Temporal Pyramid Network With Spatial-Temporal Attention for Pedestrian Trajectory Prediction
IEEE Transactions on Network Science and Engineering(2022)
摘要
Understanding and predicting human motion behavior with social interactions have become an increasingly crucial problem for a vast number of applications, ranging from visual navigation of autonomous vehicles to activity prediction of intelligent video surveillance. Accurately forecasting crowd motion behavior is challenging due to the multimodal nature of trajectories and complex social interactions between humans. Recent algorithms model and predict the trajectory with a single resolution, making them difficult to exploit the long-range information and the short-range information of the motion behavior simultaneously. In this paper, we propose a temporal pyramid network for pedestrian trajectory prediction through a squeeze modulation and a dilation modulation. The hierarchical design of our framework allows to model the trajectory with multi-resolution, then can better capture the motion behavior at various tempos. By progressively combining the global context with the local one, we finally construct a coarse-to-fine hierarchical pedestrian trajectory prediction framework with multi-supervision. Further, we introduce a unified spatial-temporal attention mechanism to adaptively select important information of persons around in both spatial and temporal domains. We show that our attention strategy is intuitive and effective to encode the influence of social interactions. Experimental results on two benchmarks demonstrate the superiority of our proposed scheme.
更多查看译文
关键词
Deep learning,social behavior,social computing,social interactions,spatial-temporal attention,temporal pyramid network,trajectory prediction
AI 理解论文
溯源树
样例
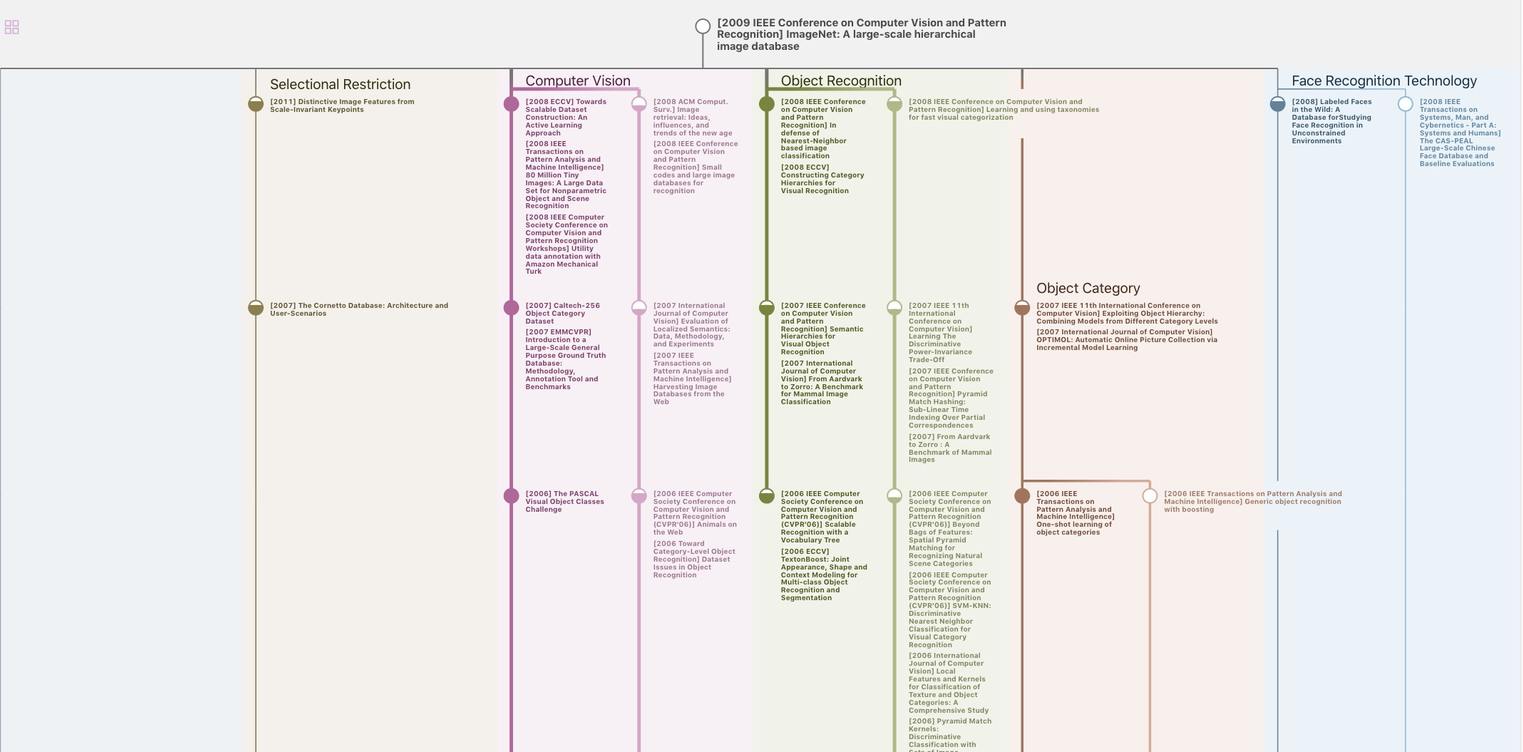
生成溯源树,研究论文发展脉络
Chat Paper
正在生成论文摘要