Ensemble Methods for Heart Disease Prediction
NEW GENERATION COMPUTING(2021)
摘要
Heart disease prediction is a critical task regarding human health. It is based on deriving an Machine Learning model from medical parameters to predict risk levels. In this work, we propose and test novel ensemble methods for heart disease prediction. Randomness analysis of distance sequences is utilized to derive a classifier, which is served as a base estimator of a bagging scheme. Method is successfully tested on medical Spectf dataset. Additionally, a Graph Lasso and Ledoit–Wolf shrinkage-based classifier is developed for Statlog dataset which is a UCI data. These two algorithms yield comparatively good accuracy results: 88.7 and 88.8 for Spectf and Statlog, respectively. These proposed algorithms provide promising results and novel classification methods that can be utilized in various domains to improve performance of ensemble methods.
更多查看译文
关键词
Randomness test,Ensemble methods,Heart disease prediction,Covariance estimator,Mahalanobis distance,Bagging classifier,Weak classifier
AI 理解论文
溯源树
样例
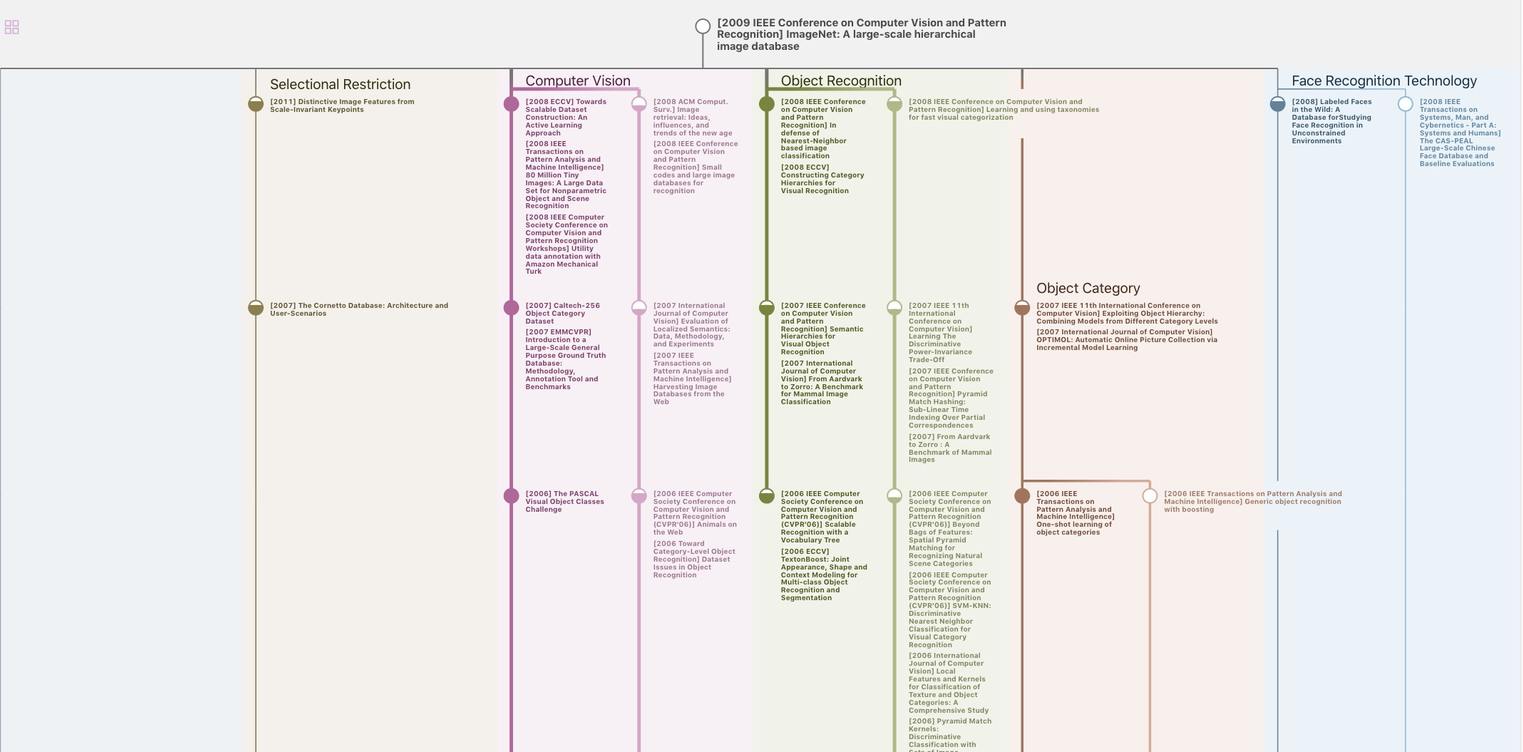
生成溯源树,研究论文发展脉络
Chat Paper
正在生成论文摘要