A method to predict heterogeneous glutenite reservoir permeability from nuclear magnetic resonance (NMR) logging
ARABIAN JOURNAL OF GEOSCIENCES(2021)
摘要
Permeability is an important input parameter in evaluating formation validity and predicting deliverability; its value is always estimated from porosity or other formation parameters based on the statistical methods. However, glutenite reservoir permeability prediction faces a great challenge due to the strong heterogeneity. In this study, to establish a reasonable model to predict heterogeneous glutenite reservoir permeability, 483 core samples were recovered from the Permian Jiamuhe Formation, Shawan Depression, northwestern Junggar Basin, China for routine physical property experiments; 21 of them were chosen to apply for laboratory nuclear magnetic resonance (NMR) measurements. After introducing the hydraulic flow unit (HFU) theory, these 483 core samples are classified into eight categories based on the flow zone indicator (FZI) difference, and applicative relationships of core-derived porosity and permeability are respectively established for every type of core sample. Meanwhile, based on the deeply analyzing of the expression of FZI and the classic Timur-Coates permeability-based model, a theoretical model of calculating FZI from NMR data is proposed. The involved input parameters are calibrated by using the experimental data of 21 core samples. After the established models are extended into field applications, the consecutive FZI curve is estimated from NMR logging to classify formations, and the corresponding relationships are used to predict permeability. Comparisons of the predicted FZI and permeability with core-derived results verify the reliability of the proposed models. They can be well used to precisely predict permeability in our target heterogeneous glutenite reservoirs.
更多查看译文
关键词
Permeability prediction, Glutenite reservoir, Nuclear magnetic resonance (NMR) data, Flow zone indicator (FZI), Timur-Coates permeability-based model
AI 理解论文
溯源树
样例
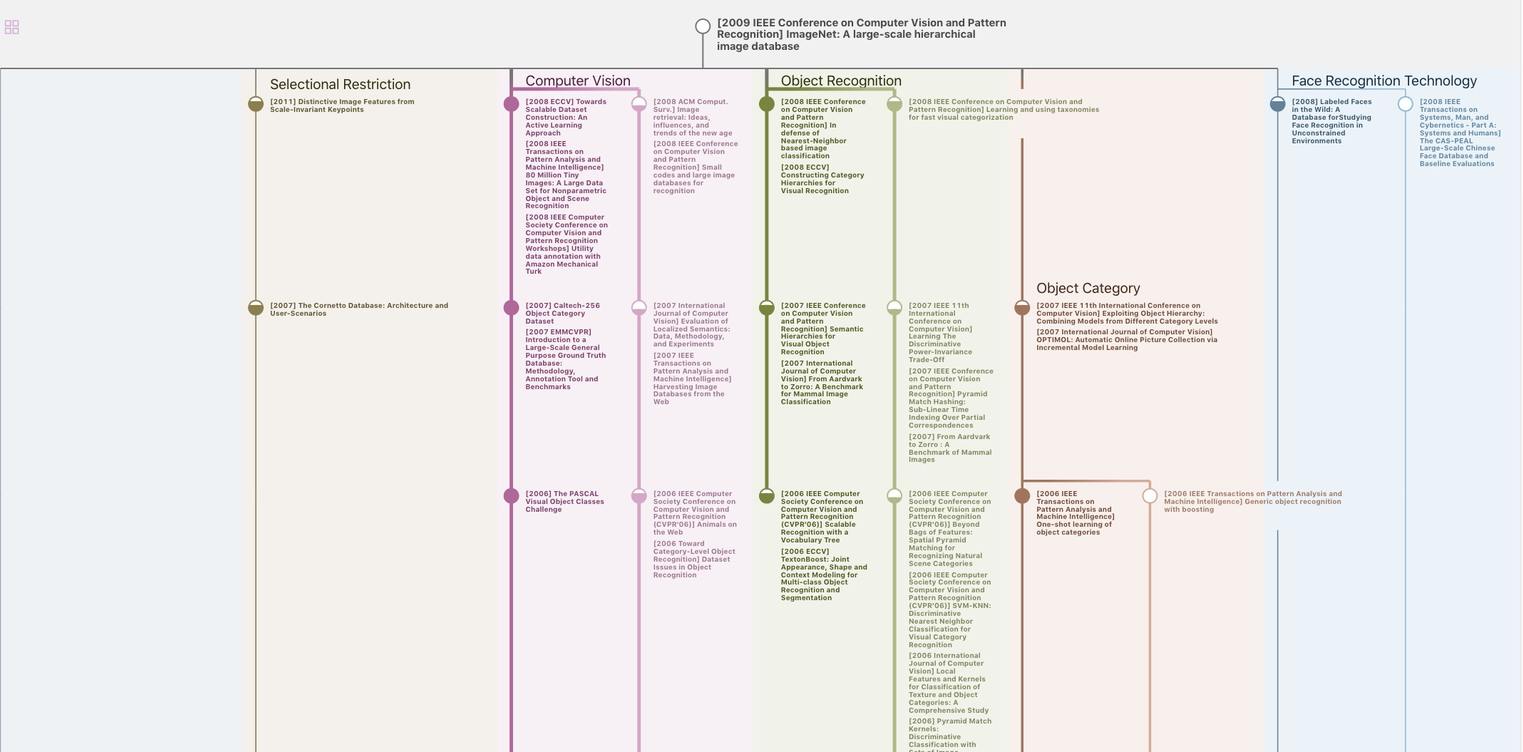
生成溯源树,研究论文发展脉络
Chat Paper
正在生成论文摘要