Hyperspectral Image Clustering: Current achievements and future lines
IEEE Geoscience and Remote Sensing Magazine(2021)
摘要
Hyperspectral remote sensing organically combines traditional space imaging with advanced spectral measurement technologies, delivering advantages stemming from continuous spectrum data and rich spatial information. This development of hyperspectral technology takes remote sensing into a brand-new phase, making the technology widely applicable in various fields. Hyperspectral clustering analysis is widely utilized in hyperspectral image (HSI) interpretation and information extraction, which can reveal the natural partition pattern of pixels in an unsupervised way. In this article, current hyperspectral clustering algorithms are systematically reviewed and summarized in nine main categories: centroid-based, density-based, probability-based, bionics-based, intelligent computing-based, graph-based, subspace clustering, deep learning-based, and hybrid mechanism-based. The performance of several popular hyperspectral clustering methods is demonstrated on two widely used data sets. HSI clustering challenges and possible future research lines are identified.
更多查看译文
关键词
Hyperspectral imaging, Clustering methods, Clustering algorithms, Data models, Computational modeling, Biological system modeling, Task analysis
AI 理解论文
溯源树
样例
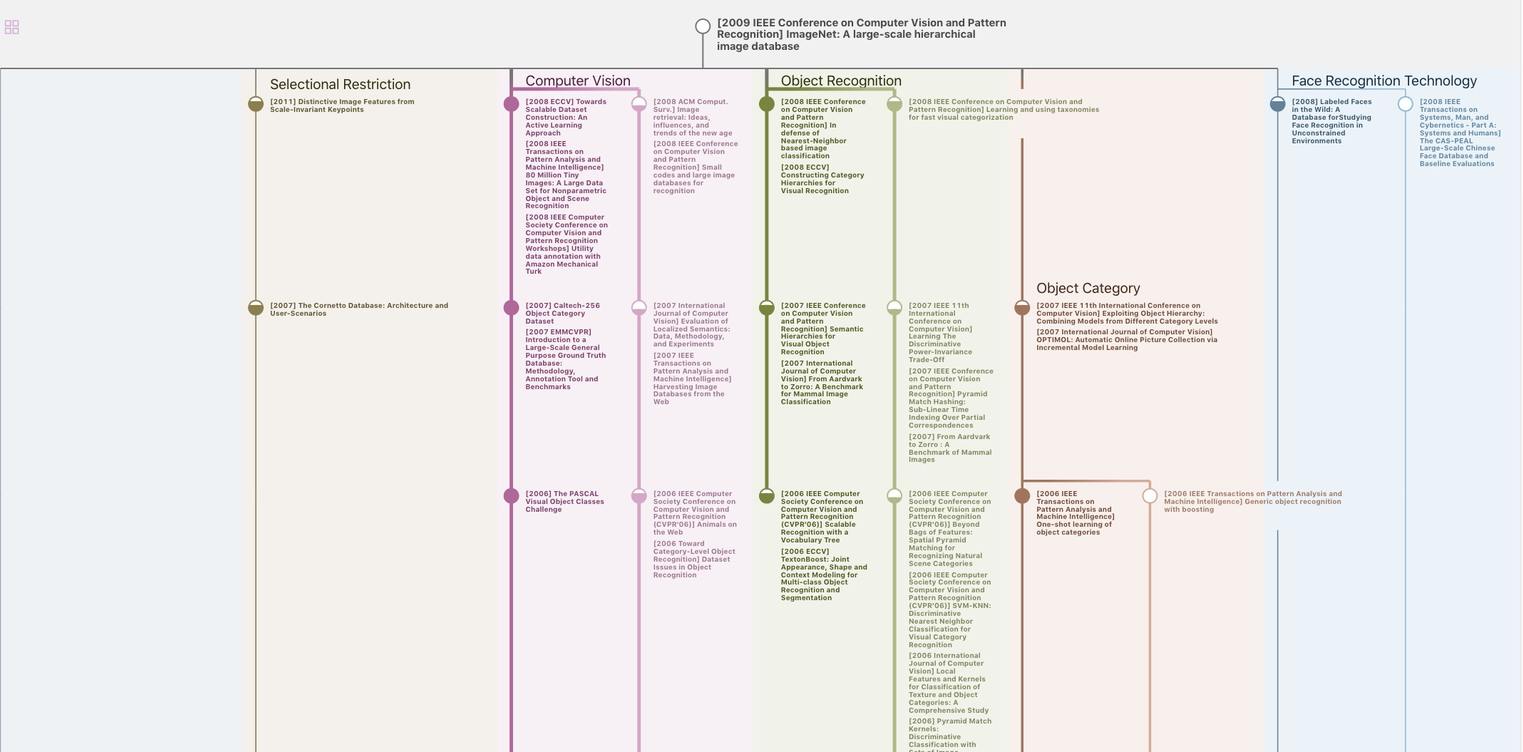
生成溯源树,研究论文发展脉络
Chat Paper
正在生成论文摘要