Coupling Bayesian-Monte Carlo Simulations With Substance Flow Analysis For Efficient Pollutant Management: A Case Study Of Phosphorus Flows In China
RESOURCES CONSERVATION AND RECYCLING(2021)
摘要
Phosphorus is an essential nutrient for plants and also a natural component of healthy rivers. Yet, an overload of phosphorus could lead to the degradation of water quality in rivers and streams, thereby causing eutrophication of surface waters. Substance flow analysis (SFA) as a well-established analytical method has been widely used by scholars and scientists to quantify and manage the major nutrient flows entering water bodies over different spatial scales, e.g., the watershed or regional or national scale. However, despite its utility, this method has a notable limitation in that it cannot quantify the data uncertainty efficiently. This may significantly limit its application and lead to less reliable results. In this study, we propose a conceptual framework which enables integration of Bayesian networks, Monte Carlo simulations and substance flow analysis. The Bayesian-Monte Carlo simulation-based substance flow analysis (BMC-SFA) technique is able to account for random uncertainty, and thus can help to deliver an improved understanding of nutrient flows in surface waters. We use the BMC-SFA technique to carry out a systematic analysis of phosphorus flows in China. The results show that the livestock droppings on farmlands account for the most considerable uncertainty of phosphorus flows in China. Suggestions for uncertainty reduction and risk management are given to enable efficient phosphorus flow management in the future. Overall, the BMC-SFA technique can be a useful tool for guiding pollution control and water quality improvement efforts in surface waters.
更多查看译文
关键词
Substance flow analysis, Phosphorus, Bayesian networks, Monte Carlo simulations, Uncertainty
AI 理解论文
溯源树
样例
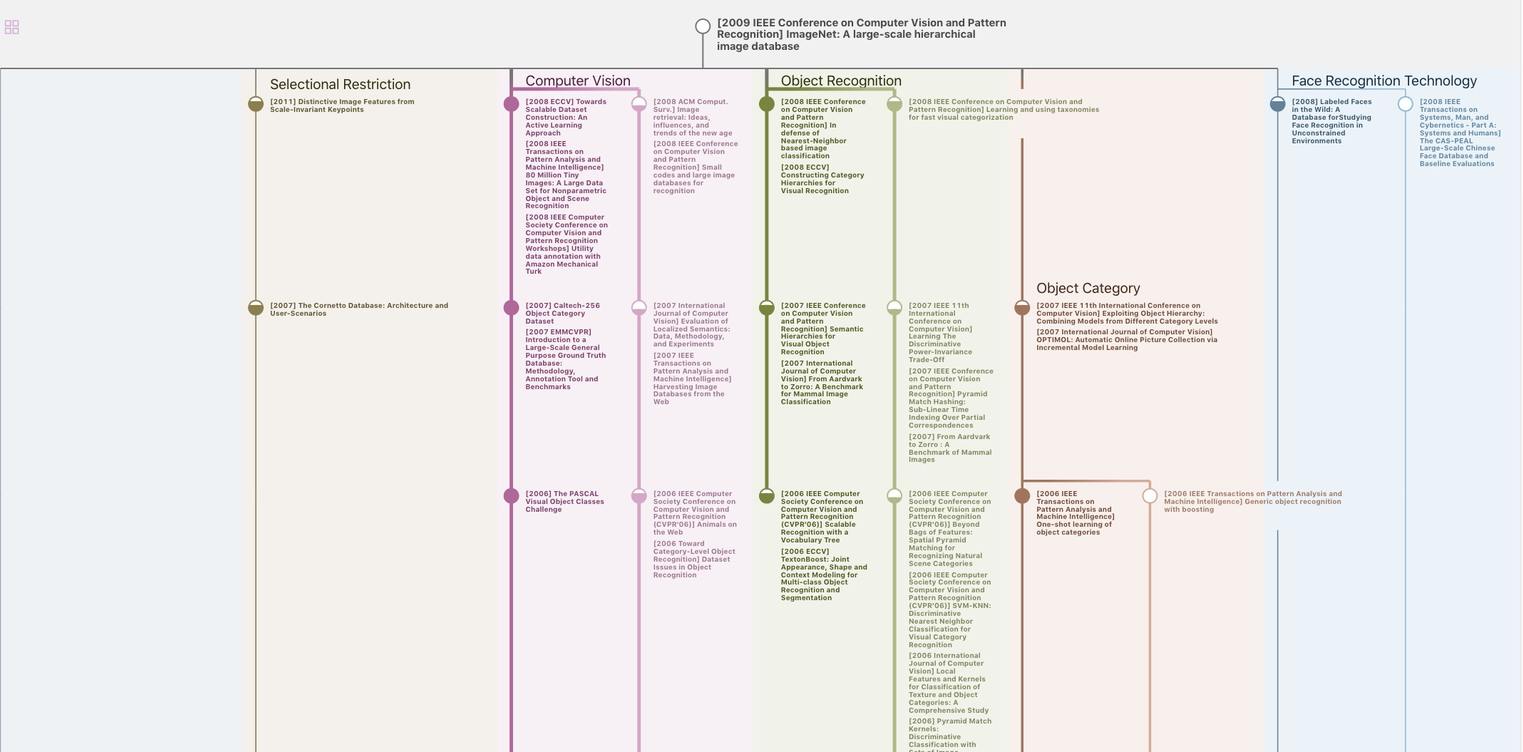
生成溯源树,研究论文发展脉络
Chat Paper
正在生成论文摘要