Single- and Multipoint Aerodynamic Shape Optimization Using Multifidelity Models and Manifold Mapping
JOURNAL OF AIRCRAFT(2021)
摘要
In this paper, a computationally efficient multifidelity local search algorithm for aerodynamic design optimization is presented. In this paper's approach, direct optimization of a computationally expensive model is replaced by an iterative updating and reoptimization of a fast multifidelity model constructed using a low-fidelity model adapted locally using manifold mapping (MM) to become a reliable representation of the high-fidelity one during the optimization process. Only one high-fidelity model evaluation is needed per design iteration, and no gradient information is used. The proposed method is validated and characterized by applying it to a few single- and multipoint optimization problems involving inviscid and viscous transonic flows. The proposed method is compared with the sequential least-squares programming (SLSQP) gradient-based approach with the gradients calculated based on adjoint sensitivities. In the inviscid single-point test case, a drag reduction of 410.8 counts was achieved by the MM algorithm while requiring approximately 1469 min on a high performance computing (HPC) with 32 processors. SLSQP with adjoints achieved a drag reduction of 425.9 counts, while requiring approximately 1536 min under the same HPC setup. For the viscous single-point test case, a 83.2 drag count reduction was reported for the MM compared to 83.4 for SLSQP with adjoints. In that case, the MM algorithm was around eight times faster in terms of computing time. In the multipoint design test case, the MM algorithm was computationally cheaper by at least an order of magnitude compared to SLSQP with adjoints, although the objective function value was around three drag counts higher. Furthermore, it was found that in the multipoint cases the MM algorithm scales favorably with the number of operational conditions considered compared to SLSQP with adjoints.
更多查看译文
AI 理解论文
溯源树
样例
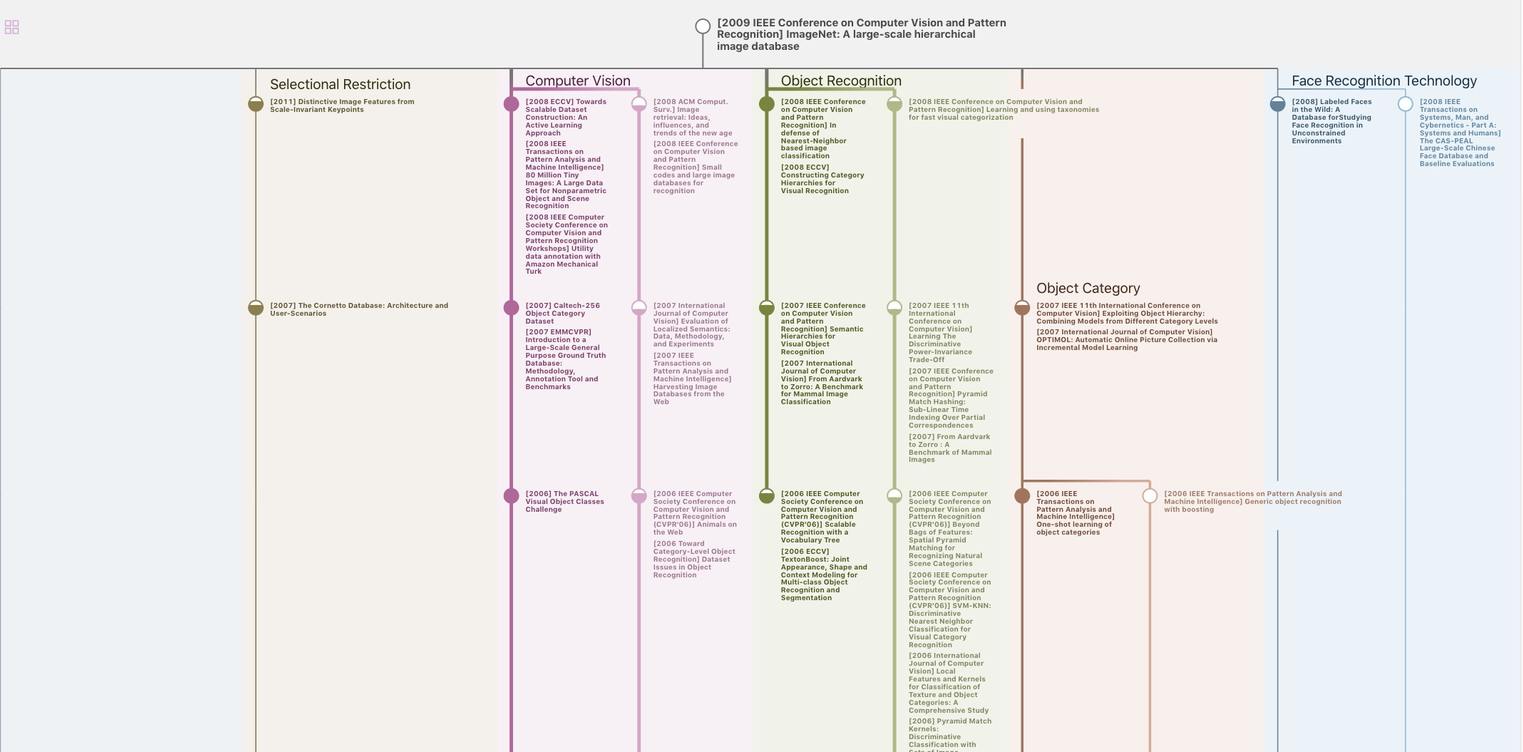
生成溯源树,研究论文发展脉络
Chat Paper
正在生成论文摘要