates all of the data, and the size of the uncertainty set is based on how well the parameters are estimated from that data when using a particular estimation procedure: regressions, the least absolute shrinkage and selection operator, and maximum likelihood estimation (among others). The JERO model maximizes the uncertainty set's size and so obtains solutions that-unlike those derived from models dedicated strictly to robust optimization- are immune to parameter perturbations that would violate constraints or lead to objective function values exceeding their desired levels. We describe several applications and provide explicit formulations of the JERO framework for a variety of estimation procedures. To solve the JERO models with exponential cones, we develop a second-order conic approximation that limits errors beyond an operating range; with this approach, we can use state-of-the-art second-order conic programming solvers to solve even large-scale convex optimization problems.
更多查看译文
关键词
robustness optimization, robust optimization, parameter estimation, -driven optimization
AI 理解论文
溯源树
样例
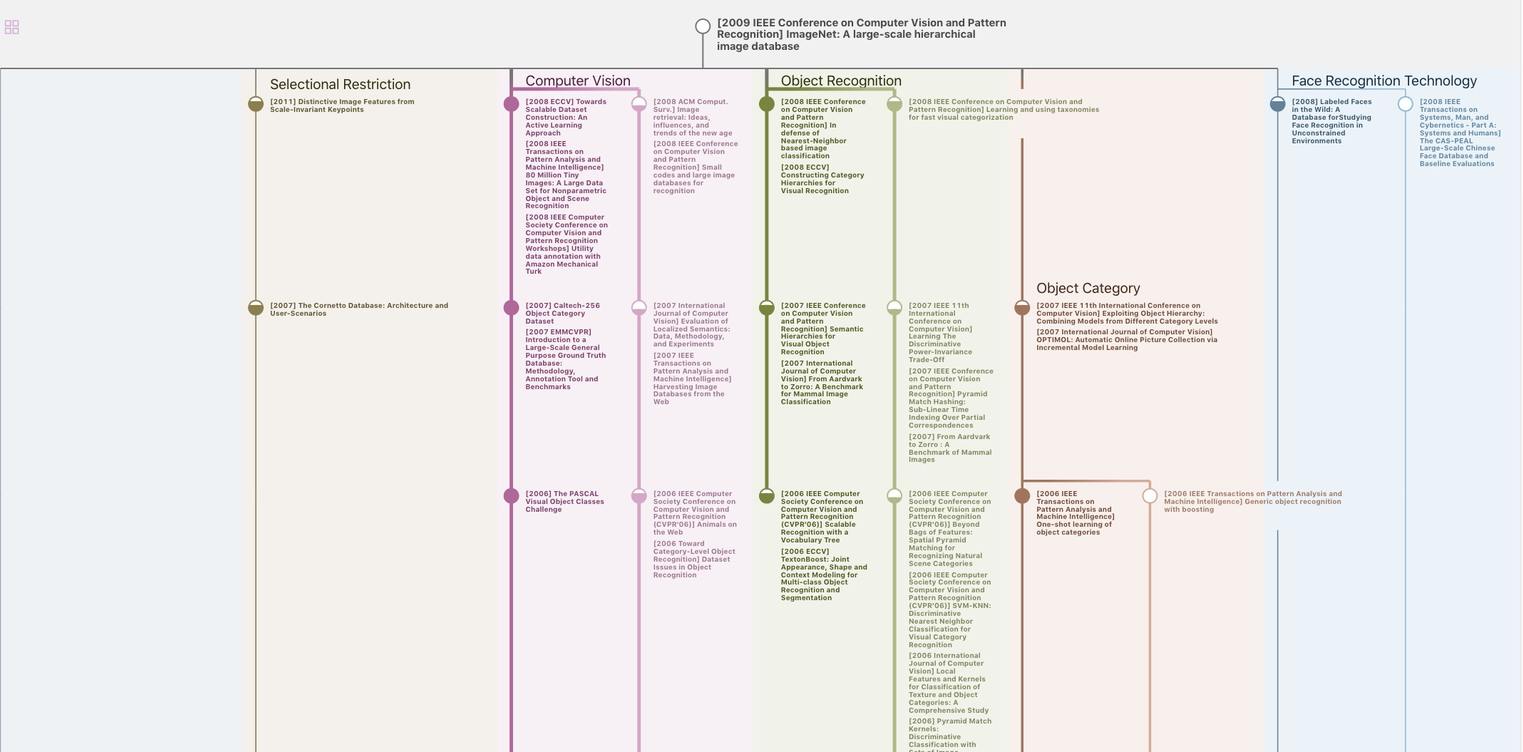
生成溯源树,研究论文发展脉络
Chat Paper
正在生成论文摘要