Broad learning system for semi-supervised learning
Neurocomputing(2021)
摘要
As an emerging technique for supervised learning, broad learning system (BLS) has been proved to have many advantages such as fast learning speed, good generalization, etc. However, it is difficult for BLS to perform well on the dataset which only contains few labeled samples. Because the focus of semi supervised learning is utilizing the information between labeled and unlabeled samples while the original BLS apparently ignores this significant information. In BLS, the mapped features are obtained by sparse autoencoder which is an unsupervised learning method and the enhancement nodes are formed based on the mapped features. But sparse autoencoder will definitely lose the information of labeled instances. Therefore, we propose a novel semi-supervised BLS (S2-BLS) in this paper. In S2-BLS, inspired by the effectiveness of extreme learning machine based autoencoder (ELM-AE), we first propose semi supervised ELM-AE (SS-ELM-AE) to obtain the mapped features. Then, the discriminative projecting weights between the ground truths and the transformed features (including the mapping features and the enhancement nodes) are calculated directly. Finally, we adopt some public datasets to verify the learning ability of S2-BLS. And the experimental results illustrate that S2-BLS has an obvious advantage to learn labeled and unlabeled data, comparing with related algorithms. (c) 2021 Elsevier B.V. All rights reserved.
更多查看译文
关键词
Semi-supervised learning,Broad learning system,Extreme learning machine,Graph
AI 理解论文
溯源树
样例
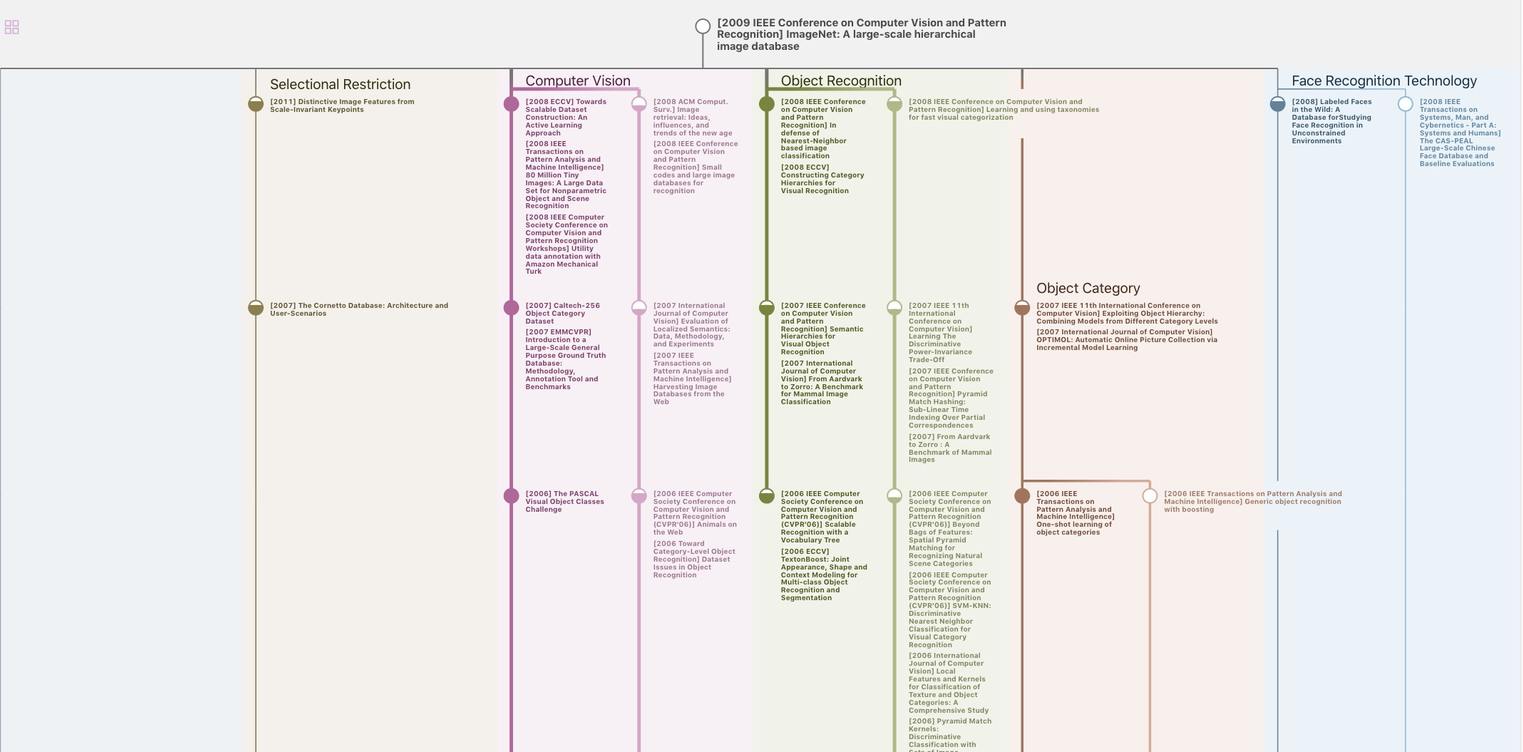
生成溯源树,研究论文发展脉络
Chat Paper
正在生成论文摘要