Surrogate Based Mutual Information Approximation And Optimization For Urban Source Localization
INTERNATIONAL JOURNAL FOR UNCERTAINTY QUANTIFICATION(2021)
摘要
The ability to efficiently and accurately localize potentially threatening nuclear radiation sources in urban environments is of critical importance to national security. Techniques to infer the location and intensity of a source using data from a configuration of radiation detectors, and the effectiveness of the source localization depends critically on how the detectors are configured. In this paper, we introduce a framework that uses surrogate models to efficiently compare and optimize different detector configurations. We compare our technique to others and demonstrate its effectiveness for selecting optimal detector configurations in the context of urban source localization.
更多查看译文
关键词
computational statistics, information theory, bayesian inference, spatial statistics
AI 理解论文
溯源树
样例
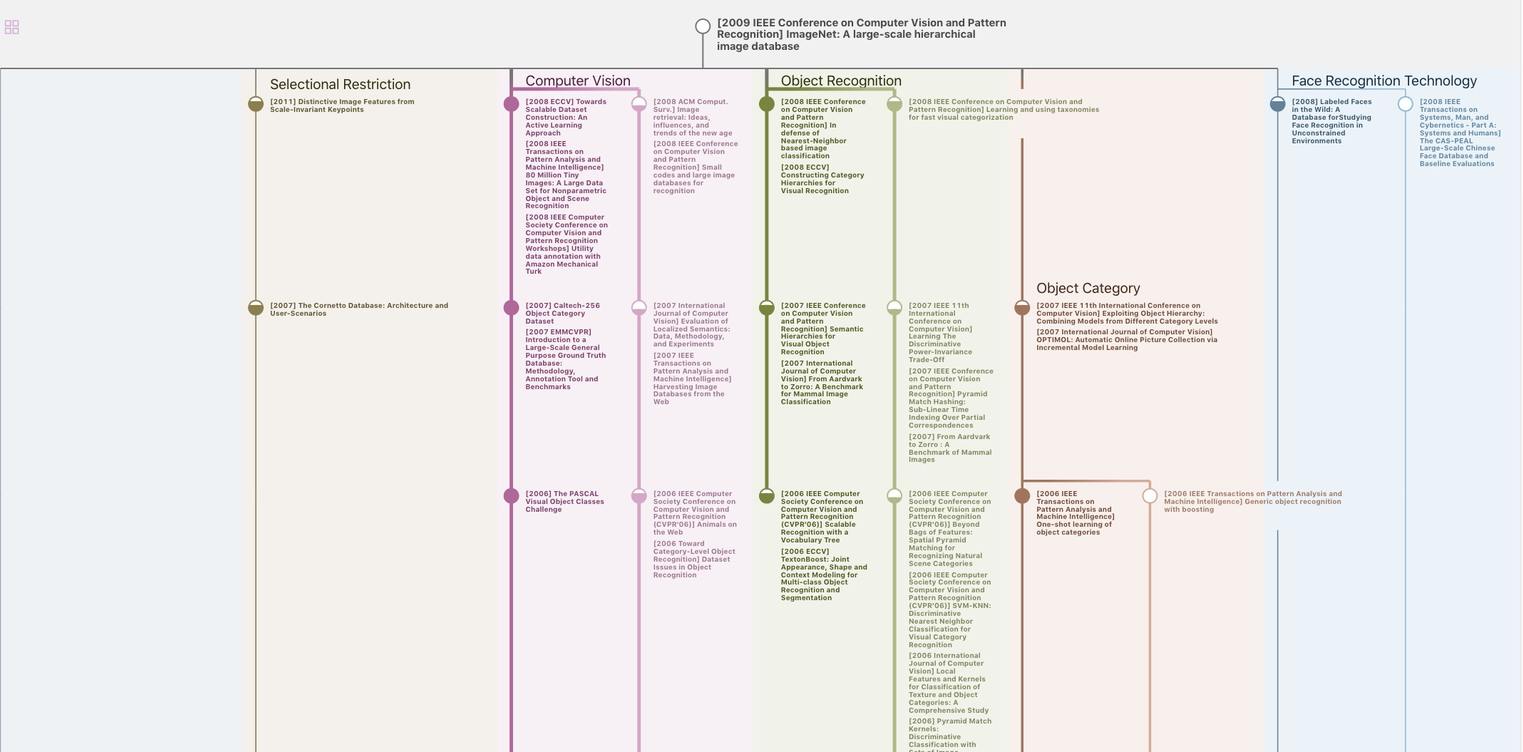
生成溯源树,研究论文发展脉络
Chat Paper
正在生成论文摘要