Object-Level Semantic Segmentation On The High-Resolution Gaofen-3 Fusar-Map Dataset
IEEE JOURNAL OF SELECTED TOPICS IN APPLIED EARTH OBSERVATIONS AND REMOTE SENSING(2021)
Abstract
Land cover classification with SAR images mainly focuses on the utilization of fully polarimetric SAR (PolSAR) images. The conventional task of PolSAR classification is single-pixel-based region-level classification using polarimetric target decomposition. In recent years, a large number of high-resolution SAR images have become available, most of which are single-polarization. This article explores the potential of object-level semantic segmentation of high-resolution single-pol SAR images, in particular tailored for the Gaofen-3 (GF-3) sensor. First, a well-annotated GF-3 segmentation dataset "FUSAR-Map" is presented for SAR semantic segmentation. It is based on four data sources: GF-3 single-pol SAR images, Google Earth optical remote sensing images, Google Earth digital maps, and building footprint vector data. It consists of 610 high-resolution GF-3 single-pol SAR images with the size of 1024 x 1024. Second, an encoder-decoder network based on transfer learning is employed to implement semantic segmentation of GF-3 SAR images. For the FUSAR-Map dataset, an optical image pretrained deep convolution neural network (DCNN) is fine-tuned with the SAR training dataset. Experiments on the FUSAR-Map dataset demonstrate the feasibility of object-level semantic segmentation with high-resolution GF-3 single-pol SAR images. Also, our algorithm obtains fourth place about the PolSAR image semantic segmentation on the "2020 Gaofen Challenge on Automated High-Resolution Earth Observation Image Interpretation." The new dataset and the encoder-decoder network are intended as the benchmark data and baseline algorithm for further development of semantic segmentation with high-resolution SAR images. The FUSAR-Map and our algorithm are available at github.com/fudanxu/FUSAR-Map/.
MoreTranslated text
Key words
Image segmentation, Radar polarimetry, Semantics, Synthetic aperture radar, Earth, Buildings, Image resolution, 2020 GaoFen challenge, encoder–, decoder network, FUSAR, map dataset, GaoFen-3 (GF-3) single -polarization SAR images, object-level semantic segmentation
AI Read Science
Must-Reading Tree
Example
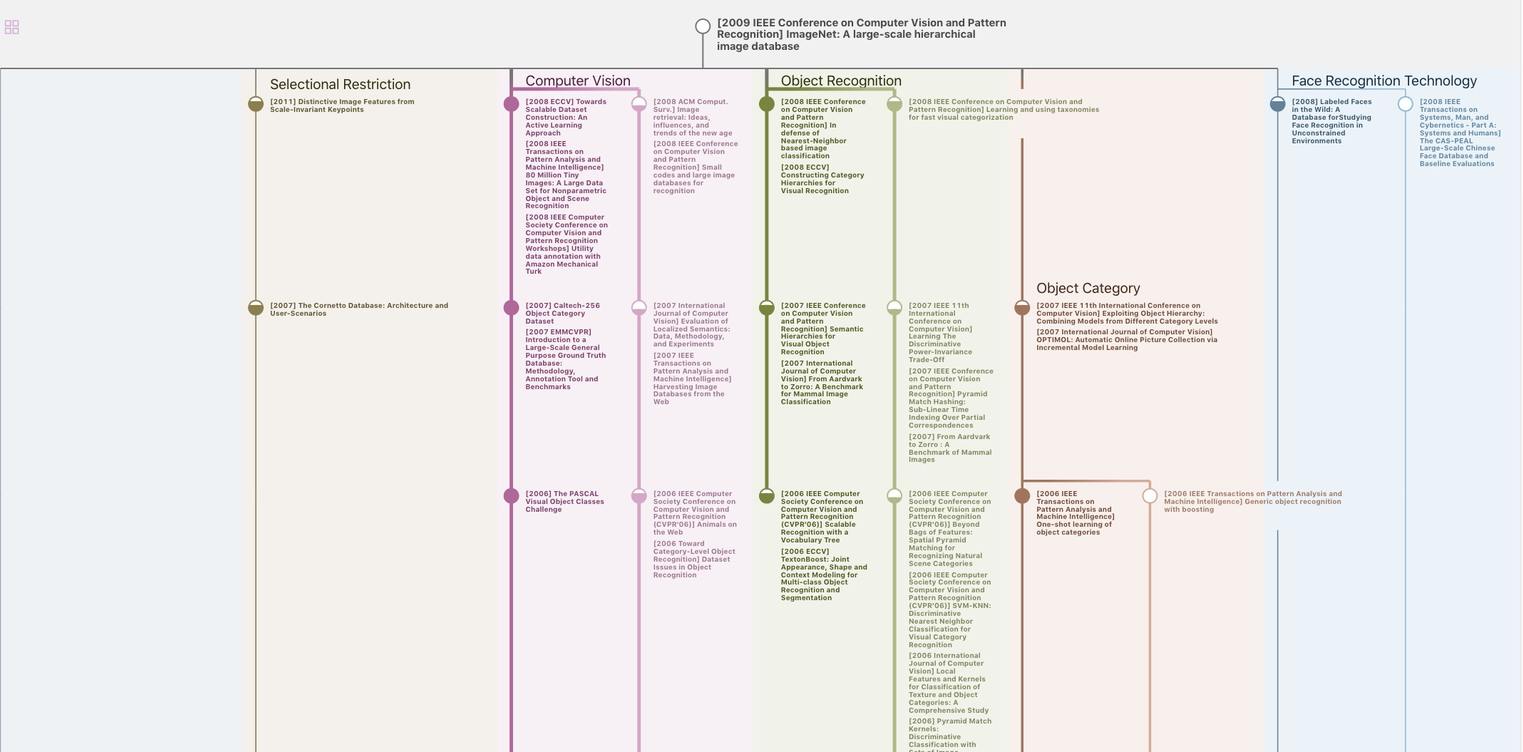
Generate MRT to find the research sequence of this paper
Chat Paper
Summary is being generated by the instructions you defined